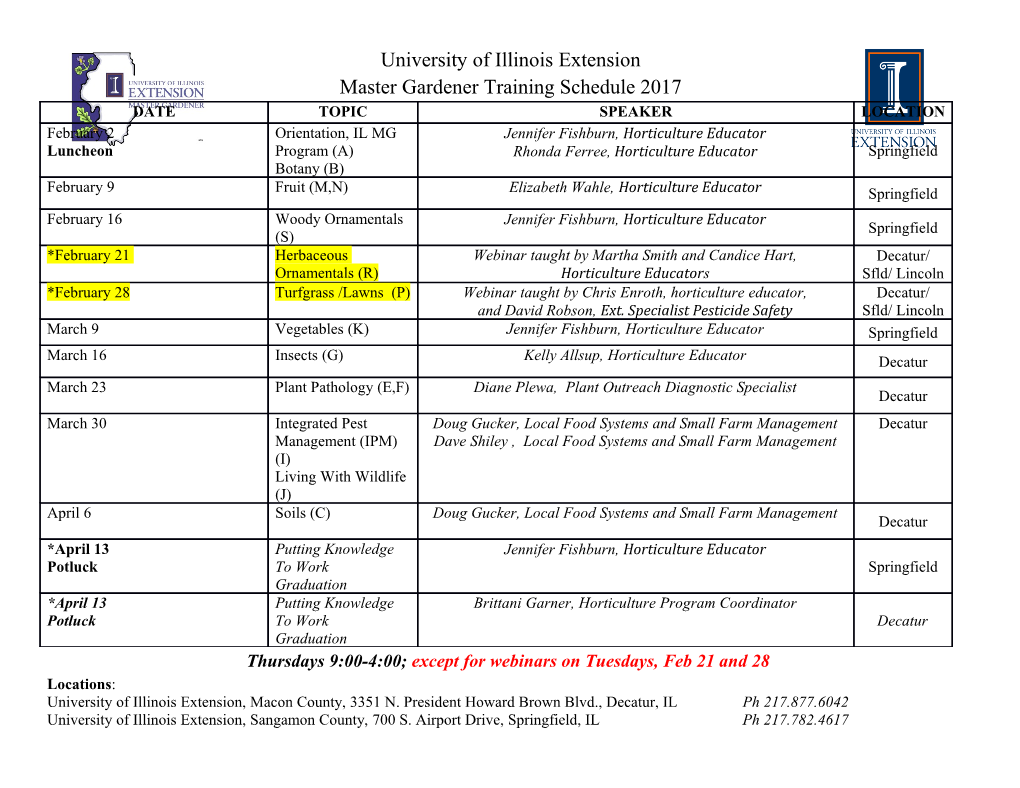
PERMANENTAL PARTITION MODELS AND MARKOVIAN GIBBS STRUCTURES HARRY CRANE Abstract. We study both time-invariant and time-varying Gibbs distributions for configura- tions of particles into disjoint clusters. Specifically, we introduce and give some fundamental properties for a class of partition models, called permanental partition models, whose distribu- tions are expressed in terms of the α-permanent of a similarity matrix parameter. We show that, in the time-invariant case, the permanental partition model is a refinement of the cele- brated Pitman-Ewens distribution; whereas, in the time-varying case, the permanental model refines the Ewens cut-and-paste Markov chains [J. Appl. Probab. (2011), 3:778–791]. By a special property of the α-permanent, the partition function can be computed exactly, allowing us to make several precise statements about this general model, including a characterization of exchangeable and consistent permanental models. 1. Introduction Consider a collection of n distinct particles (labeled i = 1;:::; n) located at distinct positions x1;:::; xn in some space . Each particle i = 1;:::; n occupies an internal state yi, which may change over time. Jointly,X the state of the system at time t 0 depends on the ≥ positions of the particles x1;:::; xn and the interactions among these particles. We assume that, with the exception of their positions in , particles have identical physical properties X and, thus, the pairwise interaction between particles at positions x and x0 is determined only by the pair (x; x0). In this setting, we model pairwise interactions by a symmetric function K : [0; 1] with unit diagonal, K(x; x) = 1 for all x , where K(x; x ) X × X ! 2 X 0 quantifies the interaction between particles at locations x and x0. Qualitatively, we regard K as the environment, or medium, equipped on . For a fixed configuration x ;:::; xn, we X 1 simply represent the interactions among x ;:::; xn by a matrix X := (Xij; 1 i; j n) for 1 ≤ ≤ which Xij := K(xi; xj). For example, the Ising model describes a system with internal states = 1 and interactions determined by the adjacency matrix of a weighted graph. More generally,Y {± g the above scheme describes an interacting particle system. In this paper, we consider interacting particle systems where the configuration is a partition of the particles 1;:::; n into disjoint clusters. In particular, we study a family of Gibbs measures on partitions of a finite set. Previously, Gibbs measures for fragmentation processes have appeared [4] in the context of modeling degradation of mass over time. More generally, models of agglomeration and fragmentation have been studied under the heading of coagulation and fragmentation processes, see e.g. [3, 5, 6, 33]. The exchangeable, or mean-field, case has garnered special attention; and, in our setting, the mean-field case Date: September 19, 2013. 1991 Mathematics Subject Classification. Primary 82C22, secondary 60C99, 60J10. Key words and phrases. Boltzmann-Gibbs measure; canonical Gibbs ensemble; Markovian Gibbs structure; permanental parition model; α-permanent; permanental process. This author is partially supported by NSF grant DMS-1308899 and NSA grant H98230-13-1-0299. 1 2 HARRY CRANE corresponds to an environment that is uniformly homogeneous, i.e. K(x; x0) 1 for all x; x0 ; see Aldous [1] for a review of mean-field models for stochastic coalescence.≡ 2 X Given an energy function H : R and a temperature β R, a Gibbs distribution on has the form Y! 2 Y ( ) 1 1 (1) Pβ,H Y = y = exp H(y) ; y : f g Zβ,H β 2 Y Boltzmann-Gibbs distributions arise in various settings in both statistical mechanics and elsewhere. In the special case where is the collection of partitions of [n]:= 1;:::; n , the energy function H can be assumedY to have the product partition form; that is,f for anyg partition π, H(π) can be expressed as a sum over the blocks of π. Product partition models were initially introduced by Hartigan [18] in the context of astronomical modeling and have since appeared in several applications involving partition models, including Bayesian nonparametrics. Because of its general form, many common distributions are of product partition type, including the Ewens distribution [14] from population genetics; however, the more general Pitman-Ewens distributions [33] are not strictly of product partition type. In this paper, we modify (1) so as to include both the Pitman-Ewens distributions and the more general permanental partition model, our principle model of interest. Extending (1) to a time-varying model, we define a Markovian Gibbs structure on as a transition probability of the form Y ( ) 1 1 (2) pβ,H(y; y0) = exp H(y; y0) ; y; y0 ; Zβ,H(y) β 2 Y where H : R is called the joint energy function and p H(y; y ) gives the probability Y × Y ! β, 0 of a transition from y to y0. In specializing to Markovian Gibbs structures on partitions, we restrict H to functions of (π, π ) that can be expressed as