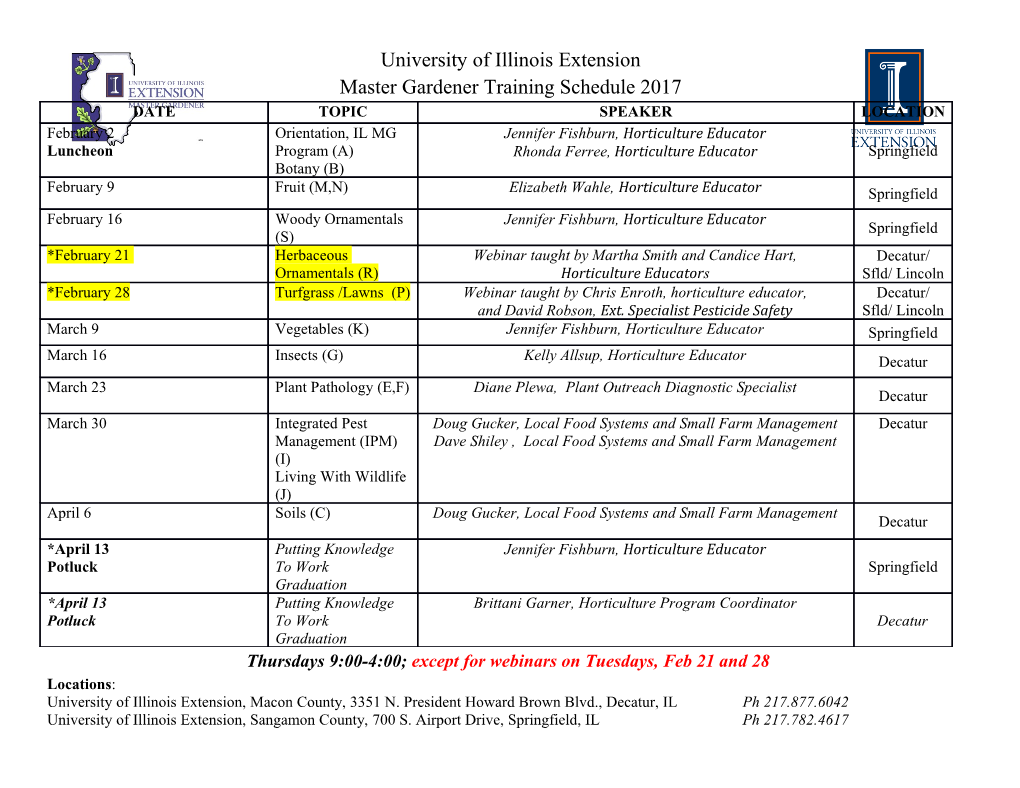
A Software Platform for Manipulating the Camera Imaging Pipeline Hakki Can Karaimer Michael S. Brown Department of Electrical Engineering and Computer Science Lassonde School of Engineering, York University, Canada [email protected], [email protected] Abstract. There are a number of processing steps applied onboard a digital camera that collectively make up the camera imaging pipeline. Unfortunately, the imaging pipeline is typically embedded in a camera's hardware making it difficult for researchers working on individual com- ponents to do so within the proper context of the full pipeline. This not only hinders research, it makes evaluating the effects from modifying an individual pipeline component on the final camera output challenging, if not impossible. This paper presents a new software platform that allows easy access to each stage of the camera imaging pipeline. The platform allows modification of the parameters for individual components as well as the ability to access and manipulate the intermediate images as they pass through different stages. We detail our platform design and demon- strate its usefulness on a number of examples. Keywords: camera processing pipeline, computational photography, color processing 1 Introduction Digital cameras are the cornerstone for virtually all computer vision applica- tions as they provide the image input to our algorithms. While camera images are often modeled as simple light-measuring devices that directly convert in- coming radiance to numerical values, the reality is that there are a number of processing routines onboard digital cameras that are applied to obtain the final RGB output. These processing steps are generally performed in sequence and collectively make up the camera imaging pipeline. Examples of these processing steps include Bayer pattern demosiacing, white-balance, color space mapping, noise reduction, tone-mapping and color manipulation. Many of these processing steps are well-known research topics in their own right, e.g. white-balance, color space mapping (colorimetry), and noise reduction. Although cameras are the most prominent hardware tools in computer vision, it is surprisingly difficult to get access to the underlying imaging pipeline. This is because these routines are embedded in the camera's hardware and may involve proprietary image manipulation that is unique to individual camera manufac- turers. This is a significant drawback to the research community. In particular, it 2 Hakki Can Karaimer Michael S. Brown Display / RAW Pre- White Post Color Color Demosaic Compress Image processing Balance processing Transform Rendering and Store Black Level Offset, Noise reduction, Tone-mapping, Normalization, Sharpening sRGB gamma, Bad Pixel Mask Color manipulation Fig. 1. This figure (adapted from [22]) overviews the common steps applied onboard a camera. Different camera hardware implementations can vary, however, most of these components will be included and in a similar processing order. forces many researchers to work on topics outside the proper context of the full imaging pipeline. For example, much of the work targeting white-balance and color constancy is performed directly on the camera-specific raw images without the ability to demonstrate how it would affect the final output on the camera. Another example includes noise reduction (NR) targeting sensor noise. On a camera, NR is applied before many of the non-linear photo-finishing routines (e.g. tone-curve manipulation), however, researchers are generally forced to ap- ply NR on the final non-linear sRGB image due to a lack of access to the camera pipeline. This presents a significant mismatch between assumptions made in the academic literature and real industry practice. Contribution We present a software platform to allow easy access to each stage of the imaging pipeline. Our approach operates on images saved in DNG raw image format which represents the unprocessed sensor response from the camera and the starting point for the camera processing pipeline. Our platform allows images to be opened and run through a software rendering API that parallels the onboard processing steps, including the individual processing components and their associated parameters. More specifically, our platform provides API calls that allow the modification of processing components' parameters and full access to the intermediate images at each processing stage. Such intermediate images can be modified and inserted back into the pipeline to see the effect on the final output. The proposed software platform is easily integrable with other softwares such as Matlab and provides a much needed environment for improving camera imaging, or performing experiments within the proper context of the full camera imaging pipeline. The remainder of this paper is organized as follows: Section 2 discusses related work; Section 3 overviews our platform and the ways the pipeline can be accessed and manipulated; Section 4 provides a number of examples that use the platform on various routines. The paper is concluded with a short discussion and summary in Section 5. 