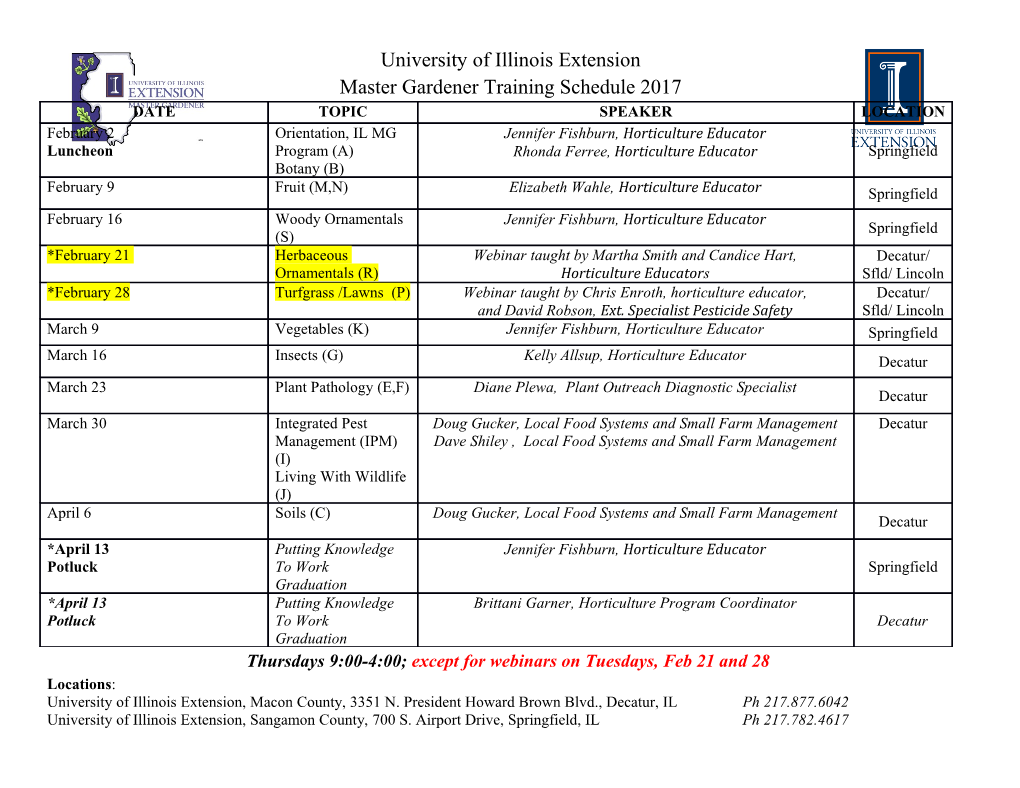
Self-Adjusting Computation with Delta ML Umut A. Acar1 and Ruy Ley-Wild2 1 Toyota Technological Institute Chicago, IL, USA [email protected] 2 Carnegie Mellon University Pittsburgh, PA, USA [email protected] Abstract. In self-adjusting computation, programs respond automati- cally and efficiently to modifications to their data by tracking the dy- namic data dependences of the computation and incrementally updating the output as needed. In this tutorial, we describe the self-adjusting- computation model and present the language ∆ML (Delta ML) for writ- ing self-adjusting programs. 1 Introduction Since the early years of computer science, researchers realized that many uses of computer applications are incremental by nature. We start an application with some initial input to obtain some initial output. We then observe the output, make some small modifications to the input and re-compute the output. We often repeat this process of modifying the input incrementally and re-computing the output. In many applications, incremental modifications to the input cause only incremental modifications to the output, raising the question of whether it would be possible to update the output faster than recomputing from scratch. Examples of this phenomena abound. For example, applications that interact with or model the physical world (e.g., robots, traffic control systems, schedul- ing systems) observe the world evolve slowly over time and must respond to those changes efficiently. Similarly in applications that interact with the user, application-data changes incrementally over time as a result of user commands. For example, in software development, the compiler is invoked repeatedly after the user makes small changes to the program code. Other example application areas include databases, scientific computing (e.g., physical simulations), graph- ics, etc. In many of the aforementioned examples, modifications to the computation data or input are external (e.g., the user modifies some data). In others, incre- mental modifications are inherent. For example, in motion simulation, objects move continuously over time causing the property being computed to change continuously as well. In particular, if we wish to simulate the flow of a fluid by modeling its constituent particles, then we need to compute certain properties of moving objects, e.g., we may want to triangulate the particles to compute the 2 forces exerted between particles, and update those properties as the points move. Since the combinatorial structure of the computed properties change slowly over time, we can often view continuous motion as an incremental modification; this makes it possible to compute the output more efficiently than re-computing it from-scratch at fixed intervals. Although incremental applications abound, no effective general-purpose tech- nique or language was known for developing incremental applications until re- cently (see Section 10 for the discussion of the earlier work on the subject). Many problems required designing specific techniques or data structures for remem- bering and re-using results to ensure that computed properties may be updated efficiently under incremental modifications to data. Recent advances on self- adjusting computation (Section 10.2) offer an alternative by proposing general- purpose techniques for automatically adapting computations to data modifica- tions by selectively re-executing the parts of the computation that depend on the modifications and re-using unaffected parts. Applications of the technique to problems from a reasonably diverse set of areas show that the approach can be effective both in theory and practice. We present a tutorial on a language for self-adjusting computation, called ∆ML (Delta ML), that extends the Standard ML (SML) language with primi- tives for self-adjusting computation. In self-adjusting computation, programs consist of two components: a self- adjusting core and a top- or meta-level mutator. The self-adjusting core is a purely functional program that performs a single run of the intended applica- tion. The mutator drives the self-adjusting core by supplying the initial input and by subsequently modifying data based on the application. The mutator can modify the computation data in a variety of forms depending on the application. For example, in a physical simulation, the mutator can insert a new object into the set of objects being considered. In motion simulation, the mutator changes the outcomes of comparisons performed between objects as the relationship be- tween objects change because of motion. After modifying computation data, the mutator can update the output and the computation by requesting change propagation to be performed. Change propagation is at the core of self-adjusting computation: it an automatic mechanism for propagating the data modifications through the computation to update the output. To support efficient change propagation, we represent a computation with a trace that records the data and control dependences in the computation. Change propagation uses the trace to identify and re-execute the parts of the compu- tation that depend on the modified data while re-using the parts unaffected by