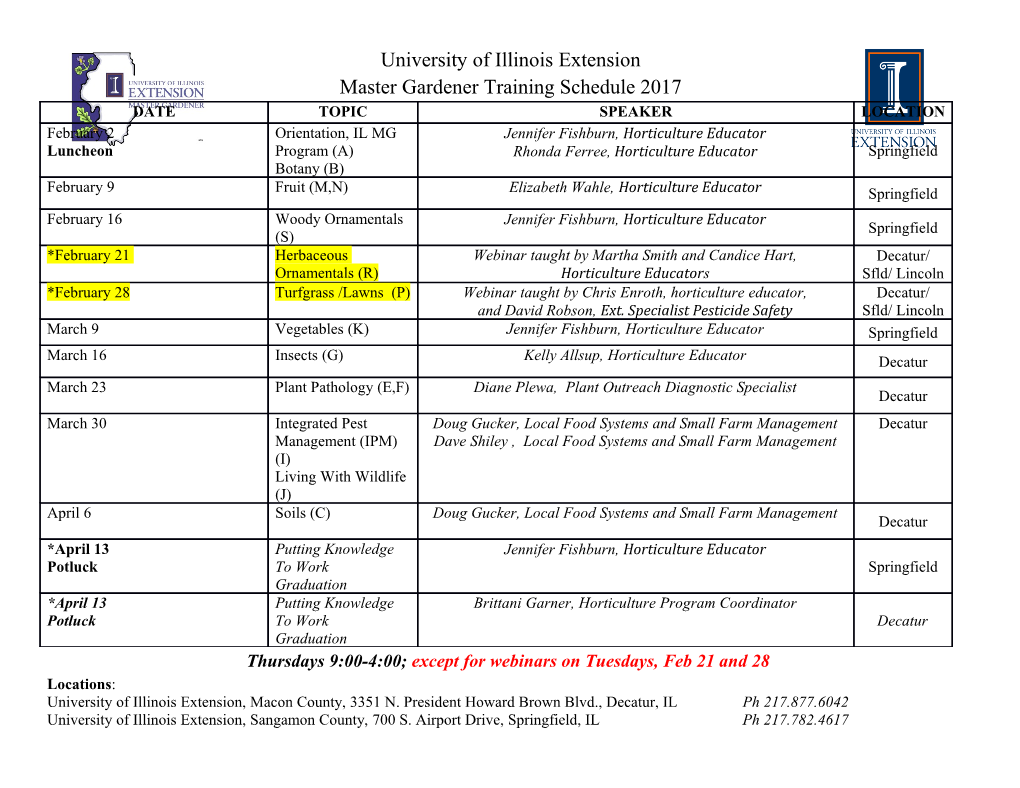
Floor Plan Generation and Room Labeling of Indoor Environments from Laser Range Data Eric Turner and Avideh Zakhor Department of Electrical Engineering and Computer Sciences, University of California, Berkeley, CA, U.S.A. Keywords: Floor Plan, Watertight Modeling, Range Data, LiDAR. Abstract: Automatic generation of building floor plans is useful in many emerging applications, including indoor naviga- tion, augmented and virtual reality, as well as building energy simulation software. These applications require watertight models with limited complexity. In this paper, we present an approach that produces 2.5D extruded watertight models of building interiors from either 2D particle filter grid maps or full 3D point-clouds captured by mobile mapping systems. Our approach is to triangulate a 2D sampling of wall positions and separate these triangles into interior and exterior sets. We partition the interior volume of the building model by rooms, then simplify the model to reduce noise. Such labels are useful for building energy simulations involving thermal models, as well as for ensuring geometric accuracy of the resulting 3D model. We experimentally verify the performance of our proposed approach on a wide variety of buildings. Our approach is efficient enough to be used in real-time in conjunction with Simultaneous Localization and Mapping (SLAM) applications. 1 INTRODUCTION advantages. As shown later, it is possible to gener- ate sizable 2.5D extruded models at real-time speeds, Indoor building modeling and floor plan generation enabling human operators to capture and navigate en- are useful in many fields such as architecture and civil vironments thoroughly and adaptively. engineering. Green buildings and sustainable con- We also propose a technique to partition the inte- struction have increased the use of building energy rior environment rooms, yielding secondary features simulation and analysis software, requiring building of buildings, such as locations of doorways. Room geometry as input. Even though existing energy simu- labeling is useful for many applications, such as fast lation tools can accurately model the thermodynamic rendering of models (Funkhouser et al., 1992). Fur- properties of building interiors, their performance is thermore, since energy simulation engines model heat hindered by overly complex geometry models (Craw- and air flow within the building environment, they ley et al., 2000). Indoor models can also be used need accurate partitions of the interior spaces to rep- for positioning in wide-area augmented reality appli- resent distinct thermal zones (Crawley et al., 2000). cations, whereby low-complexity models enable low In addition to exporting room labels, our proposed memory use for mobile client-side processing. technique uses the computed labels to further improve In this paper, we present a technique for gener- the geometry of the model. Specifically, knowledge ating aesthetically pleasing, minimalist 2.5D models of room partitions can be exploited to reduce noise of indoor building environments. Such models are in- in the computed geometry while preserving fine de- tended to capture the architectural elements of a build- tails in doorways. Furthermore, since input height es- ing such as floors, walls, and ceilings while ignoring timates are often noisy, using room labels to group transient objects such as furniture. We generate our these heights can provide substantial error reduction models by first computing a 2D floor-plan of the en- in the resulting extruded 3D meshes. vironment, then using estimated height information to This paper is organized as follows. In Section 2, extrude the floor-plan into a 3D building model. we describe related work to this research. Section 3 Generating 3D models by extruding 2D floor- describes our proposed algorithm to generate floor plans typically yield clean and aesthetically pleasing plans from the specified input. In Section 4, we de- results. Even though such models may not capture the scribe our approach to room labeling. In Section 5, fine details of the environment, they still offer many we show how room labeling is used to reduce noise 22 Turner E. and Zakhor A.. Floor Plan Generation and Room Labeling of Indoor Environments from Laser Range Data. DOI: 10.5220/0004680300220033 In Proceedings of the 9th International Conference on Computer Graphics Theory and Applications (GRAPP-2014), pages 22-33 ISBN: 978-989-758-002-4 Copyright c 2014 SCITEPRESS (Science and Technology Publications, Lda.) FloorPlanGenerationandRoomLabelingofIndoorEnvironmentsfromLaserRangeData in the model. Section 6 describes how 2D floor plans including doorways. Our approach generates a 2D are extruded into 2.5D models with height informa- floor plan of the building, then uses wall height infor- tion. Section 7 demonstrates experimental results on mation to generate a 3D extrusion of this floor plan. a wide variety of building models. Lastly, in Section 8 Such blueprint-to-model techniques have been well- we describe potential future work in this area. studied (Or et al., 2005; Lewis and Sequin, 1998), but rely on the