2 Related Work The basic steps comprising the camera processing pipeline are illustrated in Fig- ure 1 and may vary among different cameras' make and model. Full details to each component are outside the scope of this paper and readers are referred to A Software Platform for Manipulating the Camera Imaging Pipeline 3 [22] for an excellent overview. As mentioned in Section 1, many of the com- ponents in the pipeline (e.g. white-balance, noise reduction) are stand alone research topics in the computer vision and image processing community. Unfor- tunately, the hardware implementation of the camera pipeline and closed nature of proprietary cameras makes it difficult for most researchers to directly access the individual components. To address this issue, open hardware platforms have been proposed. Early work included the CMU-camera [23] targeting robotic vision. While individual components (e.g. white-blanance) were not accessible, the camera provided low- level image access and subsequent hardware releases allowed capture of the raw sensor response. A more recent and comprehensive hardware platform was the FrakenCamera introduced by Adams et al. [1]. The FrankenCamera was designed as a fully operational camera that provided full access to the underlying hard- ware components, including control of the flash and shutter, as well as the un- derlying imaging pipeline. The FrankenCamera platform targeted computational photography applications, however, the platform was suitable for modifying in- dividual components in the imaging pipeline. While the FrankenCamera project has officially stopped, much of the platform's functionality has been incorpo- rated into the recent Android's Camera2 API [15] that is available on devices running Android OS. The proposed work in this paper is heavily inspired by the FrankenCamera open design and aims to provide similar functionality via a software-based platform. The benefits of a software framework over a hardware solution is that it can work on images saved from a variety of different cameras and in an off-line manner. Moreover, a software platform allows greater flexibility in processing the image at intermediate stages than possible on fixed hardware implementations. There have been a number of works that have targeted modeling the camera processing pipeline beyond simple tone-curves for radiometric calibration (e.g. [10, 20, 19, 18, 30]). These methods use input pairs of raw and sRGB images to derive a mapping to convert an sRGB image back to the raw linearized sensor response. In some cases, this mapping closely follows the underlying imaging pipeline [10, 20, 18, 30], however, in other cases the mapping is approximated by a 3D look up table [19]. Another noteworthy example is the work by Baek et al. [4] that incorporated a fast approximation of the camera pipeline for displaying raw images in the camera's view finder. While this work focused on translating sparse user interaction applied on the view finder to control the final photo-finished image, it elucidated the need to incorporate the non-linear processing steps to give a more realistic representation of the final output to the user. While these methods are useful for simulating the onboard camera imaging process, they only provide a proxy for a real camera pipeline. The benefits of considering the full camera pipeline in various computer vision and image processing tasks have been demonstrated in prior works. For example, Bianco et al. [6, 7] showed that the overall color rendition of an image can be improved by considering white-balancing and color space conversion together in the pipeline. Work by Tai et al. [25] showed that the non-linear mapping 4 Hakki Can Karaimer Michael S. Brown (A) Stages of the camera imaging pipeline and associated parameters # # # # # # # # # # # # f f Gamma applied for visualization 6- White-balancing & 2- Black light subtraction, 3- Lens correction 4- Demosaicing 5- Noise reduction Color space [MATs] 1- Reading raw Image Linearization [2D Array(s)] [Func] [Func] [Values or 1D LUT] # # # # # # # # # 12- Gamma curve 11- Final color space 10- Tone curve 9- Color mani- 8- Exposure curve 7- Hue/Sat map application [1D LUT] conversion [Mat] application [1D LUT] pulation [3D LUT] [EV value or 1D LUT] [3D LUT] (B) Intermediate images for each stage 1 2 3 4 5 6 12 11 10 9 8 7 Fig. 2. The camera processing pipeline routines accessible by our software platform are shown in (A). Each component is denoted by the type of parameters it takes, e.g. scalar values, 2D Arrays, 3 × 3 matrices [MAT], function calls [func], or 1D or 3D Look up tables [LUT]. In addition, the software platform API supports direct access and manipulation to the intermediate images at each stage as shown in (B). applied onboard cameras had a significant impact on image deblurring results. Recent works by by Nam et al. [21] and Wong and Milanfar [27] demonstrated improvements in image denoising when the non-linear processing steps in the camera pipeline are considered in the noise model.
Details
-
File Typepdf
-
Upload Time-
-
Content LanguagesEnglish
-
Upload UserAnonymous/Not logged-in
-
File Pages16 Page
-
File Size-