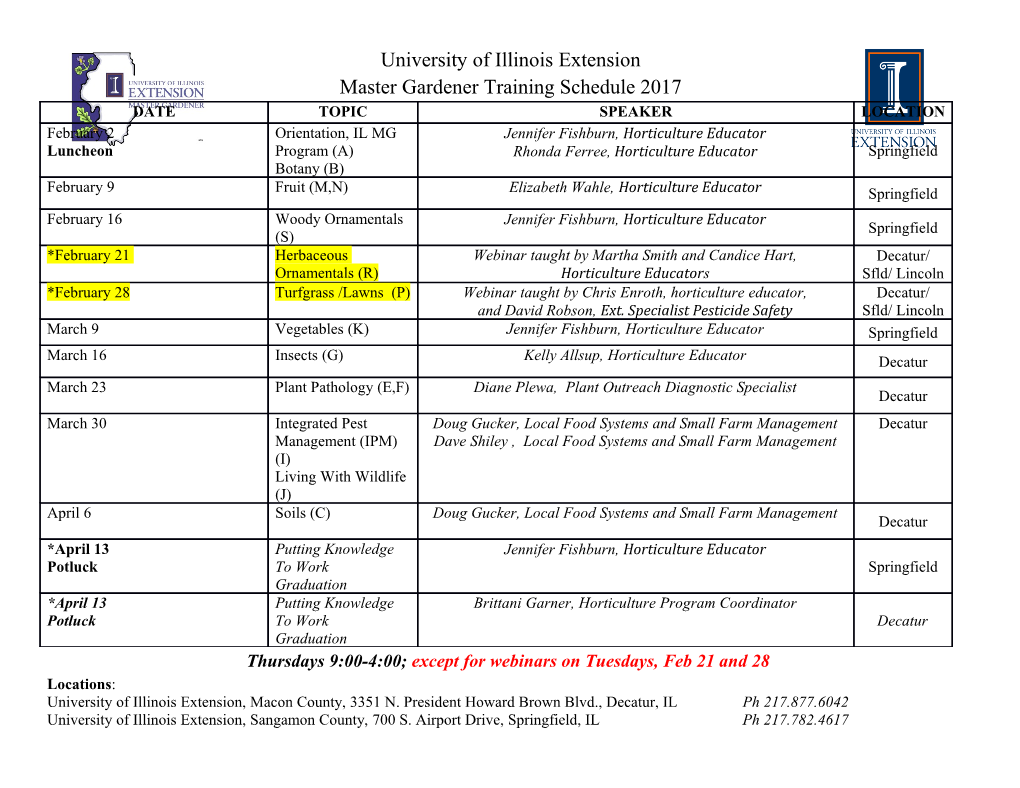
Some Expectations of a Non-Central Chi-Square Distribution With an Even Number of Degrees of Freedom Stefan M. Moser Department of Communication Engineering National Chiao Tung University (NCTU) Hsinchu, Taiwan Abstract—The non-central chi-square distribution plays an In this paper we will state closed-form expressions for important role in communications, for example in the analysis of some of these situations: we will give closed-form solutions mobile and wireless communication systems. It not only includes 1 to E[ln V ] and E n for a non-central chi-square random the important cases of a squared Rayleigh distribution and a V squared Rice distribution, but also the generalizations to a sum variable V with an even number of degrees of freedom. Note of independent squared Gaussian random variables of identical that in practice we often have an even number of degrees variance with or without mean, i.e., a “squared MIMO Rayleigh” of freedom because we usually consider complex Gaussian and “squared MIMO Rice” distribution. random variables consisting of two real Gaussian components. In this paper closed-form expressions are derived for the We will see that these expectations are all related to a family of expectation of the logarithm and for the expectation of the n-th gm(·) power of the reciprocal value of a non-central chi-square random functions that is defined in Definition 2 in the following variable. It is shown that these expectations can be expressed by section. There we will also state the main results. In Section III a family of continuous functions gm(·) and that these families we will then derive some properties of the functions gm(·) and have nice properties (monotonicity, convexity, etc.). Moreover, in Section IV we will state tight upper and lower bounds. In some tight upper and lower bounds are derived that are helpful Section V another property of gm(·) is derived as an example in situations where the closed-form expression of gm(·) is too complex for further analysis. of how the bounds from Section IV could be applied. We conclude in Section VI. Due to space limitations we cannot I. INTRODUCTION provide the complete proofs. For the proofs and more details we refer to [3], [4]. It is well known that adding several independent squared Gaussian random variables of identical variance yields a II. DEFINITIONS AND MAIN RESULTS random variable that is non-central chi-square distributed. A non-negative real random variable is said to have a non- This distribution often shows up in information theory and central chi-square distribution with n degrees of freedom and 2 communications. As an example we mention the situation of non-centrality parameter s if it is distributed like a non-coherent multiple-input multiple-output (MIMO) fading