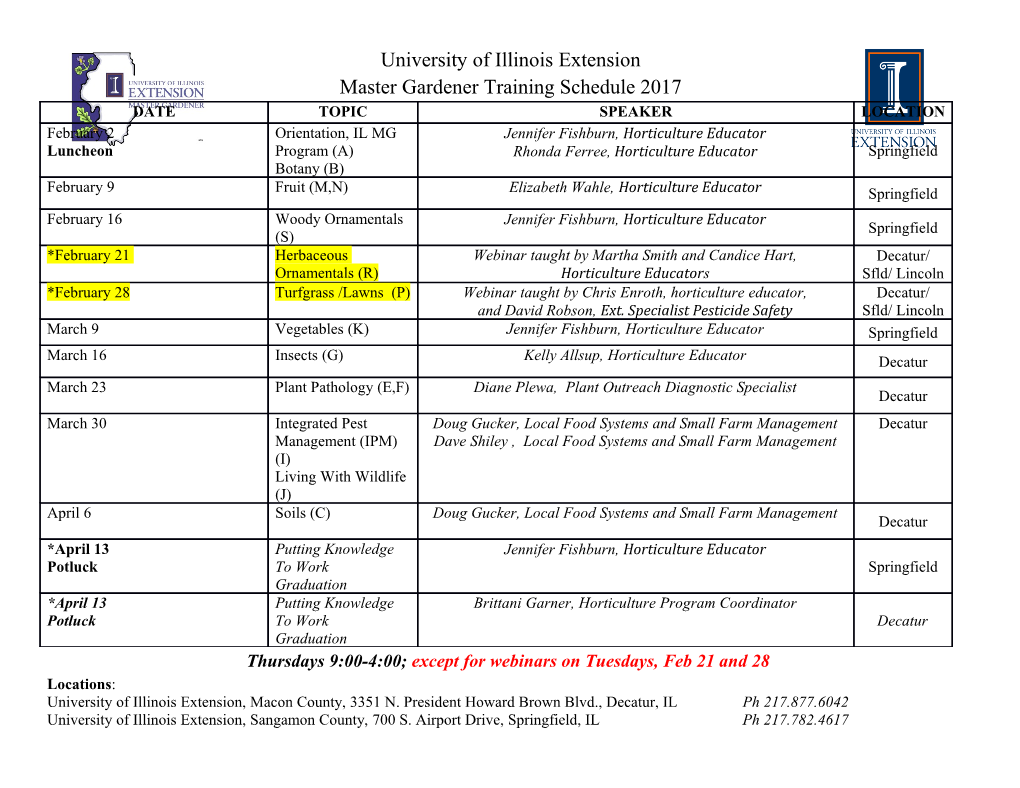
Petascaling Commodity onto Exascale with GPUs on TSUBAME1.2 onto TSUBAME2.0 Satoshi Matsuoka, Professor/Dr.Sci. Global Scientific Information and Computing Center (GSIC) Tokyo Inst. Technology 1 GPUs as Commodity Massively Parallel Vector Processors for Ultra Low Power • E.g., NVIDIA Tesla, AMD Firestream – High Peak Performance > 1TFlops • Good for tightly coupled code e.g. Nbody – High Memory bandwidth (>100GB/s) • Good for sparse codes e.g. CFD – Low latency over shared memory • Thousands threads hide latency w/zero overhead – Slow and Parallel and Efficient vector engines for HPC – Restrictions: Limited non-stream memory access, PCI-express overhead, programming model etc. How do we exploit them given vector computing experiences? GPUs as Commodity Vector Engines for Ultra Low Power Unlike the conventional accelerators, Accelerators GPUs have high memory bandwidth and N-body MatMul HPC dense &Ultra Low Power Since latest high-end GPUs support Density Computational Computational Cache-based double precision, GPUs also work CPUs as commodity vector processors. The target application area for GPUs is FFT very wide. Vector Processor Restrictions: Limited non- Memory stream memory access, Access PCI-express overhead, etc. Frequency How do we utilize them easily? From CPU Centric to GPU Centric Nodes for Scaling CPU “Centric” GDDR5 >150GB STREAM GPU persocket DDR3 4-8GB 15-20GB STREAM persocket Core 2GB/core Core Dataset Dataset CPU Roles These are - OS Isomorphic - Services NB PCIe x16 - Irregular PCIe x8 4GB/s Sparse 2GB/s PCIe x8 NB 2GB/s x n 40Gbps IB IB QDR GPU IB QDR HCA 1GB IB QDR HCA Flash 200GB HCA 400MB/s 40Gbps IB x n All-to-all 3-D Protein Docking Challenge (Collaboration with Yutaka Akiyama, Tokyo Tech.) P1 P2 P3 P4 P5 …. P1000 P1 P2 P3 1,000 x1,000 all-to-all docking P4 fitness evaluation will take only P5 1-2 months (15 deg. pitch) … with a 32-node HPC-GPGPU cluster (128 GPGPUs). Fast PPI candidate cf. screening with FFT ~ 500 years with single CPU (sus. 1+GF) P1000 ~ 2 years with 1-rack BlueGene/L Blue Protein system CBRC, AIST (4 rack, 8192 nodes) Algorithm for 3-D All-to-All Protein Docking candidate Protein 1 docking sites (1) 3-D (3) 3-D voxel (5) cluster data complex analysis Convolution NVIDIA (3-D FFT and its Inverse) CUDA Host Protein 2 CPUs (2) 3-D voxel (4) rotation data (6 deg., 15 deg.) Docking Confidence level Calculation for a single protein-protein pair: ~= 200 Tera ops. 3-D complex convolution O(N3 log N) , typically N = 256 x 200 Exa Ops for Possible rotations R = 54,000 (6 deg. pitch) 1000 x 1000 High Performance 3-D FFT on NVIDIA CUDA GPUs [Nukada et. al. SC08] Fast Fourier Transform is an O(NlogN) algorithm with O(N) memory access. Acceleration of FFT is much more difficult than N-body, MatMul, etc., but possible! At least, GPUs’ theoretical memory bandwidth is much higher than CPUs. multi-row FFT algorithm for GPU [SC08] Input This algorithm accesses 4 streams multiple streams, but each of them is successive. 