a sum over the blocks of π π , the 0 ^ 0 infimum of π and π0 in the partition lattice. We take up certain statistical and mathematical properties of permanental partition models. In particular, we consider conditions under which permanental models are ex- changeable, consistent and, in the time-varying case, reversible. Reversible models are important in many scientific contexts of temporally evolving physical systems [22]. Many physical phenomena are, at least intuitively, reversible, and so models for such phenomena should reflect this property. Consistency (under subsampling) is important in statistical inference, and reflects a coherence among a collection of probability distributions that per- mits out-of-sample statistical inference. Consistency also results in beautiful mathematical theory involving limiting stochastic processes as the size of the system grows to infinity; see e.g. [6] for an overview of the mathematical theory underlying consistent systems of partition-valued processes and see [27] for a discussion of consistency in statistical models. Consistency under subsampling belies an assumed lack of interference among particles in a physical system. As such, consistency may not be appropriate in some applications and, in keeping, the permanental model is consistent only under special choices of K. 1.1. The α-permanent of a matrix. Gibbs models for both time-invariant and time-varying partition structures have a history in diverse settings and here we focus primarily on perma- nental partition models. Specifically, we introduce both the permanental partition distribution and its related family of permanental partition transition probabilities, whose energy function entails the α-permanent of some similarity matrix. PERMANENTAL PARTITION MODELS AND MARKOVIAN GIBBS STRUCTURES 3 The permanent of any real-valued matrix X := (Xij; 1 i; j n) is defined by ≤ ≤ X Yn (3) per X := Xj,σ(j); σ n j=1 2S where n is the symmetric group acting on [n]:= 1;:::; n . In pure mathematics, the permanentS arises in representation theory of the symmetricf groupg as the immanant associ- ated to the trivial representation ρ 1 of n [16, 31]. In probability theory and statistical physics, permanents and determinants≡ appearS in spatial point process models of bosons and fermions, respectively. In contrast to Poisson point processes, permanental and de- terminantal processes incorporate dependence among the points in an aggregated point pattern with clumping (permanental) or repellence (determinantal). Relevant to fermions is the Pauli exclusion principle, by which no two particles can occupy the same quantum state, and, consistent with this principle, any matrix with two or more rows identical has zero determinant; this principle does not apply to bosons, for which the permanent is an appropriate kernel, see [11, 19, 29, 35]. In permanental point processes, X plays the role of a correlation matrix–a higher value of Xij indicates correlation between measurements on particles labeled i and j. In the permanental partition models we study, X plays the role of a similarity matrix–a higher value of Xij indicates that particles i and j are more likely to occupy the same internal state. In a different context, Gyires [17] studied a family of discrete probability distributions related to the permanent; and, more recently, a generalization of the permanent, called the α-permanent, has been connected to some well-known distributions in probability and statistics [37, 38]. For α R, the α-permanent of X is defined by 2 X Yn #σ (4) perα X := α Xj,σ(j); σ n j=1 2S where #σ denotes the number of cycles of σ n. Valiant [36] first showed the computa- tional complexity of the permanent is #P-complete;2 S and, since this seminal work, several algorithms have been proposed for its approximation, e.g. [21, 26, 28]. It has been recently conjectured [10] that, aside from α a negative integer, computation of the α-permanent is at least as complex as the permanent. 1.2. Permanental Gibbs models. While the permanent has received substantial attention in the literature, the α-permanent has been mostly neglected, except by a few authors. In this paper, we draw on ideas from statistical mechanics (Bolzmann-Gibbs distributions and permanental processes), applied probability (the Ewens sampling formula) and linear alge- bra (the α-permanent) to derive a natural family of distributions and transition probabilities on partitions of a finite set [n]. Throughout the paper, we generically assume that particles i = 1;:::; n occupy fixed positions in some abstract medium , and X := (Xij; 1 i; j n) X ≤ ≤ defines a similarity matrix so that Xij quantifies the similarity (or interaction) between particles i and j. Throughout the paper, we assume that X satisfies Xii = 1 for all 1 i n, • ≤ ≤ 0 Xij 1 for 1 i , j n, and • ≤ ≤ ≤ ≤ Xij = Xji for all 1 i; j n.
Details
-
File Typepdf
-
Upload Time-
-
Content LanguagesEnglish
-
Upload UserAnonymous/Not logged-in
-
File Pages26 Page
-
File Size-