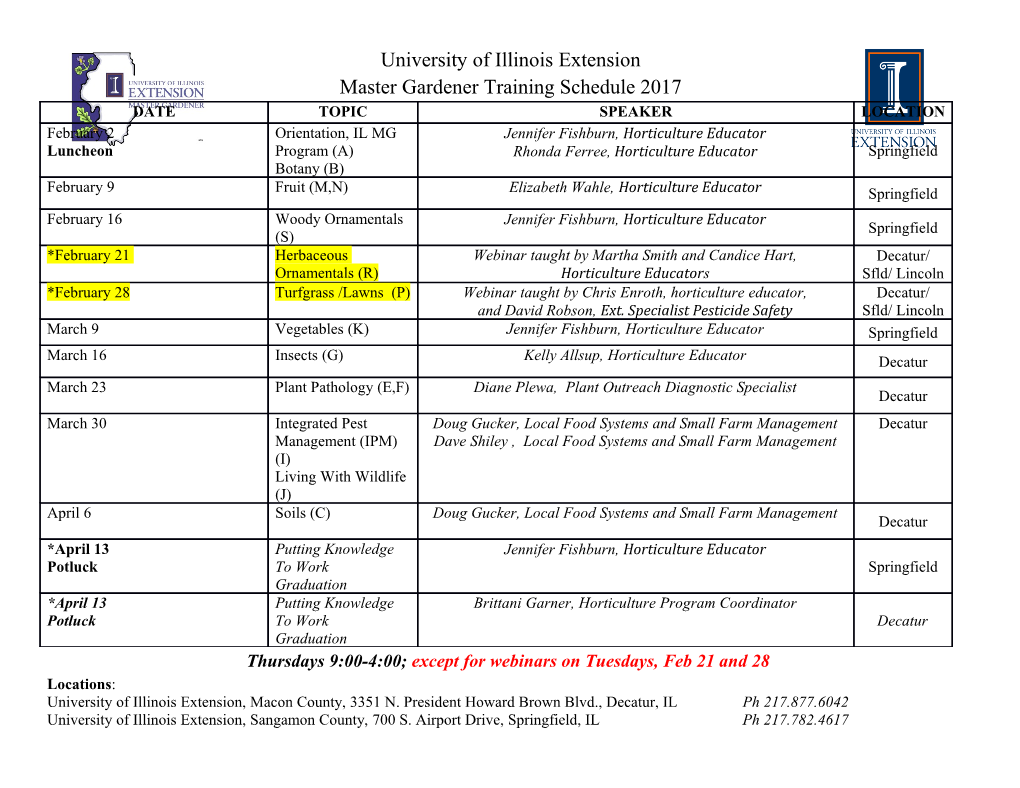
bioRxiv preprint doi: https://doi.org/10.1101/2020.07.14.201475; this version posted July 14, 2020. The copyright holder for this preprint (which was not certified by peer review) is the author/funder, who has granted bioRxiv a license to display the preprint in perpetuity. It is made available under aCC-BY 4.0 International license. Tareen et al. RESEARCH MAVE-NN: Quantitative Modeling of Genotype- Phenotype Maps as Information Bottlenecks Ammar Tareen1, William T. Ireland2, Anna Posfai1, David M. McCandlish1 and Justin B. Kinney1* are commonly used to study DNA or RNA sequences that regulate gene expression at a variety of steps, in- cluding transcription [16, 17, 18, 19, 20, 21], splicing [22, 23, 24, 25, 26], polyadenylation [27], and mRNA Abstract degradation [28, 29, 30, 31, 32]. Most MPRAs read Multiplex assays of variant effect (MAVEs) are out the expression of a reporter gene in one of two being rapidly adopted in many areas of biology ways [1]: by quantifying RNA abundance via the se- including gene regulation, protein science, and quencing of RNA barcodes that are linked to known evolution. However, inferring quantitative models variants (RNA-seq MPRAs; Fig. 1c), or by quanti- of genotype-phenotype maps from MAVE data fying protein abundance using fluorescence-activated remains a challenge. Here we introduce MAVE-NN, cell sorting (FACS) then sequencing the sorted vari- a neural-network-based Python package that ants (sort-seq MPRAs; Fig. 1e). addresses this problem by conceptualizing MAVE data can enable rich quantitative modeling of genotype-phenotype maps as information G-P maps. This key point was recognized in some of bottlenecks. We demonstrate the versatility, the earliest work on MAVEs [17, 18] and has persisted performance, and speed of MAVE-NN on a diverse as a major theme in MAVE studies [33, 34, 35, 25, 31, range of published MAVE datasets. MAVE-NN is 36]. But in contrast to MAVE experimental techniques, easy to install and is thoroughly documented at which continue to advance rapidly, there remain key https://mavenn.readthedocs.io. gaps in the methodologies available for quantitatively Keywords: MAVE; Neural Networks; Noise modeling G-P maps from MAVE data. Agnostic Regression; Global Epistasis Regression Most computational methods for analyzing MAVE data have focused on accurately quantifying the ac- tivities of individual assayed sequences [37, 38, 39, 40, 41, 42, 43]. However, MAVE measurements for individ- Background ual sequences often cannot be interpreted as providing Over the last decade, the ability to quantitatively direct quantification of the underlying G-P map that study genotype-phenotype (G-P) maps has been rev- one is interested in. First, MAVE measurements are olutionized by the development of multiplex assays of usually distorted by strong nonlinearities and noise, variant effect (MAVEs), which can measure molecu- and distinguishing interesting properties of G-P maps lar phenotypes for thousands to millions of genotypic from these confounding factors is not straight-forward. variants in parallel [1]. MAVE is an umbrella term Second, MAVE data is often incomplete. Missing data that describes a diverse set of experimental methods is common, but a more fundamental issue is that re- [2,3], three examples of