the changes. The structure and the representation of the trace is critical to the effectiveness of the change propagation. Techniques have been developed for implementing both tracing and change propagation efficiently (Section 10.2). The ∆ML language provides linguistic facilities for writing self-adjusting pro- grams consisting of a core and a mutator. To this end, the language distinguishes between two kinds of function spaces: conventional and self-adjusting. The mu- tator consists solely of conventional functions. The self-adjusting core consists 3 of self-adjusting functions and all pure (self-adjusting or conventional) functions that they call directly or indirectly (transitively). ∆ML enables the programmer to mark the computation data that is expected to change across runs (or over time) by placing them into modifiable references or modifiables for short. For implementing a self-adjusting core, ∆ML provides facilities for creating and reading modifiables within a self-adjusting function. In this tutorial, we do not include the update operation on modifiables in the core| modifiables are write-once within the self-adjusting core. 3 ∆ML also provides facilities for defining self-adjusting functions to be memoized if so desired. For implementing a mutator, ∆ML provides meta-level facilities to create, read, and update modifiables, and to perform change propagation. The mutator can use the update operation to modify destructively the contents of modifiables—this is how mutators modify the inputs of the self-adjusting core. After such modifications are performed, the mutator can use change propagation to update the result of the core. Writing a self-adjusting program is very similar to writing a conventional, purely functional program. Using the techniques described in this tutorial, it is not hard to take an existing purely functional SML program and make it self-adjusting by annotating the code with ∆ML primitives. Annotated code is guaranteed to respond to modifications to its data correctly: the result of an updated run is equivalent to a (from-scratch) run. Guaranteeing efficient change propagation, however, may require some additional effort: we sometimes need to modify the algorithm or use a different algorithm to achieve the optimal update times. When an algorithm does not yield to efficient change propagation, it is some- times possible to change it slightly to regain efficiency, often by eliminating un- necessary dependences between computation data and control. For example, the effectiveness of a self-adjusting mergesort algorithm can be improved by employ- ing a divide-and-conquer strategy that divides the input into two sublists ran- domly instead of deterministically dividing in the middle. Using randomization eliminates the dependence between the length of the list and the computation, making the computation less sensitive to modiciations to the input (e.g. when a new element is inserted the input length changes, causing the divide-and-conquer algorithm to create different splits than before the insertion, ultimately prevent- ing re-use). Sometimes, such small changes to the algorithm do not suffice to improve its efficiency and we need to consider an entirely different algorithm. For example, the quicksort algorithm is inherently more sensitive to input mod- ifications than the mergesort algorithm, because it is sensitive to values of the pivots, whereas the mergesort algorithm is not. Similarly, an algorithm that sums a list of numbers by performing a traversal of the list and maintaining an accumulator will not yield to efficient change propagation, because inserting an element can change the value of the accumulator at every recursive call. No 3 The actual ∆ML language places no such restrictions on how many time modifiables can be written in the core. 4 small modification will improve this algorithm as we would like. Considering a different, random sampling algorithm addresses the problem (Section 8). The structure of the rest of the tutorial is as follows. In Section 2 we de- scribe how incremental modifications arise and why they can lead to improved efficiency and why having general-purpose techniques and languages can help take advantage of this potential. In Section 3 we describe the self-adjusting com- putation model and the core and the meta primitives for writing self-adjusting programs. In Section 4 we describe an example self-adjusting application, called CIRCLES, and how the user can interact with such a program. In the rest of the tutorial, we use this example to illustrate how the ∆ML language may be used to implement self-adjusting programs. 2 Motivation We consider the two kinds of modifications, discrete and continuous, that arise in incremental applications via simple examples and describe how we may take ad- vantage of them to improve efficiency. We then describe how and why language- based techniques are critical for scalability. 2.1 Discrete and Continuous Modifications Close inspection of incremental applications reveal that two kinds of modification arise naturally: discrete/dynamic and continuous/kinetic.
Details
-
File Typepdf
-
Upload Time-
-
Content LanguagesEnglish
-
Upload UserAnonymous/Not logged-in
-
File Pages38 Page
-
File Size-