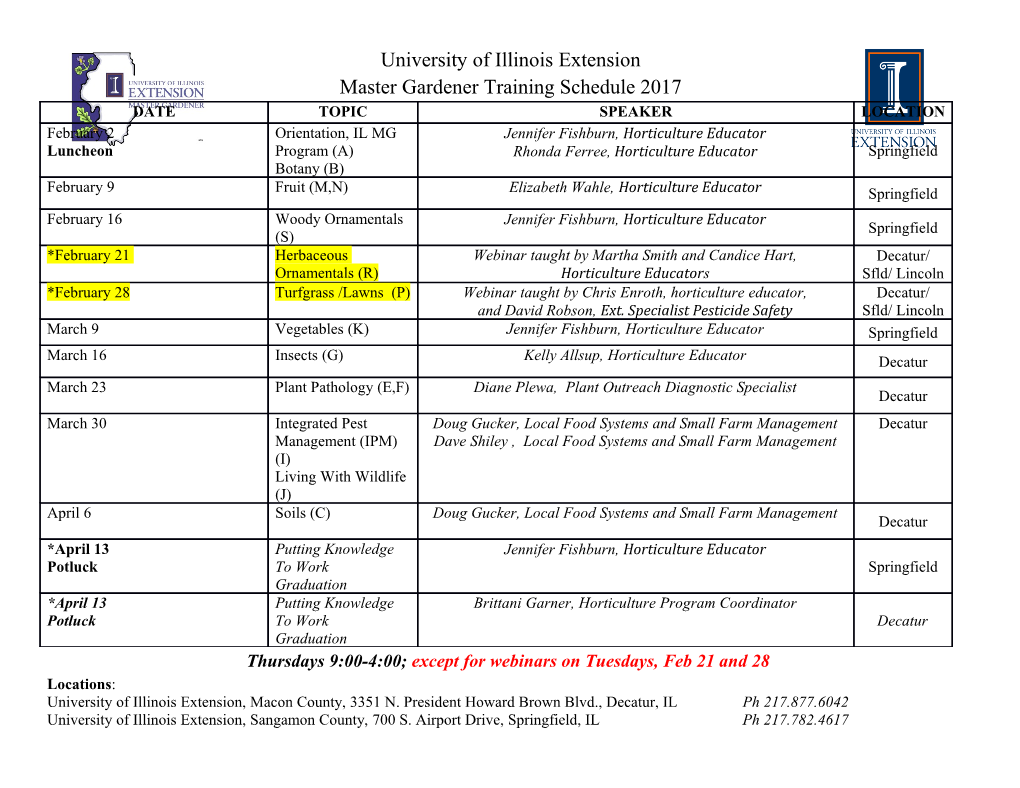
Learning to Explain Non-Standard English Words and Phrases Ke Ni and William Yang Wang Department of Computer Science University of California, Santa Barbara Santa Barbara, CA 93106 USA fke00@umailg,[email protected] Abstract We describe a data-driven approach for au- tomatically explaining new, non-standard English expressions in a given sentence, building on a large dataset that includes Figure 1: An example Tweet with a non-standard 15 years of crowdsourced examples from English expression. Our model aims at automati- UrbanDictionary.com. Unlike prior stud- cally explaining any newly emerged, non-standard ies that focus on matching keywords from expressions (generating the blue box). a slang dictionary, we investigate the pos- sibility of learning a neural sequence-to- sequence model that generates explana- tions from Wiktionary to detect satirical news ar- tions of unseen non-standard English ex- ticles. Wang and McKeown (2010) show that us- pressions given context. We propose a ing a slang dictionary of 5K terms can help detect- dual encoder approach—a word-level en- ing vandalism of Wikipedia edits. Han and Bald- coder learns the representation of context, win (2011) make use of the same slang dictionary, and a second character-level encoder to and achieve the best performance by combining learn the hidden representation of the tar- the dictionary lookup approach with word simi- get non-standard expression. Our model larity and context for Twitter and SMS text nor- can produce reasonable definitions of new malization. However, using a 5K slang dictionary non-standard English expressions given may suffer from the coverage issue, since slang their context with certain confidence. is evolving rapidly in the social media age1. Re- cently, Thanapon Noraset (2016) shows that it is 1 Introduction possible to use word embeddings to generate plau- sible definitions. Nonetheless, one weakness is In the past two decades, the majority of NLP re- that definition of a word may change within dif- search focused on developing tools for the Stan- ferent contexts. dard English on newswire data. However, the non- standard part of the language is not well-studied In contrast, we take a more radical approach: in the community, even though it becomes more we aim at building a general purpose sequence-to- and more important in the era of social media. sequence neural network model (Sutskever et al., While we agree that one must take a cautious ap- 2014) to explain any non-standard English expres- proach to automatic generation of non-standard sion, which can be used in many NLP and social language (Hickman, 2013), but for many practical media applications. More specifically, given a sen- purposes, it is also of crucial importance for ma- tence that includes a non-standard English expres- chines to be able to understand and explain this sion, we aim at automatically generating the trans- important subversion of the language. lation of the target expression. Previously, this In the NLP community, using dictionaries of is not possible because the resources of labeled non-standard language as an external knowledge 1For example, more than 2K entries are submitted daily to source is useful for many tasks. For example, Bur- Urban Dictionary (Kolt and Lazier, 2009), the largest online foot and Baldwin (2009) consult the slang defini- slang resource. non-standard expressions are not available. In this and McKeown, 2011). However, we argue that us- paper, we collect a large corpus of 15 years of ing a small, fixed-size dictionary approach may crowdsourced examples, and formulate the task as be suboptimal: it suffers from the low coverage a sequence-to-sequence generation problem. Us- problem, and to keep the dictionary up to date, ing a word-level model, we show that it is pos- maintaining such dictionary is also expensive and sible to build a general purpose non-standard En- time-consuming. To the best of our knowledge, glish words and phrases explainer using neural se- our work is the first to build a general purpose ma- quence learning techniques. To summarize our chine learning model for explaining non-standard contributions: English terms, using a large crowdsourced dataset. • We present a large, publicly available corpus 3 Our Approach of non-standard English words and phrases, including 15 years of definitions and exam- 3.1 Sequence-to-Sequence Model ples for each entry via crowdsourcing; Since our goal is to automatically generate expla- nations for any non-standard English expressions, • We present a hybrid word-character we select sequence-to-sequence models with at- sequence-to-sequence model that directly tention mechanisms as our fundamental frame- explains unseen non-standard expressions work (Bahdanau et al., 2014), which can pro- from social