4-point FFT Since each thread compute independent set of small FFT, Output many registers are required. 4 streams For 256-point FFT, use two- pass 16-point FFT kernels. Performance of 1-D FFT Note: An earlier sample with 1.3GHz is used for Tesla S1070. 3-D FFT Performance on various ComparisonCPUs, CellBE, with and CPUsGPUs 140 GFLOPS Overcoming the PCI-e BW Limitations-- -Keeping the Protein Data on the GPU Receptor Ligand Time (ms) 120 Rotate Device-to-Host 3-D FFT 80 Generate Grid Generate Grid Host-to-Device FWD 3D FFT MUL FWD 3D FFT 40 3D Convolution using 3D FFT INV 3D FFT 0 8800 GT 8800 GTS 8800 GTX Search Max Scores (Values) MAIN LOOP on GPGPU Card => pack as much card in node as possible Heavily GPU Acclerated Windows HPC Prototype Cluster • 32 compute nodes • 128 NVIDIA 8800GTS • one head node. • Gigabit Ethernet network • Three 40U rack cabinets. • Windows Compute Cluster Server 2008 • Visual Studio 2005 SP1 • nVidia CUDA 2.x Performance Estimation of 3D PPD Single Node Power (W) Peak (GFLOPS) 3D-FFT Docking Nodes per (GFLOPS) (GFLOPS) 40 U rack Blue Gene/L 20 5.6 - 1.8 1024 TSUBAME 1000 (est.) 76.8 (DP) 18.8 (DP) 26.7 (DP) 10 8800 GTS *4 570 1664 256 207 8~13 System Total ! Only CPUs for TSUBAME. DP=double precision. # of nodes Power Peak Docking MFLOPS/ (kW) (TFLOPS) (TFLOPS) W Blue Gene/L (Blue 4096 80 22.9 7.0 87.5 Protein@AIST,Japan) (4racks) TSUBAME. (Opteron 655 (~70 ~700 50.3 (DP) 17.5 (DP) 25 Only) racks) GPU Accel .WinHPC 32 (4racks) 18 53.2 6.5 361 Can compute 1000x1000 in 1 month (15 deg.) or 1 year (6 deg.) On full TSUBAME 1.2, ~100TFlops (1MW)-> ~52 rack BG/L TSUBAME 1.2 Experimental Evolution (Oct. 2008) The first “Petascale” SC in Japan Storage Voltaire ISR9288 Infiniband x8 1.5 Petabyte (Sun x4500 x 60) 10Gbps x2 ~1310+50 Ports NEC SX-8i 0.1Petabyte (NEC iStore) ~13.5Terabits/s 500GB Lustre FS, NFS, CIF, WebDAV (over IP) (3Tbits bisection) 48disks 60GB/s aggregate I/O BW 10Gbps+External NW 10,000 CPU Cores Sun x4600 (16 Opteron Cores) 32~128 GBytes/Node Unified Infiniband 300,000 SIMD Cores network 10480core/655Nodes > 20 Million Threads 21.4TeraBytes 50.4TeraFlops ~900TFlops-SFP, ~170TFlops-DFP OS Linux (SuSE 9, 10) NEW Deploy: 80TB/s Mem BW (1/2 ES) NAREGI Grid MW GCOE TSUBASA Harpertown-Xeon 90Node 720CPU 8.2TeraFlops PCI-e ClearSpeed CSX600 SIMD accelerator 648 boards, 52.2TeraFlops SFP/DFP 170 Nvidia Tesla 1070, ~680 Tesla cards High Performance in Many BW-Intensive Apps 10% power increase over TSUBAME 1.0 680 Unit Tesla Installation… While TSUBAME in Production Service (!) 