n 2 channel (Xj + μj) , (2) Y = Hx + Z (1) j=1 n where {Xj}j=1 are IID ∼NR (0, 1) and the real constants with an additive white Gaussian noise vector Z and with a n {μj}j=1 satisfy fading matrix H that consists of independent and unit-variance n 2 2 Gaussian distributed components with or without mean. Here s = μj . (3) conditional on the input x the squared magnitude of the output j=1 Y2 is non-central chi-square distributed. (The distribution of (2) depends on the constants {μj} only 1 While the special case of a squared Rayleigh distribution via the sum of their squares.) The probability density function is well understood in the sense that there exist closed-form of such a distribution is given by [5, Chapter 29] expressions for more or less all interesting expected values, the −2 n 2 √ 1 x 4 − s +x more general situation of a non-central chi-square distribution 2 ≥ 0 2 e In/2−1 s x ,x. (4) is far more complex. Here, standard integration tools (e.g.,[1]) 2 s or integration lookup tables (e.g., [2]) will very quickly cease Here Iν (·) denotes the modified Bessel function of the first to provide closed-form expressions. kind of order ν ∈ R, i.e., ∞ 1 x ν+2k This work was supported by the Industrial Technology Research Institute ( ) ≥ 0 Iν x !Γ( + +1) 2 ,x (5) (ITRI), Zhudong, Taiwan, under JRC NCTU-ITRI and by the National Science k=0 k ν k Council under NSC 95-2221-E-009-046. 1A squared Rayleigh random variable is actually exponentially distributed. (see [2, Eq. 8.445]). If the number of degrees of freedom n is even, i.e.,if (see [6, Eq. (417)], [7, Eq. (A.39)]). Note that gm(·) is also n =2m for some positive integer m, then the non-central continuous, i.e., in particular chi-square distribution can also be expressed as a sum of the ⎧ ⎛ ⎞⎫ ⎨ m−1 ⎬ squared norms of complex Gaussian random variables. (−1)mΓ(m) (−1)j lim ⎝e−ξ − ξj⎠ Definition 1: Let the random variable V have a non-central ξ↓0 ⎩ m ! ⎭ ξ j=0 j chi-square distribution with an even number 2m of degrees of 1 freedom, i.e., = = gm(0). (13) m m 2 V Uj + μj (6) Now we will give closed-form expressions for some expec- j=1 tations of a non-central chi-square random variable. We start m m with the logarithm. where {Uj}j=1 are IID ∼NC(0, 1),and{μj}j=1 are complex 2 Theorem 3: The expected value of the logarithm of a non- constants. Let further the non-centrality parameter s be central chi-square random variable with an even number 2m defined as of degrees of freedom is given as m 2 2 s |μj| . (7) 2 E[ln V ]=gm(s ) (14) j=1 V s2 Next we define the following continuous functions. where and are defined in (6) and (7). Hence, we have (·) found the solution to the following integral: Definition 2: The functions gm are defined as follows: ∞ m−1 √ ⎧ ln · v 2 −v−s2 (2 ) = ( 2) ⎪ m−1 v 2 e Im−1 s v dv gm s (15) ⎪ j −ξ 0 s ⎪ln(ξ) − Ei(−ξ)+ (−1) e (j − 1)! ⎪ 2 ⎪ j=1 for any m ∈ N and s ≥ 0. ⎨ ( ) ( − 1)! 