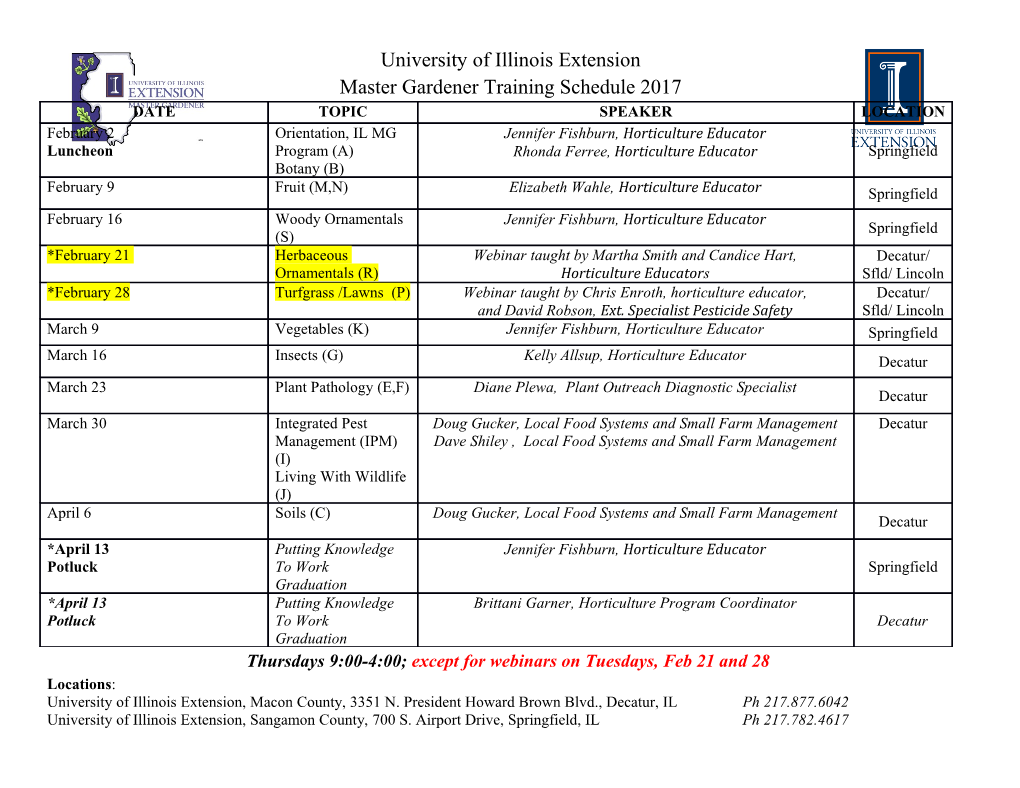
Iowa State University Capstones, Theses and Retrospective Theses and Dissertations Dissertations 1994 Some problems with nonsampling errors in receiver operating characteristic studies Marie Ann Coffin Iowa State University Follow this and additional works at: https://lib.dr.iastate.edu/rtd Part of the Statistics and Probability Commons Recommended Citation Coffin,a M rie Ann, "Some problems with nonsampling errors in receiver operating characteristic studies " (1994). Retrospective Theses and Dissertations. 10591. https://lib.dr.iastate.edu/rtd/10591 This Dissertation is brought to you for free and open access by the Iowa State University Capstones, Theses and Dissertations at Iowa State University Digital Repository. It has been accepted for inclusion in Retrospective Theses and Dissertations by an authorized administrator of Iowa State University Digital Repository. For more information, please contact [email protected]. UMI MICROFILMED 1994 INFORMATION TO USERS This manuscript has been reproduced from the microfilm master. UMI films the text directly firom the original or copy submitted. Thus, some thesis and dissertation copies are in typewriter face, while others may be from any type of computer printer. The quality of this reproduction is dependent upon the quality of the copy submitted. Broken or indistinct print, colored or poor quality illustrations and photographs, print bleedthrough, substandard margins, and improper alignment can adversely affect reproduction. In the unlikely event that the author did not send UMI a complete manuscript and there are missing pages, these will be noted. Also, if unauthorized copyright material had to be removed, a note will indicate the deletion. Oversize materials (e.g., maps, drawings, charts) are reproduced by sectioning the original, beginning at the upper left-hand comer and continuing firom left to right in equal sections with small overlaps. Each original is also photographed in one exposure and is included in reduced form at the back of the book. Photographs included in the original manuscript have been reproduced xerographically in this copy. Higher quality 6" x 9 " black and white photographic prints are available for any photographs or illustrations appearing in this copy for an additional charge. Contact UMI directly to order. University Microlilms International A Bell & Howell Information Company 300 North Zeeb Road. Ann Arbor. Ml 48106-1346 USA 313/761-4700 800/521-0600 r Order Number 9424205 Some problems with nonsampling errors in receiver operating characteristic studies Coffin, Marie Ann, Ph.D. Iowa State University, 1994 UMI 300 N. Zeeb Rd. Ann Arbor, MI 48106 L- F Some problems with nonsampling errors in receiver operating characteristic studies by Marie Ann Coffin A Dissertation Submitted to the Graduate Faculty in Partial Fulfillment of the Requirements for the Degree of DOCTOR OF PHILOSOPHY Department: Statistics Major: Statistics Approved: Signature was redacted for privacy. In Charge of Major Work Signature