15 TSUBAME 1.2 Node Configuration Thread Processor nVidia Tesla T10: 55nm, 470m2, Cluster (TPC) Thread Processor 1.4billion transistors ArrayThread (TPA or ProcessorSM) SP >1TF SFP 90GF DFP 240 SP Cores “Powerful Scalar” PCI-e x8 gen1 30 SMs GDDR3 4GB 2GB/s 1.4Ghz x86 102 GBytes/s 16 cores 1.08TFlops SFP Tesla Accelerator 2.4Ghz 16 90GFlops DFP 80GFlops 240 SP Cores 20GBytes/s 32GB 30 SMs GDDR3 4GB 1.4Ghz Dual Rail x4 IB SDR 102 GBytes/s 16 2 x 1GB/s 1.08TFlops SFP Tesla Accelerator 90GFlops DFP TSUBAME in Top500 Ranking Jun 06 Nov 06 Jun 07 Nov 07 Jun 08 Nov 08 Jun09 Rmax 38.18 47.38 48.88 56.43 67.70 77.48 87.01 (Tflops) [HPDC 2008] Rank 7 9 14 16 24 29 41 Opteron CS 360 CS 648 Xeon Tesla • Continuous improvement for 6 times • The 2nd fastest heterogeneous supercomputer in the world (No.1 is RoadRunner) 17 Linpack on TSUBAME---Heterogeneity at Work [Endo et.al. IPDPS08, etc.] • Linpack implementation that uses cooperatively: – 10,368 Opteron cores – 612 Tesla GPUs – 648 ClearSpeed accelerators – 640 Xeon cores (another cluster named “tsubasa”) • Different strategy than on Roadrunner is required • 87.01TFlops: The world’s highest Linpack performance on GPU-accelerated clusters + + Electric Power Consumption • About 1090kW during Linpack – An estimated value from sampling – Cooling, network are excluded 100% Even for dense 124.8 90% 16.2 problems GPUs 53.913 80% 30.28 30.4 70% are effective low 60% power vector- 50% 52.254 27.37 like engines 40% 920.16 30% 7.253 3.48 20% 49.766 25.87 Higher 10% 0% performance DP-peak (TF) Linpack (TF) power (kW) Total 163.2TF Total 87.0TF Total 1092kW with much lower Opteron Xeon ClearSpeed Tesla memory footprint GPU vs. Standard Many Cores? Shared Shared Shared Shared Shared Shared PE PE PE PE SP SP SP SP SP SP SP SP SP SP SP SP SP SP SP SP SP SP SP SP SP SP SP SP SP SP SP SP SP SP SP SP SP SP SP SP PE PE PE PE SP SP SP SP SP SP SP SP SP SP SP SP vs. PE PE PE PE MC MC MC MC MC MC PE PE PE PE GDDR3 GDDR3 GDDR3 GDDR3 GDDR3 GDDR3 • Consider strong scaling simple stencil computation e.g., SOR Multi-GPU in CFD: Riken Himeno Benchmark (Joint Work w/NEC) RIKEN Himeno CFD Benchmark Himeno for CUDA 16 64 16 Poisson Equation: 64+2 1 block = (Generalized coordinate) p 16x16x8 compute 2 p 2 p 2 p 2 p 2 p 2 p region x2 y2 z 2 xy xz yz Block has 256 thread Discretized Form: 128+2 Total 256 p 2 p p p 2 p p p 2 p p i 1, j,k i, j,k i 1, j,k i, j 1,k i, j,k i, j 1,k i, j,k 1 i, j,k i, j,k 1 blocks = x2 y 2 y 2 65536 pi 1, j 1,k pi 1, j 1,k pi 1, j 1,k pi 1, j 1,k threads 4 x y p p p p i 1, j,k 1 i 1, j,k 1 i 1, j 1,k i 1, j,k 1 16+2 4 x z Block 16+2 pi, j 1,k 1 pi, j 1,k 1 pi, j 1,k 1 pi, j 1,k 1 shared mem 4 y z i, j,k =16kB 8+2 18 neighbor point access Boundary region used21 for transfer Parallelization of Himeno Benchmark 513 Communication of border data is required Divide X axis for processes 1025 X Z 513 Y • Although original Himeno supports 3D division, our GPU version currently supports only 1D division Himeno Size L/L’ (257x257x513) CPU vs.
Details
-
File Typepdf
-
Upload Time-
-
Content LanguagesEnglish
-
Upload UserAnonymous/Not logged-in
-
File Pages45 Page
-
File Size-