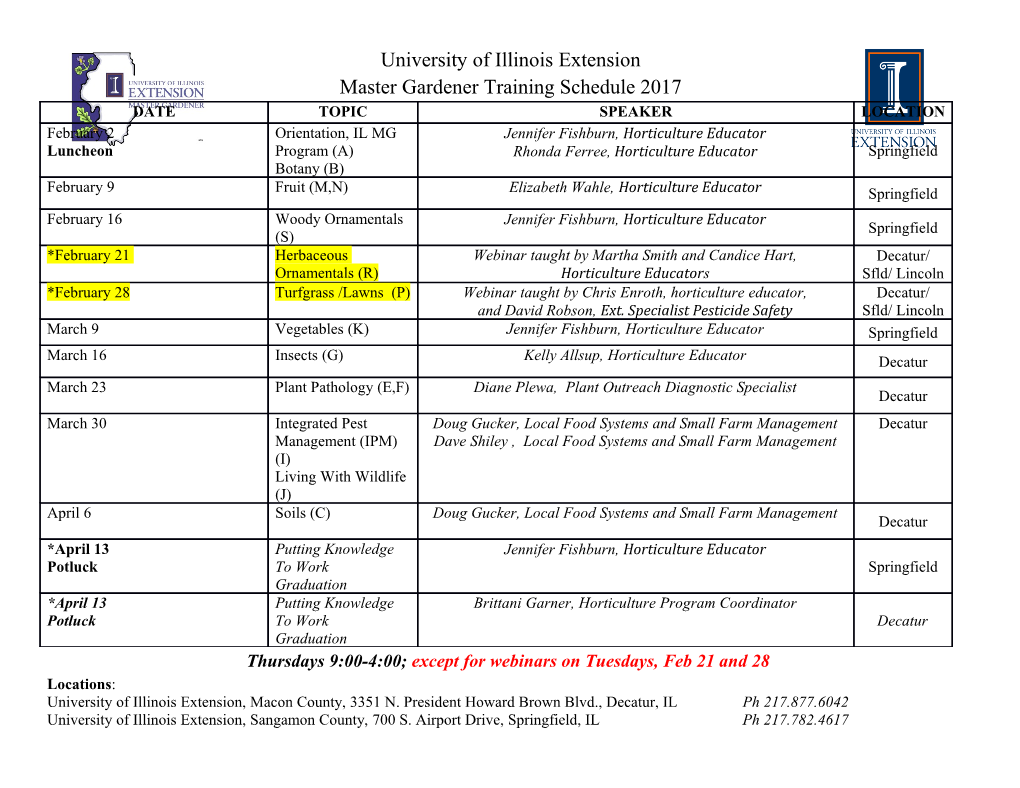
HST 190: Introduction to Biostatistics Lecture 3: Two-sample comparisons, hypothesis testing and power/sample size calculations 1 HST 190: Intro to Biostatistics Estimating two-sample comparisons • In Lecture 2, we introduced: § probability distributions § sampling from a population § estimation of the mean of some random variable § quantification of uncertainty via standard errors and confidence intervals • Today, we focus on a common setting in public health and medical research, specifically the comparison of two groups • Consider, for example, the question: “What is the difference in average birthweight between children born at a specific hospital in a disadvantaged area and children born at a specific hospital in a wealthy area?” 2 HST 190: Intro to Biostatistics • This question moves beyond the estimation scenario we discussed in Lecture 2 § that is, interest lies in more than a single mean � • Now, we want to compare two unknown means § each corresponding to some (sub-)population • How we achieve this, specifically estimation and the quantification of uncertainty, depends on how the data we have at our disposal was collected. 3 HST 190: Intro to Biostatistics Paired samples • Solish et al (2010, Clinical Ophthalmology) reported on a study of medical therapy for uncontrolled glaucoma § � = 55 patients § bimatoprost 0.03% once daily in one eye § either travoprost 0.004% or latanoprost 0.005% once daily in the fellow eye § measured compared intraocular pressure (IOP) at baseline and at 4- 6 weeks follow-up • This is an example of a paired study § � is number of pairs not number of total observations 4 HST 190: Intro to Biostatistics • Let � denote the change in IOP between baseline and follow-up • Measurements in each of the two eyes: § �$$, �&$, … , �)$ § �$&, �&&, … , �)& • Solish et al report estimated mean reductions in IOP of: § �$̅ = 2.7 mmHg among bimatoprost-treated eyes § �̅&= 1.7 mmHg among travoprost-treated eyes • Suggests that treatment with bimatoprost is associated with greater improvements • Q: How can we use the methods we’ve been exposed to so far to formally assess this comparison? 5 HST 190: Intro to Biostatistics • Define a new random variable � to be the difference in the IOP change between the two eyes: § �- = �-& – �-$ • Thus, we have exploited the special nature of the paired data to turn the two-sample problem into a one-sample § E D = Δ & § ��� � = �7 • We can now proceed just as in Lecture 2: $ § use �̅ = ∑) � sample mean of the differences to estimate Δ ) -:$ - ? $ & § use � = ∑) � − �̅ to estimate the standard deviation < )=$ -:$ - ̅ ̅ ? o note, this means that the standard error of � is sd � = �</ � <C=D § use ? ~�)=$ to derive 100(1 − �)% confidence interval for Δ EF/ ) � ̅ < � ± � P ⋅ ? )=$,$=& � 6 HST 190: Intro to Biostatistics Unpaired samples • In many settings there may not be an obvious path for creating “pairs” on which to base the two-sample comparison • As an example, Maciejewski et al (2016, JAMA) report on weight loss following bariatric surgery: § 1787 veterans who underwent RYGB § 5305 non-surgical patients from the same VA populationResearch Original Investigation Bariatric Surgery and Long-term Weight Loss