was redacted for privacy. For the Major Department Signature was redacted for privacy. For the Graduate College Iowa State University Ames, Iowa 1994 ii TABLE OF CONTENTS ACKNOWLEDGEMENTS X ABSTRACT xi CHAPTER 1. INTRODUCTION 1 1.1 The ROC curve 1 1.2 The total area index 3 1.3 Nonparametric estimation of 0 4 1.4 The partial area index 6 1.5 Measurement errors 7 1.6 Nonparametric estimation of the total area 8 1.7 Other sources of bias S 1.8 The partial area index 9 1.9 Parametric estimation oi 0 10 CHAPTER 2. MEASUREMENT ERRORS AND THE TOTAL AREA INDEX 11 2.1 Introduction 11 2.2 The model with measurement errors 13 2.2.1 Assumptions 13 2.3 An expression for the bias 14 m 2.4 Estimating the bias caused by measurement errors 17 2.4.1 Resampling methods to estimate the bias 17 2.4.2 Overview of kernel esimation 18 2.4.3 The kernel estimates of fx and gy 21 2.4.4 Estimating fx and gy 22 2.4.5 Estimating the bias 24 2.5 Monte Carlo study 25 2.6 Multiple observations 29 2.7 Application: Analysis of PKU data 30 CHAPTER 3. OBSERVER BIASES AND THE TOTAL AREA INDEX 34 3.1 Introduction 34 3.2 One observer with constant bias 35 3.2.1 The model with a single observer 35 3.2.2 Estimating 9 for this model 35 3.3 Several observers 37 3.3.1 The model with several observers 37 3.3.2 Estimating the bias ofÔi 37 3.3.3 Estimating 0 when bias estimates are available 41 3.3.4 Finding the bias of #2 41 3.3.5 Estimating the densities of the unobserved variables 43 3.4 Repeated observations 43 3.4.1 The model 44 3.4.2 Finding the bias of #3 44 iv 3.4.3 Estimating the bias of 03 46 3.5 Estimating 9 when bias estimates are not available 47 3.5.1 Stratifying the sample 47 3.5.2 Multiple observations 50 CHAPTER 4. NONPARAMETRÎC ESTIMATION OF THE PAR­ TIAL AREA INDEX 54 4.1 Introduction 54 4.2 An expression for 0c 55 4.3 Estimating Oc nonparametrically 56 4.3.1 Estimating (1 — c) 56 4.3.2 The bias of#c 57 4.4 Assessing the bias oi 9^ 58 4.4.1 Normally distributed separator variable 59 4.4.2 A skewed separator variable 60 4.5 Developing a linear model for PBIAS 61 4.6 Implications of the linear model 62 4.7 Conclusion 64 CHAPTER 5. A PARAMETRIC APPROACH TO MEASURE­ MENT ERRORS 84 5.1 Introduction 84 5.2 Rating data approach 84 5.3 A normal separator variable 86 5.3.1 The model with measurement errors 87 5.3.2 An expression for the bias 89 5.3.3 Estimating B 91 5.3.4 Estimating the measurement error variance 92 5.4 The partial area index: normal model 93 5.5 The exponential model 96 5.5.1 Measurement errors in the exponential model 99 5.6 Partial area index for the exponential model 101 REFERENCES 103 APPENDIX A. PKU DATA 108 APPENDIX B. JACKKNIFE ESTIMATE OF BIAS Ill APPENDIX C. A SOLUTION TO THE FREDHOLM EQUATION 114 vi LIST OF TABLES Table 2.1; 10,000 Monte Carlo simulations, X ~ iV(S,2), Y ~ iV(12,2), e~ iV(0,l). 0 = 0.9772499 26 Table 2.2: 10,000 Monte Carlo simulations, A' ~ vV(8,2), Y ~ A'(10,2), e ~ #(0,1). 0 = 0.8413447 26 Table 2.3: 10,000 Monte Carlo simulations, X GAM(2,1), Y ~ 3 + GAM(2,1), iV(0,l). 