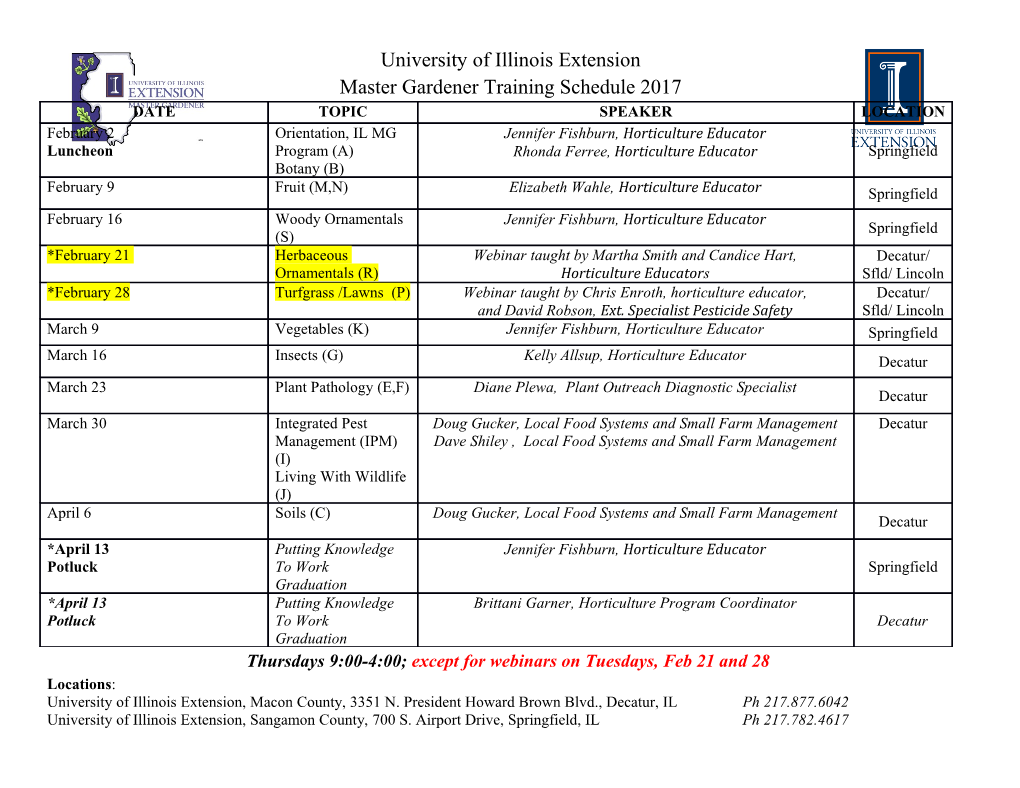
This lecture will be recorded!!! Welcome to DS595/CS525 Reinforcement Learning Prof. Yanhua Li Time: 6:00pm –8:50pm R Zoom Lecture Fall 2020 Quiz 3 today Week 7 (10/15 R) v Model-Free Control § 30 min at the beginning Quiz 4 next Thursday Week 8 (10/22 R) v Linear Value Function Approximation (20 mins) § Stochastic Gradient Decent § VFA for policy evaluation § VFA for control Last Lecture v Value Function Approximation VFA § Introduction § VFA for Policy Evaluation § VFA for Control This Lecture v Non-linear value function approximation § Intro of Deep Reinforcement Learning (DRL) § Review on Deep Learning § Deep Q-Learning § Project 3 (by Yingxue) • Pytorch configuration and Google cloud environment This Lecture v Non-linear value function approximation § Intro of Deep Reinforcement Learning (DRL) § Review on Deep Learning § Deep Q-Learning § Project 3 (by Yingxue) • Pytorch configuration and Google cloud environment RL algorithms Tabular Representation Function Representation Value Function Value Function v Model-based Model-Free Policy function control Control approximation § Policy § Policy evaluation (DP) evaluation Value Function MC (First/every approximation visit) and TD Linear/Non-linear § Policy iteration § Value/Policy § Value iteration Iteration (Asynchronous) • MC Iteration Advantage Actor Critic: • TD Iteration – SARSA A2C – Q-Learning A3C – Double Q- Learning Reinforcement Inverse Learning Reinforcement Learning Tabular representation of reward Linear reward function learning Model-based control Imitation learning Model-free control Apprenticeship learning (MC, SARSA, Q-Learning) Inverse reinforcement learning MaxEnt IRL Function representation of reward MaxCausalEnt IRL 1. Linear value function approx MaxRelEnt IRL (MC, SARSA, Q-Learning) 2. Value function approximation (Deep Q-Learning, Double DQN, Non-linear reward function learning Single Agent Single prioritized DQN, Dueling DQN) Generative adversarial 3. Policy function approximation imitation learning (GAIL) (Policy gradient, PPO, TRPO) 4. Actor-Critic methods Adversarial inverse reinforcement (A2C, A3C) learning (AIRL) Review of Deep Learning Review of Generative Adversarial nets As bases for non-linear function approximation (used in 2-4). Multi-Agent Reinforcement Learning Multi-Agent Inverse Reinforcement Multi-agent Actor-Critic Learning etc. MA-GAIL MA-AIRL AMA-GAIL Agents Applications Multiple Review: Value Function Approximation (VFA) v Represent a (state-action/state) value function with a parameterized function instead of a table v Vπ(s) s Vπ(s;w) w v Qπ(s,a) s Qπ(s,a;w) a w Linear Value Function Approximation (VFA) v Use features to represent both the state and action v Represent state-action value function (Q-function) v Stochastic gradient descent update: From full gradient to Stochastic gradient Linear VFA Linear VFA model-free control v Similar to model-free policy evaluation, true Q(s,a) is unknown, and so substitute a target value v In MC methods, use a return Gt as a target value Linear VFA model-free control v Similar to model-free policy evaluation, true Q(s,a) is unknown, and so substitute a target value v In MC methods, use a return Gt as a