Durability Statistical Analysis Figure 1. Differences in Estimated Weight Changes Among Patients Undergoing Roux-en-Y Gastric Bypass (RYGB) and Nonsurgical Matches The statistical analysis was developed to estimate mean tra- jectories of the percentage of weight change and estimates at Patients undergoing RYGB specific postsurgical years. Penalized spline mixed-effects 10 Nonsurgical matches models were used, with piecewise linear functions with pre- specified knots included as fixed and random effects at the 0 population-average and subject-specific levels.31,32 This ap- proach allowed us to estimate overall mean trajectories and –10 individual trajectories with enough flexibility to reflect weight 30 27 24 22 21 (29-30) (25-29) (20-28) (16-28) (11-31) change fluctuations during an individual’s entire follow-up –20 (eFigure 3 in the Supplement illustrates 75 cases). Because of the extent of weight change in the 2 years after –30 surgery,our primary model specification for the overall mean in- Weight Change From Baseline, % Change From Weight cluded a linear term for time and knots at 3 months, 6 months, –40 and 1, 2, 3, 5, 7,and9 years. All time terms were interacted with 0 1 2 3 4 5 6 7 8 9 10 11 atreatmentindicatortoallowdifferentialestimatesforpatients Years Since Baseline undergoing RYGB and nonsurgical matches using HPMIXED in SAS statistical software, version 9.4(SAS Institute Inc). The model 7 Estimated values, differences, and 95% CIs (shown in parentheses) were HST 190: Intro to Biostatistics generated from a penalized spline mixed-effects model (7092 patients: 5305 also included individual-level random effects with knots at 6 nonsurgical matches and 1787 patients undergoing RYGB). Numbers and arrows months and 1, 2, 3, 5, 7,and9 years;asubject-levelrandomslope in the center of the figure represent the differences and 95% CIs of the to account for correlation among patients’ repeated measures; differences between nonsurgical matches and patients undergoing RYGB at and a VA geographic area–level random slope to account for cor- years 1, 3, 5, 7, and 10. The sample for whom there was follow-up weight data for each year are as follows: year 1, n = 6894 patients (5131 nonsurgical matches relation between each pair matched within VA geographic area and 1763 patients undergoing RYGB); year 3, n = 6301 (4629 nonsurgical and patients matching more than once. Estimate statements were matches and 1672 patients undergoing RYGB); year 5, n = 5172 (3748 used to generate the predicted percentage of weight change at nonsurgical matches and 1424 patients undergoing RYGB); year 7, n = 3942 (2806 nonsurgical matches and 1136 patients undergoing RYGB); and year 10, 1, 3, 5, 7 and 10 years of follow-up for the patients undergoing n = 1847 (1274 nonsurgical matches and 573 patients undergoing RYGB). RYGBcomparedwiththenonsurgicalmatches.Themodel’sem- pirical Bayes estimates were used to calculate patients’ predicted weights at 1,3,5,7,and10 yearsonlyforthosewithaweightmea- surement within a 12-month interval of (6 months before to 6 (n = 61), who only had inpatient weights after surgery (n = 1), months after) and at least 1 measurement after each follow-up who lacked postsurgical weights (n = 6), or who did not