original building blueprints as input. Our technique automatically generates the floor plan of the building and uses this information to create a 2 BACKGROUND 2.5D model of the environment. Prior work on automatic floor plan generation use Modeling and navigation of indoor environments is a dense 3D point-clouds as input, and take advantage of well-studied field. Due to cost of full 3D laser range the verticality of walls to perform histogram analysis finders, the majority of indoor modeling systems use to sample wall position estimates (Okorn et al., 2009; 2D LiDAR scanners. Examples of such systems in- Turner and Zakhor, 2012), which are in the same for- clude autonomous unmanned vehicles (Shen et al., mat as a grid map for particle filtering (Grisetti et al., 2011; Brunskill et al., 2007) or systems worn by a hu- 2005). In situations where dense 3D point-clouds are man operator (Chen et al., 2010; Fallon et al., 2012). available, we apply similar techniques to convert them Most simultaneous localization and mapping to a 2D wall sampling. (SLAM) systems use a horizontally-oriented 2D Li- A novel contribution of this paper is the use of DAR scanner, which estimates the trajectory of the room labeling to enhance building models, e.g. for system, creating a 2D map of the environment (Thrun thermal simulations of interior environments (Craw- et al., 2005). The constructed 2D grid map is stored ley et al., 2000). One motivation for existing work has 2 as a set of points in R that represent the primary been to capture line-of-sight information for fast ren- features of the environment, such as walls and build- dering of building environments (Funkhouser et al., ing architecture. Particle filtering approaches to local- 1992). This technique requires axis-aligned rectilin- ization typically result in real-time mapping (Hahnel ear building geometry, which often is not a valid as- et al., 2003; Grisetti et al., 2007) and can therefore sumption. Others have partitioned building environ- benefit from a real-time floor plan generation algo- ments into submap segments with the goal of efficient rithm that delivers a live map of the environment. localization and tracking (Brunskill et al., 2007). This These mapping systems can also use additional approach is meant to create easily recognizable sub- scanners to create a dense 3D point-cloud representa- sections of the environment, whereas our proposed tion of the environment geometry (Smith et al., 2011; room labeling technique uses geometric features to Kua et al., 2012), which can be used to develop full capture semantic room definitions for both architec- 3D models (Chauve et al., 2010; Holenstein et al., tural and building energy simulation applications. 2011). Many applications are unable to use these 3D models due to their complexity and number of ele- ments. For example, building energy simulations re- quire watertight meshes that are also highly simplified 3 FLOOR PLAN GENERATION in order to perform effectively (Crawley et al., 2000). To address this issue, a number of simplified In this section, we present a technique to automati- building modeling algorithms have been developed, cally generate accurate floor plan models at real-time most of which assume vertical walls, rectified rooms, speeds for indoor building environments. Section 3.1 and axis-alignment (Xiao and Furukawa, 2012). Un- describes the type of input for our approach, which der these assumptions, fundamental features of the can be generated from either 2D mapping systems building can be identified, while ignoring minor de- or dense 3D point-clouds of environments. In Sec- tails such as furniture or other clutter (Adan and Hu- tion 3.2, we discuss the way these input data are used ber, 2011). One of the major limitations of these to compute the interior space of the 2D floor-plan, techniques is that they are developed only for axis- which defines the resultant building geometry. aligned models. Often, such techniques correctly re- construct major rooms while fundamentally changing 3.1 Input Data the topology of minor areas, such as ignoring door- ways, shapes of rooms, or small rooms entirely. The input data used during floor plan generation con- In this paper, we show that simple models can be sist of points in the (x,y) horizontal plane, which generated with only 2.5D information, while preserv- we call wall samples. These points depict loca- ing connectivity and geometry of building features, tions of walls or vertical objects in the environ- 23 GRAPP2014-InternationalConferenceonComputerGraphicsTheoryandApplications the leaf is considered a wall sample. The value of H may vary depending on application, but a length of 2 meters works well to capture permanent wall features while ignoring furniture and other interior clutter. The result is a set of wall samples P ⊆ R2, where each wall sample p 2 P is represented by its 2D po- sition, the minimum and maximum height values of the points that sample represents, and the poses of the scanners that observed the sample location.
Details
-
File Typepdf
-
Upload Time-
-
Content LanguagesEnglish
-
Upload UserAnonymous/Not logged-in
-
File Pages12 Page
-
File Size-