target value v For SARSA, use a target value of Linear VFA model-free control v Similar to model-free policy evaluation, true Q(s,a) is unknown, and so substitute a target value v In MC methods, use a return Gt as a target value v For SARSA, use a target value of v For Q-Learning, use a target value of Non-Linear VFA model-free control v Similar to model-free policy evaluation, true Q(s,a) is unknown, and so substitute a target value v In MC methods, use a return Gt as a target value v For SARSA, use a target value of v For Q-Learning, use a target value of Non-Linear VFA model-free control v Similar to model-free policy evaluation, true Q(s,a) is unknown, and so substitute a target value v In MC methods, use a return Gt as a target value v For SARSA, use a target value of v For Q-Learning, use a target value of Model-Free Q-Learning Control With linear/non-linear VFA Model-Free Q-Learning Control With linear VFA Model-Free Q-Learning Control with general (non-linear) VFA This Lecture v Non-linear value function approximation § Intro of Deep Reinforcement Learning (DRL) § Review on Deep Learning § Deep Q-Learning § Project 3 (by Yingxue) • Pytorch configuration and Google cloud environment Deep Learning! All the parameters of the logistic regressions are jointly learned. x z1 " “Neuron” 1 + !! z + y z2 " x2 + !# Feature Transformation Classification Neural Network Deep Learning! All the parameters of the logistic regressions are jointly learned. x z1 " “Neuron” 1 + !! z + y z2 " x2 + !# Feature TransformationSigmoid Function s (Classificationz) 1 s (z) = Neural1+ e-z Network z Deep learning attracts lots of attention. v I believe you have seen lots of exciting results before. Deep learning trends at Google. Source: SIGMOD 2016/Jeff Dean Neural Network + s (z) + s (z) + s (z) + s (z) “Neuron” Neural Network Different connection leads to different network structures Network parameter !: all the weights and biases in the “neurons” Fully Connect Feedforward Network neuron Input Layer Layer Layer Output 1 2 L y x1 …… 1 x2 …… y2 …… …… …… …… …… xN …… yM Input Output Layer Hidden Layer Layers Deep = Many hidden layers 152 layers Special structure 3.57% 7.3% 6.7% 16.4% AlexNet VGG GoogleNet Residual Net Taipei (2012) (2014) (2014) (2015) 101 Example Application Input Output 0.1y1 is 1 x1 x 2 0.7y2 is 2 The image …… …… …… is “2” 0.2y is 0 x256 10 16 x 16 = 256 Ink → 1 Each dimension represents No ink → 0 the confidence of a digit. Example Application v Handwriting Digit Recognition x1 y1 is 1 x2 y2 is 2 …… …… MachineNeural “2…… Network ” x 256 What is needed is a y10 is 0 function …… Input: output: 256-dim 10-dim vector vector Example Application Input Layer Layer Layer Output 1 2 L x1 …… y1 is 1 x 2 …… A function set containing the y2 is 2 …… …… …… …… …… candidates for “2…… Handwriting Digit Recognition ” x …… N y10 is 0 Input Output Layer Hidden Layer Layers You need to decide the network structure to let a good function in your function set. Loss for an Example target “1 ” …… #" x1 y1 ! 1 x Softmax 2 #" Given a set ……of y2 " 0 …… …… …… …… …… parameters …… Cross Entropy x 256 …… y10 #"!# 0 !