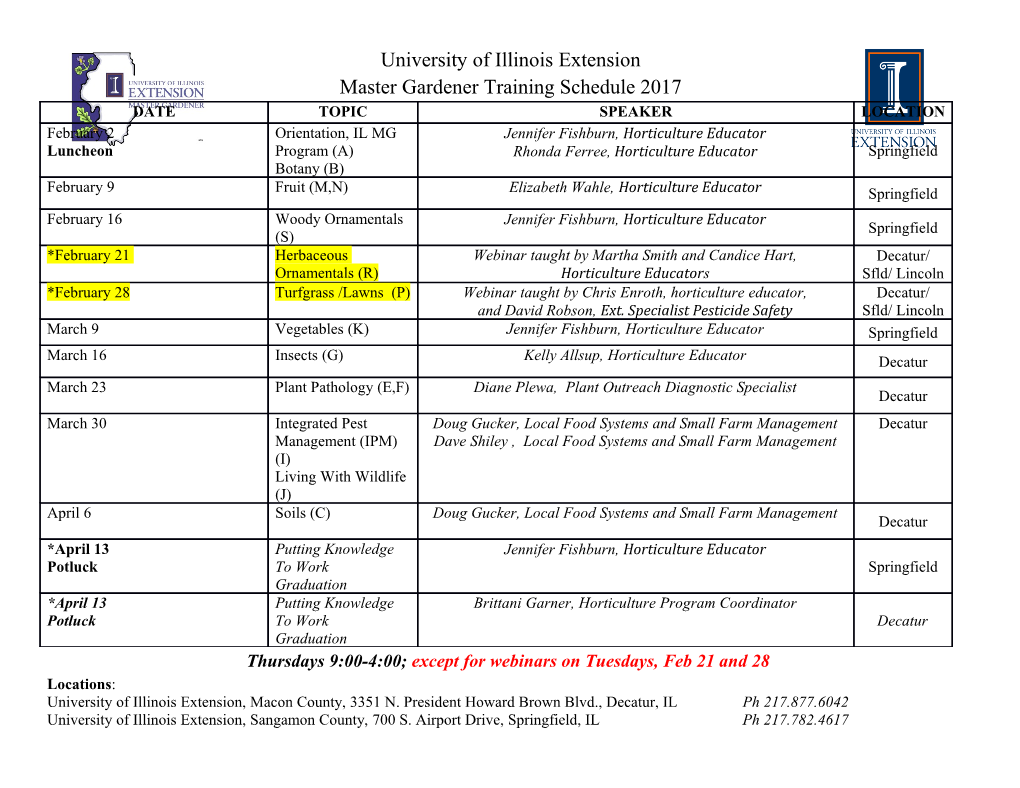
Transient Effects of Linear Dynamical Systems von Elmar Plischke Dissertation zur Erlangung des Grades eines Doktors der Naturwissenschaften – Dr. rer. nat. – Vorgelegt im Fachbereich 3 (Mathematik & Informatik) der Universit¨at Bremen im Juli 2005 Datum des Promotionskolloquiums: 19. August 2005 Gutachter: Prof. Dr. Diederich Hinrichsen (Universit¨atBremen) PD Dr. Fabian Wirth (NUI Maynooth, Irland) Contents Introduction 1 1 Preliminaries 7 1.1 Matrix Analysis . 7 1.2 Properties of Norms . 8 1.3 Spectral Value Sets and Stability Radii . 10 1.4 Linear Operators . 11 2 Contractions and Liapunov Norms 15 2.1 One-Parameter Semigroups in Banach Spaces . 15 2.2 Asymptotic Growth Rates . 22 2.3 Initial Growth Rates . 25 2.4 Liapunov Norms . 38 2.5 Notes and References . 48 3 Bounds for the Transient Amplification 51 3.1 (M, β)-Stability . 51 3.2 Bounds from the Spectrum . 55 3.3 Bounds from Singular Value Decompositions . 60 3.4 Bounds via Liapunov Functions . 70 3.5 Bounds from the Resolvent . 77 3.6 Notes and References . 89 4 Examples 91 4.1 Explicit Formulas . 91 4.2 Construction of Transient Norms . 93 4.3 Liapunov Matrices of Minimal Condition Number . 99 4.4 Dissipativity for Polytopic Norms . 104 4.5 Notes and References . 105 5 Positive Systems Techniques 107 5.1 Properties of Metzler Matrices . 107 5.2 Transient Bounds for Metzler Matrices . 109 iii iv CONTENTS 5.3 Optimal Liapunov Vectors . 113 5.4 Common Liapunov Vectors . 117 5.5 The Metzler Part of a Matrix . 121 5.6 Transient Bounds for General Matrices . 123 5.7 Notes and References . 128 6 Differential Delay Systems 131 6.1 Functional Analytic Approach . 132 6.2 Liapunov Functionals . 137 6.3 Existence and Uniqueness of Delay Liapunov Matrices . 147 6.4 The One-Delay Case . 156 6.5 Uncertain Delays . 165 6.6 Multiple Delays . 172 6.7 Scalar Differential Delay Equations . 179 6.8 Notes and References . 181 7 (M, β)-Stabilization 183 7.1 Synthesis of Contractions . 183 7.2 Contractibility for Polytopic Norms . 188 7.3 (M, β)-Stabilizability . 190 7.4 Quadratic (M, β)-Stabilizability . 191 7.5 Quadratic Programs for (M, β)-Stabilization . 196 7.6 Notes and References . 198 List of Symbols 201 Bibliography 203 Index 212 Introduction From a practical point of view the concept of stability may be deceiving: Asymptotic stability allows for arbitrary growth before a decay occurs. These transient effects which are only of temporary nature have no influence on the asymptotic stability of a dynamical system. However, these effects might dominate the system’s performance. Hence we are in need of information which describes the short-time behaviour of a dynamical system. Motivation Everyone has noticed that devices need some time to get ready for use, like an old radio warming up or a computer booting. On a larger scale, plants also need an amount of time to reach their working point. But in this initial phase, the plant is particularly vulnerable. The faster one wants to reach the working point, the more stress the plant has to endure: there may be overshots which carry some parts of the plant to the limit of their capacity. One may think of chemicals, which advance towards toxic or explosive concentrations, before reaching the desired concentration of the