1 j Proof: A proof can be found in [6, Lemma 10.1], [7, gm ξ ⎪ − m 0 ⎪ ( − 1 − )! ,ξ>Lemma A.6] ⎪ j m j ξ ⎪ Next we look at the reciprocal value. ⎩⎪ ψ(m),ξ=0 Theorem 4: Let n ∈ N with n<m. The expected value of (8) the n-th power reciprocal value of a non-central chi-square for m ∈ N, where Ei(·) denotes the exponential integral random variable with an even number 2m of degrees of function defined as freedom is given as ∞ e−t 1 (−1)n−1 Ei(− ) − 0 = · (n) ( 2) ξ dt, ξ > (9) E n gm−n s ,m>n (16) ξ t V (n − 1!) and ψ(·) is Euler’s psi function given by where () ∂ gm(ξ) gm (ξ)= (17) m−1 1 ∂ξ ( ) − + ∈ N ψ m γ ,m (10) 2 j=1 j denotes the -th derivative of gm(·) and where V and s are defined in (6) and (7). In particular, for m>1 with γ ≈ 0.577 denoting Euler’s constant. 1 Note that gm(ξ) is continuous for all ξ ≥ 0, i.e., in particular 2 E = gm−1(s ). (18) V m−1 lim ln(ξ) − Ei(−ξ)+ (−1)j e−ξ(j − 1)! Hence, we have found the solution to the following integral: ξ↓0 j=1 ∞ m−1 1 2 2 √ j · v −v−s (2 ) (m − 1)! 1 n 2 e Im−1 s v dv − = ψ(m) (11) 0 v s j(m − 1 − j)! ξ n−1 (−1) (n) 2 = · gm−n(s ) (19) (n − 1!) for all m ∈ N. Therefore its first derivative is defined for all 2 ξ ≥ 0 and can be evaluated to for any m, n ∈ N, m>n, and any real s ≥ 0. ⎛ ⎞ Note that in the cases where m ≤ n the expectation is ( ) (−1)mΓ( ) m−1 (−1)j unbounded. ∂gm ξ m ⎝ −ξ j⎠ gm(ξ) = e − ξ Proof: The proof is based on a series expansion of ∂ξ ξm j! j=0 the modified Bessel function similarly to the proof of [6, (12) Lemma 10.1], [7, Lemma A.6]. For more details see [3]. III. PROPERTIES OF gm(·) AND gm(·) Theorem 9: We have the following relations: In this section we will show that the family of functions gm+1(ξ)=gm(ξ)+gm(ξ) (27) gm(·) g (·) and m are well-behaved. for all m ∈ N and all ξ ≥ 0,and Corollary 5: The functions gm(·) are monotonically strictly 1 m increasing and strictly concave in the interval [0, ∞) for all gm+1(ξ)= − gm(ξ),ξ>0, (28) ξ ξ m ∈ N. 1 ξ Proof: From [6, Eqs. (415), (416)], [7, Eqs. (A.37), and gm(ξ)= − gm+1(ξ),ξ≥ 0, (29) (A.38)] we know that the first and second derivative of gm(·) m m ∈ N can be written as for all m . Proof: These relations can be proven by algebraic rear- ∞ 1 1 ( )= −ξ · · k rangements using (20). For details we refer to [3]. gm ξ e ! + ξ , (20) k=0 k k m IV. BOUNDS ON gm(·) AND gm(·) ∞ 1 1 −ξ k In this section we derive some tight bounds on the functions gm(ξ)=−e · · ξ , (21) ! ( + )( + +1) (·) (·) k=0 k k m k m gm and gm . Theorem 10: The function gm(·) can be bounded as fol- i.e.,thefirst derivative of gm(·) is positive and the second lows: derivative is negative.
Details
-
File Typepdf
-
Upload Time-
-
Content LanguagesEnglish
-
Upload UserAnonymous/Not logged-in
-
File Pages4 Page
-
File Size-