6 = 0.9378457 28 Table 2.4: 10,000 Monte Carlo simulations, X ~ GAM(2,1), i'' ~ 2 + GAM(2,1), e~ .'V(0,1). 0 = 0.8647443 28 Table 5.1: 1,000 Monte Carlo simulations, A' ~ N0R(4,2), F ~ NOR(7,2), e ~ NOR(0,0.25) 95 L: vii LIST OF FIGURES Figure 2.1; Kernel estimates of fx{-) and gvi-) 33 Figure 4.1: Proportion of bias in using §c to estimate 9^ r = 1.34, X and Y normally distributed. (The number accompanying each curve is the value of m.) 65 Figure 4.2: Proportion of bias in using Oc to estimate 6^, r = 1.5, X and Y normally distributed.(The number accompanying each curve is the value of m.) 66 Figure 4.3: Proportion of bias in using Ôc to estimate t = 1-73, X and Y normally distributed. (The number accompanying each curve is the value of m.) 67 Figure 4.4: Proportion of bias in using 9c to estimate 9c, t = 2.12,X and Y normally distributed. (The number accompanying each curve is the value of m.) 68 Figure 4.5: Proportion of bias in using Ôc to estimate 6c, r = 3, X and Y normally distributed. (The number accompanying each curve is the value of m.) 69 f vin Figure 4.6: Proportion of bias in using Oc to estimate 9c, r = 0.71, X and Y gamma. (The number accompanying each curve is the value of m.) 70 Figure 4.7: Proportion of bias in using 6c to estimate 9c, t = 1.41, X and Y gamma.(The number accompanying each curve is the value of m.) 71 Figure 4.8: Proportion of bias in using 9c to estimate Oc, r = 2.12, X and Y gamma.(The number accompanying each curve is the value of m.) 72 Figure 4.9: Proportion of bias in using 9c to estimate 9c, t = 2.83, X and Y gamma. (The number accompanying each curve is the value of m.) 73 Figure 4.10: Proportion of bias in using 9c to estimate 9c, t = 3.54, X and Y gamma. (The number accompanying each curve is the value of m.) 74 Figure 4.11: Proportion of bias in using 9c to estimate Oc, r = 4.24, X and Y gamma. (The number accompanying each curve is the value of m.) 75 Figure 4.12: Relationship between «i and m at different levels of r, X and Y normally distributed. (The number accompanying each curve is the value of r.) 76 Figure 4.13: Relationship between b\ and m at different levels of r, X and Y normally distributed. (The number accompanying each curve is the value of T.) 77 ix Figure 4.14: Relationship between ai and m at different levels of r, X and Y gamma. (The number accompanying each curve is the value of T.) 78 Figure 4.15: Relationship between (z% and m at different levels of r, X and Y gamma. (The number accompanying each curve is the value of r.) 79 Figure 4.16: Relationship between og and r for W = 0(X and Y normal), 1(X and Y gamma) 80 Figure 4.17: Relationship between as and r for VV = 0(X and Y normal) , 1(X and Y gamma) 81 Figure 4.18: Relationship between 62 and r for VV = 0(X and Y normal), 1(X and Y gamma) 82 Figure 4.19: Relationship between 63 and T for W = 0 (X and Y normal), 1(X and Y gamma) 83 ACKNOWLEDGEMENTS I would like to thank my major professor, Dr.
Details
-
File Typepdf
-
Upload Time-
-
Content LanguagesEnglish
-
Upload UserAnonymous/Not logged-in
-
File Pages135 Page
-
File Size-