reagents, or an autopilot steering the wrong way before getting on track. One could believe that such a distinctive behaviour in the initial phase can only occur for complex dynamical systems. However, this behaviour can already be observed for linear differential equations which provide simple models for dynamical processes. To avoid catastrophes like those indicated above, one wishes to eliminate the bad influences in the starting phase, or at least, to keep them small. Furthermore, methods are needed that allow to predict if the system under consideration shows these transient effects, and if so, to obtain information on the duration and intensity of these excursions. This work is mainly concerned with questions dealing with the last two issues, namely finding bounds on the exponential growth of linear systems. Although there are many results on exponential bounds, there is still no systematic treatment in the literature. The mathematical model of a plant will in general not yield the accurate description of the behaviour of the real plant. Hence we are in need of results which are robust under small perturbations of the mathematical model. Fortunately, these results follow directly from our systematic treatment of the exponential bounds. In addition to the general theory, we study two major classes of linear systems, namely positive systems and delay-differential systems, which are used frequently in economics and biology. 1 2 INTRODUCTION Moreover, we study the influence of state feedback on the transient behaviour. We ob- tain necessary and sufficient conditions to obtain a closed-loop system without transient excursions. Finite-dimensional linear systems are mostly used as an approximation of more complex dynamical systems. These are obtained by linearization or discretization. Let us now discuss two possible ways in which the transients of linear systems may influence the dynamics. From Transience to Turbulence Most linear dynamical systems are obtained by linearizing a nonlinear model of a real process around an equilibrium point. Now, Liapunov’s theorem implies that the nonlinear system is asymptotically stable if the linearization is asymptotically stable. Figure 1: Toy model for turbulence. But if the asymptotically stable linear system has solutions which move far afield before eventually decaying, these solutions of the linear system may leave the domain for which the linear system is a valid approximation of the nonlinear system. Hence small perturba- tions from the equilibrium point may incite nonlinearities. Models of this kind have been suggested in Baggett and Trefethen [8] to explain why turbulence of certain flows occurs at Reynolds numbers much smaller than predicted from a spectral analysis. For example, let us investigate the following nonlinear time-invariant ordinary differential equation −5 36 0 −1 x˙ = Ax + B(x) = x + kxk x, x ∈ 2, (1) 0 −20 1 0 R where A is an asymptotically stable, but nonnormal matrix and the nonlinearity B(x) is conservative (energy-preserving), thus B only adds a rotation of the state-space to the INTRODUCTION 3 linear systemx ˙ = Ax. Figure 1 shows many trajectories of system (1) starting on a circle of radius 50. The trajectories which converge to the origin are colored in black, which gives a rough