& # #% " # , #% = − ( #)$"*#$ $%! Softmax (3 classes as example) Probability: n 1 > #$ > 0 n ∑$ #$ = 1 yi = P(Ci | x) Softmax 3 3 z 20 0.88 z z j e 1 1 z1 e ÷ y1 = e åe j=1 0.12 3 1 z 2.7 z z j z e 2 2 2 e ÷ y2 = e åe j=1 0.05 ≈0 3 - z3 z z z3 e e ÷ 3 j 3 y3 = e åe 3 j=1 + åez j j=1 Total Loss Total Loss: ( + = ( "' For all training data '%! … x1 NN y1 #'! (! Find a function in x2 NN y2 #'" (" function set that minimizes total loss L x3 NN y3 #'& & …… …… ( …… …… Find the network ∗ xN NN yN #'% parameters , that (% minimize total loss L Gradient Descent - Compute +,⁄+)! .+ )! 0.2 0.15 −. +,⁄+)! ./! .+ Compute +,⁄+)" ) " -0.1 0.05 2+ = ./# … … −. +,⁄+)" ⋮ .+ Compute +,⁄+/ ! .1! /! 0.3 0.2 ⁄ ⋮ … … −. +, +/! gradient Gradient Descent - Compute +,⁄+)! Compute +,⁄+)! )! 0.2 0.15 0.09 ⁄ −. +,⁄+)! −. +, +)! … Compute +,⁄+)" Compute +,⁄+)" … )" -0.1 0.05 0.15 … … −. +,⁄+) −. +,⁄+)" " … … Compute +,⁄+/! Compute +,⁄+/! /! 0.3 0.2 0.10 ⁄ −. +,⁄+/! … … −. +, +/! … … Backpropagation v Backpropagation: an efficient way to compute +,⁄+) in neural network libdnn Why Deep Learning? Deep models are better than shadow models? Take DS504 in Spring 2020 This Lecture v Non-linear value function approximation § Intro of Deep Reinforcement Learning (DRL) § Review on Deep Learning § Deep Q-Learning § Project 3 (by Yingxue) • Pytorch configuration and Google cloud environment Generalization with Deep Reinforcement Learning v Using function approximation to help scale up to making decisions in really large domains Generalization with Deep Reinforcement Learning v Use deep neural networks to represent § Value function (Deep Q-Learning, etc.) § Policy function (Policy Gradient, etc.) § Both value function and policy (A2C, A3C) v Optimize loss function by stochastic gradient descent (SGD) Recall: Non-Linear VFA model-free control v Similar to model-free policy evaluation, true Q(s,a) is unknown, and so substitute a target value v In MC methods, use a return Gt as a target value v For SARSA, use a target value of v For Q-Learning, use a target value of Recall: Model-Free Q-Learning Control with general (non-linear) VFA ??????????Going to work? https://deepmind.com/research/open-source/dqn - - - Breakout game demo https://www.youtube.com/watch?v=TmPfTpjtdgg Recall: Version 1 * * https://papers.nips.cc/paper/3964-double-q-learning.pdf - Example: TD policy evaluation s1 s2 s3 s4 s5 s6 a1 a2 Taxi passenger-seeking process: R=[1,0,0,0,3,0] For any action, π(s) = a1, ∀s, γ = 1. any action from s1 and s6 terminates episode Given (s3, a1, 0, s3, a1, 0, s2, a1, 0, s1, a1, 1, T); Q1: First visit MC estimate of V of each state? [111000] Q2: TD estimate of states (init at 0) with α=1? [100000] Q3: Now get to chose 2 ”replay” backups to do. Which should we pick to get best estimate? Example: TD policy evaluation s1 s2 s3 s4 s5 s6 a1 a2 Taxi passenger-seeking process: R=[1,0,0,0,3,0] For any action, π(s) = a1, ∀s, γ = 1. any action from s1 and s6 terminates episode Given (s3, a1, 0, s3, a1, 0, s2, a1, 0, s1, a1, 1, T); Q1: First visit MC estimate of V of each state? [111000] Q2: TD estimate of states (init at 0) with α=1? [100000] Q3: Now get to chose 2 ”replay” backups to do.
Details
-
File Typepdf
-
Upload Time-
-
Content LanguagesEnglish
-
Upload UserAnonymous/Not logged-in
-
File Pages69 Page
-
File Size-