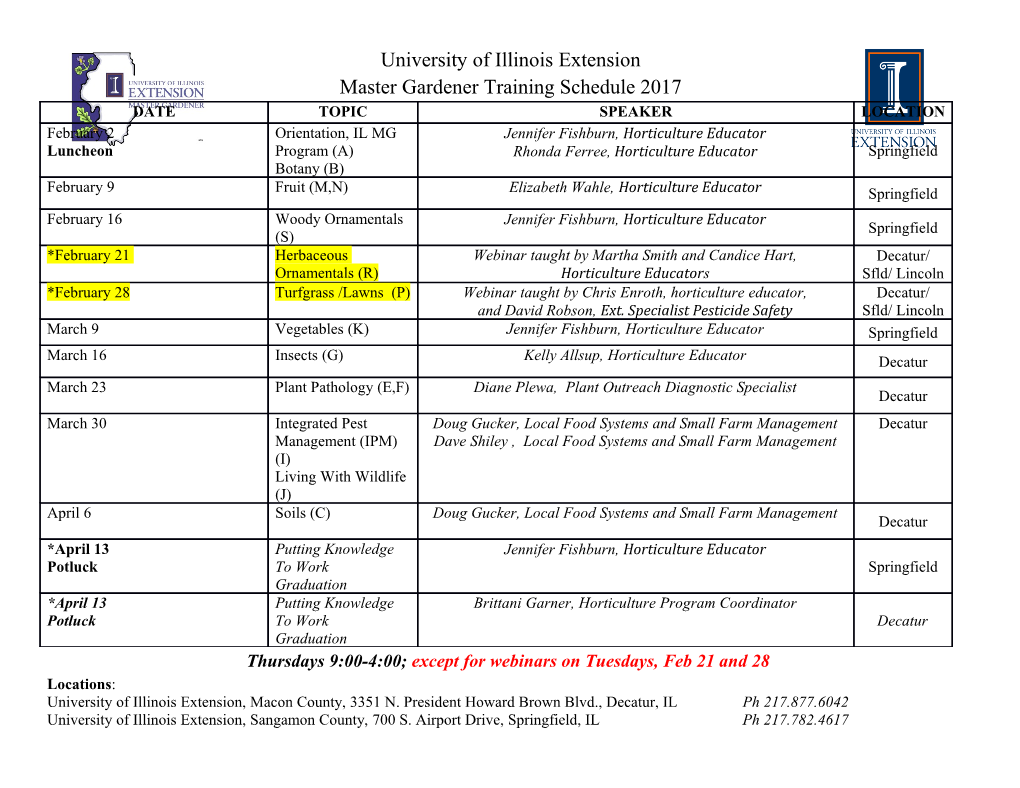
Decomposing the Dynamics of the Lorenz 1963 model using Unstable Periodic Orbits: Averages, Transitions, and Quasi-Invariant Sets Chiara Cecilia Maiocchi∗ and Valerio Lucarini† Centre for the Mathematics of Planet Earth, University of Reading and Department of Mathematics and Statistics, University of Reading Unstable periodic orbits (UPOs) are a valuable tool for studying chaotic dynamical systems. They allow one to extract information from a system and to distill its dynamical structure. We consider here the Lorenz 1963 model with the classic parameters’ value and decompose its dynamics in terms of UPOs. We investigate how a chaotic orbit can be approximated in terms of UPOs. At each instant, we rank the UPOs according to their proximity to the position of the orbit in the phase space. We study this process from two different perspectives. First, we find that, somewhat unexpectedly, longer period UPOs overwhelmingly provide the best local approximation to the trajectory, even if our UPO-detecting algorithm severely undersamples them. Second, we construct a finite-state Markov chain by studying the scattering of the forward trajectory between the neighbourhood of the various UPOs. Each UPO and its neighbourhood are taken as a possible state of the system. We then study the transitions between the different states. Through the analysis of the subdominant eigenvectors of the corresponding stochastic matrix we provide a different interpretation of the mixing processes occurring in the system by taking advantage of the concept of quasi-invariant sets. I. INTRODUCTION would suffice in obtaining an accurate approximation of ergodic averages [70–72]. These results are proven to be Unstable periodic orbits (UPOs) play an important valid for dynamical systems exhibiting strong chaoticity role in the analysis of dynamical systems that exhibit [73, 74], such as hyperbolic and Axiom A systems chaotic behaviour and in some cases they provide a [75, 76]. However, in complex models of fluid flows, it is powerful tool to describe the statistical properties of often difficult, if not impossible, to verify the hypothesis the system. As early noticed by Poincar´e, [63] unstable required for the validity of periodic orbit expansion. periodic orbits represent a rigid skeleton hidden in the When turbulent conditions are considered, such systems chaos of the dynamics and might be the key to the live, after transients have died out, in nonequilibrium understanding of chaotic systems [64]. Since then, UPOs steady state (NESS) [77, 78]. This state is in general have been at the centre of mathematical investigation. characterised by generation of entropy, contraction of Unstable periodic orbits are skeletal for the dynamics phase space and finite-time predictability. The ’Chaotic in the sense that they can be used to reconstruct the hypothesis’ of Gallavotti [79, 80] offers a possible solution statistical properties of a chaotic dynamical system [65]. to the first problem, allowing to consider ’a turbulent In fact, since UPOs are dense in the attractor [66], they fluid as a transitive Axiom A system for the purpose of can approximate any trajectory in the system with an computing macroscopic properties of the system.’ arbitrary accuracy [67]. UPOs can be considered as islets of order in a landscape of chaos. The trajectory moves from the neighbourhood of one UPO to another, undergoing a mechanism of repulsion because of their A. Unstable Periodic Orbits: Applications instability. UPOs provide a modal decomposition of the arXiv:2108.04181v2 [nlin.CD] 12 Aug 2021 dynamics, enabling to identify the dynamical properties A first application of periodic orbit expansion was of the trajectory with those of neighbouring UPOs. performed by Auerbach et al. in [81], by proving that Within this context it is possible to develop a theory UPOs are experimentally accessible and capable of that allows to write dynamical averages as weighted unfolding the structure of chaotic trajectories. In fact, sum over the full set of UPOs. Gutzweller [68] first by extracting the complete set of UPOs of order n demonstrated that UPOs are the essential building and calculating their stabilities, they approximated the blocks of chaotic dynamics. Ruelle later derived the fractal dimension and topological entropy of the strange dynamical ζ function [69] , that allows one to write attractor of the paradigmadic H´enon map with very the natural measure of the system as a weighted sum high accuracy. Cvitanovi´c[82] argued that UPOs are over the infinite set of UPOs. It is well known that a the optimal practical tool for measuring the invariant complete understanding of the full set of short UPOs properties of a dynamical system and provided a solid ground for the applications of periodic orbit theory (POT). Artuso et al. tested this procedure through a ∗ [email protected] series of applications [72, 83] and demonstrated that † [email protected] cycle expansion of the dynamical ζ function is instru- 2 mental for the analysis of deterministic chaos, even in B. This paper more generic settings than the ones required by [82], i.e. when the system is