approximation of the domain of attraction for the origin. One observes that this domain of attraction is flat, the nonnormality of the linear systemx ˙ = Ax quickly drives the state into regions where the nonlinearity has strong effects on the state. Note that in this example nonlinearity and nonnormality form opposite forces which create a sort of conveyor belt driving the states away from the stable origin. The picture drastically changes when replacing B(x) by −B(x). From Transience to Permanence Another interesting observation can be made when approximating infinite dimensional systems by finite dimensional approximants. Now assume that there exists a sequence of finite dimensional matrices which approximate an infinite dimensional linear operator, and that this sequence consists only of stable matrices. Then the infinite dimensional system need not be stable. But this can be detected by studying the transient behaviour of the approximants. Let us consider the matrix exponential of Jordan-blocks Jn of growing size n 1 associated with the eigenvalue λ0 = − /2. These blocks approximate a “multiply-and-shift” 2 operation J∞ on the sequence space ` (C) given by 2 2 1 J∞ : ` (C) → ` (C), (xk) 7→ (xk+1 − 2 xk). But the spectrum of J∞ consists of a whole unit ball centered around λ0, because it is a 1 Toeplitz operator with symbol s 7→ − 2 + s, see B¨ottcher and Silbermann [21], and 1 ∂σ(J∞) = − 2 + S, where S = {s ∈ C | |s| = 1}. Hence the asymptotic growth rate of the semigroup generated by J∞ is given by α(J∞) = 1 sup{Re λ | λ ∈ σ(J∞)} = /2. Figure 2 shows the growth of kexp(Jnt)k for n = 2, 4, 8, 16, 32. 1 Although all Jn, n ∈ N, are stable, the limit /2 of the transient growth rates given by d Jnt µn = dt+ e t=0 coincides with the asymptotic growth rate of J∞. The Curse of Nonnormality Both of these examples suffer from the same defect: the linear matrix A is highly nonnor- mal, i.e., there exists no orthogonal basis of eigenvectors. Moreover, if there are eigenvectors which roughly point into the same direction then there are vectors of small size for which the coordinates will blow up if they are represented in an eigenvector basis, see Figure 3 7 13 where w = /2v1 − /4v2. Clearly, if the angle spanned by v1 and v2 becomes more acute, this results in larger coordinates of w in the {v1, v2}-basis. Grossmann [49, 50] calls this behaviour the “blind spot” of such a basis. Henrici [54] has identified the nonnormality as a cause for the failure of many numerical algorithms. He introduced the departure from normality as a measure of nonnormality. 4 INTRODUCTION Figure 2: Exponential growth of Jordan blocks. However, there are matrices for which the departure from normality is small, but transient effects are present, e.g. dep(Jn) = 1 for a Jordan block of dimension n. Outline of the Thesis The next chapter is devoted to some mathematical preliminaries which fix the notations used throughout this thesis and cover some topics from linear algebra and functional anal- ysis that are used in the later chapters. In Chapter 2 we collect some facts for generators of strongly continuous semigroups and semigroups of contractions.
Details
-
File Typepdf
-
Upload Time-
-
Content LanguagesEnglish
-
Upload UserAnonymous/Not logged-in
-
File Pages218 Page
-
File Size-