have year,whichwereusedtoclassifyeachpatientashavinglostless a weight measurement within 5 years after surgery (n = 2 pa- than 5%, 5%ormore,10%ormore,20%ormore,and30%or tients undergoing RYGB). This resulted in a final sample of 1785 more of their baseline weight. The 12-month interval requirement patients undergoing RYGB, 246 undergoing AGB, and 379 un- modestly reduced the number of patients for whom individual dergoing SG (eFigure 2 in the Supplement). weights could be predicted. For example, 573 surgical patients were followed up for at least 10 years, but only 564 of these 573 Definition and Cleaning of Weight Outcome had a weight measurement between 9.5and 10.5years. Patients’ Follow-up weight data were obtained from measurements re- predicted weights at 10 years of follow-up were used to calcu- corded in the electronic health records during outpatient vis- late mean weight loss and excess body weight loss from baseline. its from January 1, 2000,throughDecember31, 2014.Thepri- Asimilarmodelwithknotsspecifiedat3 months, 6 months, mary outcome was percentage change in weight at follow-up and 1,2,and3yearswasusedtocompareweightchangethrough compared with baseline, which is less confounded by base- 4yearsoffollow-upacrosssurgicalprocedures,whichhadshorter line BMI than other commonly reported measures (eg, per- follow-up because of the recent introduction of the SG procedure centage of excess weight loss).30 Baseline weight data on the and study end date of December 31,2014 (eFigure 2in the Supple- surgery day was available for 78.6%ofpatientsundergoing ment). To understand the effect of baseline differences in patients RYGB but only 2.2%ofnonsurgicalmatches,andthemean(SD) who underwent each surgical procedure, patients’ predicted per- number of days for the baseline weight measurement before centage of weight loss at 4 years was regressed on surgery type surgery was 7.3 (24.0) for patients undergoing RYGB and 59.8 while adjusting for baseline BMI, comorbidity score, demograph- (48.6) for nonsurgical matches. ics, and diabetes status (eTable in the Supplement). Postsurgical weight measurements are highly variable and have a nonlinear trend, so we developed a multistep outlier- detection algorithm that examined the SD of consecutively Results measured weights (eAppendix in the Supplement). An algo- rithm to identify multiple weight measures on the same day, Characteristics of Patients Undergoing RYGB or weights that deviated from clinically plausible trends over and Nonsurgical Matches time, excluded a small proportion (4201 of 89 757 [4.7%]) of Patients undergoing RYGB had a mean (SD) age of 52.1 (8.5) weight measurements. yearsandnonsurgicalmatcheshadamean(SD)ageof 52.2 (8.4) E4 JAMA Surgery Published online August 31, 2016 (Reprinted) jamasurgery.com Copyright 2016 American Medical Association. All rights reserved. Downloaded From: http://archsurg.jamanetwork.com/ by a Department of Veterans Affairs User on 08/31/2016 • More generally, consider two independent (unpaired) samples of size �$ and �& • Suppose: & § �$$, �&$, … , �)R$ ~ ������ �$, �$ & § �$&, �&&, … ,
Details
-
File Typepdf
-
Upload Time-
-
Content LanguagesEnglish
-
Upload UserAnonymous/Not logged-in
-
File Pages71 Page
-
File Size-