media; duce abstractive explanations, and assign different • Our novel dual encoder LSTM model outper- weights to different parts of a sentence. To model forms a standard attentive LSTM baseline, both the context words and the non-standard ex- and it is capable of generative plausible ex- pression, we propose a hybrid word-character dual planation for unseen non-standard words and encoder. An overview of our model is shown in phrases. Figure2. In the next section, we outline related work on 3.2 Context Encoder non-standard expressions and social media text Our context encoder is basically a recurrent processing. We will then introduce our dual en- neural network with long-short term memory coder based attentive sequence-to-sequence model (LSTM) (Hochreiter and Schmidhuber, 1997). for explaining non-standard expressions in Sec- LSTM consists of input gates, forget gates and tion3. Experimental results are shown in Sec- output gates, together with hidden units as its in- tion4. And finally, we conclude in Section5. ternal memory. Here, i controls the impact of new input, while f is a forget gate, and o is an output 2 Related Work gate. C~t is the candidate new value. h is the hidden The study of non-standard language is of inter- state, and m is the cell memory state. “ ” means ests to many researchers in the social media and element-wise vector product. The definition of the NLP communities. For example, Eisenstein et gates, memory, and hidden state is: al. (2010) propose a latent variable model to study i = σ(W [x ; h ]) the lexical variation of the language on Twitter, t i t t−1 where many regional slang words are discovered. ft = σ(W [xt; ht−1]) Zappavigna (2012) identifies the Internet slang as f an important component in the discourse of Twit- ot = σ(Wo[xt; ht−1]) ter and social media. Gouws et al. (2011) provide C~ = tanh(W [x ; h ]) a contextual analysis of how social media users t c t t−1 shorten their messages. Notably, a study on Tweet mt = mt−1 ft + it C~t normalization (Han and Baldwin, 2011) finds that, h = m o even when using a small slang dictionary of 5K t t t words, Slang makes up 12% of the ill-formed At each step, RNN is given a vector as input, words in a Twitter corpus of 449 posts. In the changes its hidden states and produces outputs NLP community, slang dictionary is widely used from its last layer. Hidden states and outputs are in many tasks and applications (Burfoot and Bald- stored and later passed to a decoder, which pro- win, 2009; Wang and McKeown, 2010; Rosenthal duces final outputs based on hidden states, outputs, it confuses the decoder on which one it should ex- plain. In practice, the user can often pin point to the exact expression she or he does not know, so in this work, we design a second encoder to learn the representation of the target non-standard expres- sion. Since our goal is to explain any non-standard English words and phrases, we consider a character-level encoder for this purpose. This sec- Figure 2: Dual Encoder Structure. Top left: a ond encoder reads the embedding vector of each word-level LSTM encoder for modeling context. character at each time step, and produces an out- Bottom left: a character-level LSTM encoder for put vector, and hidden states. Our dual encoder modeling target non-standard expression. Right: model linearly combines the hidden states of the an LSTM decoder for generating explanations. character-level target expression encoder with the hidden states of the word-level encoder with the and the decoder’s own parameters.The context en- following equation: coder learns and encodes sentence-level informa- tion for the decoder to generate explanations. hnew = h1W1 + h2W2 + B 3.3 Attention Mechanism Here, h1 is the context representation, and h2 is The idea of designing an attention mechanism is the target expression representation. The Bias to select key focuses in the sentence. More specif- B and the combination weights W1 and W2 are ically, we would like to pay attention to specific learnable. parts of encoder outputs and states. We follow a 4 Experiment recent study (Vinyals et al., 2015) to setup an at- tention mechanism. We have a separate LSTM for 4.1 Dataset decoding. We collect the non-standard English corpus2 from Here we briefly explain the method. When the Urban Dictionary (UD)3—the largest online slang decoder starts its decoding process, it has all hid- dictionary, where terms, definitions and examples den units from the encoder, denoted as (h ::h ). 1 T1 are submitted by the crowd. UD is made a reliable Also we denote the hidden state of the decoder resource, due to the quality control of its publish- as (d ::d ). T and T are input and output 1 T2 1 2 ing procedure.
Details
-
File Typepdf
-
Upload Time-
-
Content LanguagesEnglish
-
Upload UserAnonymous/Not logged-in
-
File Pages5 Page
-
File Size-