not uniformly hyperbolic nor Our research aims to give a contribution towards the the results depend on the assumption of the existence computational problems in the application of periodic of invariant measures or structural stability of the orbits theory. We believe that POT could indeed dynamics. Eckhardt and Ott [70] presented one of represent a very powerful tool allowing to gain insight the first numerical applications of the periodic orbit and simplify complex systems such as the climate, but formalism for studying the statistical and the dynamical its implementation is often hindered by the numerical properties of the Lorenz 1963 (L63) system [84]. A difficulties associated with UPOs search [93]. We subsequent analysis of the linear and nonlinear response consider the L63 model as a test case. The use of UPOs of the L63 to perturbations found that specific UPOs for averaging in L63 has been hugely debated in the were responsible for resonance mechanisms leading to an literature (See discussion in section II A 2 ). We will amplified response [85]. not delve into this matter, but we rather want to shed light on the shadowing mechanism and investigate its underpinning dynamics. In order to avoid confusion, Later on, periodic orbit theory found fruitful applica- since the term ”shadowing” can be used with the tions also within the context of higher dimension forced meaning given in [67], we specify that by ”shadowing” and dissipative non-equilibrium systems, specifically in we refer to the process of approximation of the chaotic the case of (geophysical) fluid dynamics. UPOs can be trajectory in terms of UPOs. In particular, at each considered as a mean to simplify and interpret qualitative point in time we rank UPOs based on their distance behaviour of a complex system [86], allowing to extract with respect to the given chaotic trajectory. We name information and distill its dynamical structure. This ob- this process ranked shadowing. Within this context, servation, together with the study of the stability and our approach is twofold. On the one side we aim at thus predictability properties of the tangent space, al- numerically understand how chaotic trajectories are lows to associate relevant dynamical features of the flow approximated in terms of UPOs. On the other side, we to specific UPOs or classes of UPOs. UPOs, true nonlin- study transitions between neighbourhood of UPOs. ear modes of flow, can be interpreted as a generalisation of the normal modes observed in a network of coupled linear oscillators, that allow for a study of the system In the first part of our work we analyse the shadowing in its complexity, without the necessity of considering a process by taking a local point of view and studying heavily truncated model. the shadowing statistics of a chaotic trajectory. Namely, we numerically implement the shadowing process, fo- Even though a periodic-orbit-theory-type expansion cusing on the point-wise dynamics along the trajectory, for turbulence is still a far reaching goal, many steps by locating the closest UPOs to each point, ordered have been made in this direction. The path towards the accordingly to their distance from the chaotic trajec- description of a turbulent flow in terms of UPOs is paved tory. Extending periodic search to higher dimensional with promising results [87]. Kawahara and Kida [88], systems still represents an open challenge [89] and who found a UPO embedded in the attractor of a nu- standard periodic averaging with an incomplete set of merical simulation of plane Couette flow, showed that one UPOs does not provide accurate results. A possible UPO only manages to capture in a surprisingly accurate strategy to overcome the aforementioned difficulties way the turbulence statistics. Chandler and Kerswell [89] has been proposed by Lasagna [94, 95], who found identified 50 UPOs of a turbulent fluid and used them to numerical evidence that long period orbits could be used reproduce the energy and dissipation probability density as proxies of chaotic trajectories. Following the idea functions of the system as dynamical averages over the that long UPOs might be able to capture statistical orbit. These encouraging results suggested that periodic properties of chaotic trajectories, periodic search could orbit theory could represent a valid investigation tool also be limited to few long UPOs, notably reducing the in the realm of climate systems. Lucarini and Gristun computational complexity of the problem. In line with [86] considered POT as a novel mathematical framework this work, we find that longer UPOs have the lion’s for investigating atmospheric, ocean and climate dynam- share in reproducing the invariant measure of the system. ics. Gritsun [90] numerically applied the theory of UPOs expansion [73, 91] to reconstruct the probability distri- In the second part of this paper, inspired by the work bution function of a simple atmospheric model based on of Froyland and Padberg on quasi-invariant sets [96, 97], the barotropic vorticity equation of the sphere.
Details
-
File Typepdf
-
Upload Time-
-
Content LanguagesEnglish
-
Upload UserAnonymous/Not logged-in
-
File Pages14 Page
-
File Size-