which are illustrated in Fig. searchers often want to understand G-P maps over 1. Deep mutational scanning (DMS) experiments are vastly larger regions of sequence space than can be one large class of MAVE [4]. These work by linking exhaustively assayed. proteins [5,6, 7] or structural RNAs [8, 9, 10, 11] to their coding sequences, either directly or indirectly, Quantitative modeling can address both the incom- then using deep sequencing to assay which variants pleteness and indirectness of MAVE measurements [1]. survive a process of activity-dependent selection (Fig. The goal here is to determine a mathematical func- 1a). Massively parallel reporter assays (MPRAs) are tion that, given any sequence as input, will return a another major class of MAVE [12, 13, 14, 15], and quantitative value for that sequence's molecular phe- notype. Quantitative models thus fill in the gaps in *Correspondence: [email protected] G-P maps and, if appropriate inference methods are 1Simons Center for Quantitative Biology, Cold Spring Harbor Laboratory, 11375, Cold Spring Harbor, NY used, can further remove confounding effects of nonlin- Full list of author information is available at the end of the article earities and noise. The simplest quantitative modeling bioRxiv preprint doi: https://doi.org/10.1101/2020.07.14.201475; this version posted July 14, 2020. The copyright holder for this preprint (which was not certified by peer review) is the author/funder, who has granted bioRxiv a license to display the preprint in perpetuity. It is made available under aCC-BY 4.0 International license. Tareen et al. Page 2 of 15 strategy is linear regression (e.g. [18, 44]). However, into a type of information bottleneck [63, 64], one that linear regression yields valid results only when one's separates the task of compressing sequence-encoded in- measurements are linear readouts of phenotype and formation (the job of the G-P map) from the task of exhibit uniform Gaussian noise. Such assumptions are mapping this information to a realistic experimental often violated in dramatic fashion by MAVEs, and fail- output (the job of the measurement process). Some ure to account for them can give rise to major artifacts, ambiguity between the quantitative properties of the such as spurious epistatic interactions [45]. G-P map and those of the measurement process in- Multiple MAVE analysis approaches that can sepa- evitably remain, but such ambiguities often affect only rate the effects of nonlinearities and noise from under- a small number of parameters in the underlying G-P lying G-P maps have been reported, but a conceptually map [54, 55]. unified strategy is still needed. Work in the theoreti- We also introduce MAVE-NN, a software package cal evolution literature has focused on a phenomenon that can rapidly execute this type of bottleneck-based called global epistasis (GE), in which measurements re- inference on large MAVE datasets. MAVE-NN cur- flect a nonlinear function of an underlying latent phe- rently supports two inference approaches: GE regres- notype [46, 47, 48, 49, 50, 51, 52, 53, 45]. In particular, sion and noise agnostic (NA) regression. GE regres- Otwinowski et al. [46] describe a regression approach in sion is modeled after the approach of [46]. NA re- which one parametrically models G-P maps while non- gression is closely related to non-parametric regres- parametrically modeling nonlinearities in the MAVE sion using information maximization (see [54, 55]) but measurement process. Parallel work in the biophysics is much faster due to its compatibility with back- literature has focused on developing ways to infer G-P propagation. MAVE-NN is available as a Python API maps from high-throughput data in a manner that is and is built on top of TensorFlow, allowing the use agnostic to the quantitative form of both nonlinearities of the powerful and flexible optimization methods. and noise [54, 55, 56, 17]. This approach focuses on the MAVE-NN is rigorously tested and is easily installed use of mutual information as an objective function. It arose from techniques in sensory neuroscience [57, 58] from PyPI using the command pip install mavenn. that were elaborated and adapted for the analysis Comprehensive documentation is available at http: of microarray data [59, 56], then applied to MPRAs //mavenn.readthedocs.io. [33, 34, 17, 18]. However, due to difficulties in esti- mating mutual information, such analyses of MAVE Results data have relied on Metropolis Monte Carlo, which Inference approaches (in our experience) is too slow to support widespread MAVE-NN supports the analysis of DNA, RNA, and adoption. A third thread in the literature has arisen protein sequences. All sequences must be the same from efforts to apply techniques from deep learning to length and, for the resulting models to be inter- modeling the genotype-phenotype map [60, 28]. Here pretable, must satisfy a natural notion of alignment. the emphasis has been on using the highly expressive The two inference methods supported by MAVE-NN, nature of deep neural networks to directly model ex- GE regression and NA regression, are illustrated in perimental output from input sequences. Yet it has re- Fig. 3. Each type of regression can be used to infer mained unclear how such neural networks might sepa- three different types of model: additive, neighbor, or rate out the intrinsic features of GP maps from effects of MAVE measurement processes. This is a manifes- pairwise. MAVE-NN represents each sequence as a bi- tation of the neural network interpretability problem, nary vector ~x of one-hot encoded sequence features. one that that is not addressed by established post-hoc The measurement obtained for each sequence is then attribution methods [61, 62]. assumed to depend on a latent phenotype φ, which is Here we describe a unified conceptual and com- assumed to depend the sequence via a linear model ~ ~ putational framework for the quantitative modeling φ = θ · ~x, where θ denotes model parameters. In both of MAVE data, one that unites the three strains of types of regression, MAVE-NN assumes that the mea- thought described above. As illustrated in Fig. 2, surement of each sequence is a function of a latent we assume that each sequence has a well-defined la- phenotype φ. This function is inferred from data using tent phenotype, of which the MAVE provides a noisy the dense subnetworks shown in Fig. 3a and 3c. Ex- nonlinear readout. To remove potentially confound- amples of these functions are shown in Fig. 3b and 3d.
Details
-
File Typepdf
-
Upload Time-
-
Content LanguagesEnglish
-
Upload UserAnonymous/Not logged-in
-
File Pages15 Page
-
File Size-