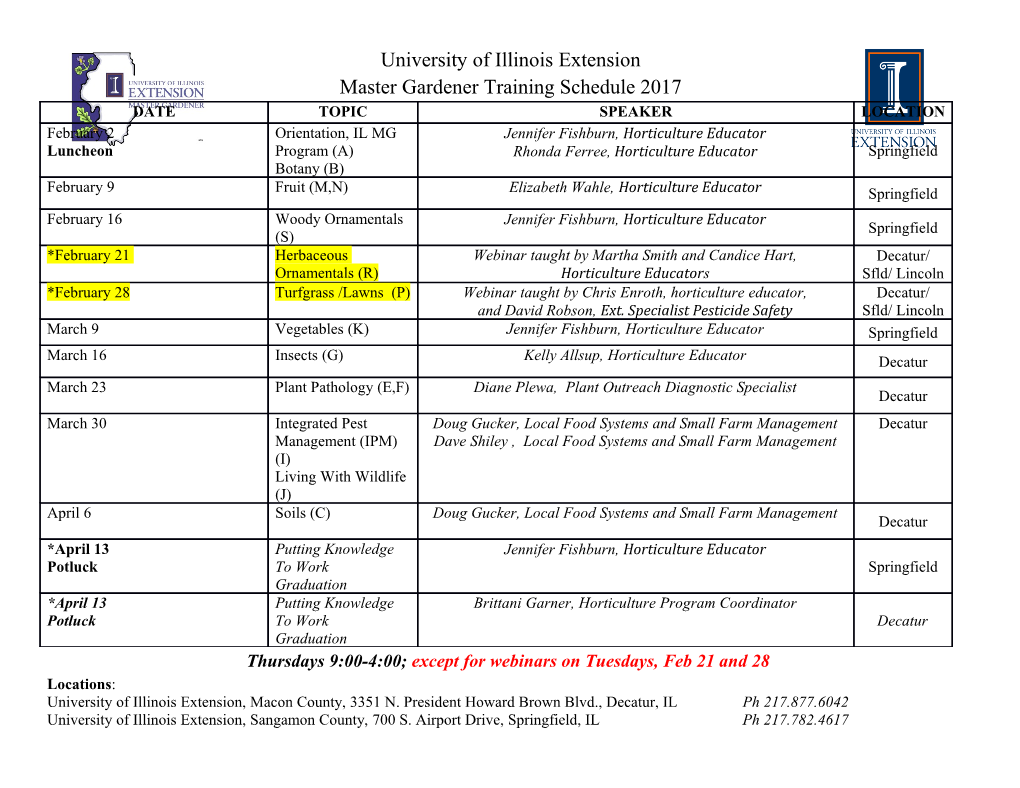
PREDICTING INFORMATIVE SPATIO-TEMPORAL NEURODEVELOPMENTAL WINDOWS AND GENE RISK FOR AUTISM SPECTRUM DISORDER. a thesis submitted to the graduate school of engineering and science of bilkent university in partial fulfillment of the requirements for the degree of master of science in computer engineering By O˘guzhanKarakahya October 2020 Predicting informative spatio-temporal neurodevelopmental windows and gene risk for autism spectrum disorder. By O˘guzhanKarakahya October 2020 We certify that we have read this thesis and that in our opinion it is fully adequate, in scope and in quality, as a thesis for the degree of Master of Science. A. Erc¨ument C¸i¸cek(Advisor) Can Alkan Tunca Do˘gan Approved for the Graduate School of Engineering and Science: Ezhan Kara¸san Director of the Graduate School ii ABSTRACT PREDICTING INFORMATIVE SPATIO-TEMPORAL NEURODEVELOPMENTAL WINDOWS AND GENE RISK FOR AUTISM SPECTRUM DISORDER. O˘guzhanKarakahya M.S. in Computer Engineering Advisor: A. Erc¨ument C¸i¸cek October 2020 Autism Spectrum Disorder (ASD) is a complex neurodevelopmental disorder with a strong genetic basis. Due to its intricate nature, only a fraction of the risk genes were identified despite the effort spent on large-scale sequencing studies. To perceive underlying mechanisms of ASD and predict new risk genes, a deep learning architecture is designed which processes mutational burden of genes and gene co-expression networks using graph convolutional networks. In addition, a mixture of experts model is employed to detect specific neurodevelopmental periods that are of particular importance for the etiology of the disorder. This end-to-end trainable model produces a posterior ASD risk probability for each gene and learns the importance of each network for this prediction. The results of our approach show that the ASD gene risk prediction power is improved compared to the state-of-the-art models. We identify mediodorsal nucleus of thalamus and cerebellum brain region and neonatal & early infancy to middle & late childhood period (0 month - 12 years) as the most informative neurodevelopmental window for prediction. Top predicted risk genes are found to be highly enriched in ASD- associated pathways and transcription factor targets. We pinpoint several new candidate risk genes in CNV regions associated with ASD. We also investigate confident false-positives and false negatives of the method and point to studies which support the predictions of our method. Keywords: Autism Spectrum Disorder, Graph Convolutional Networks, Deep Learning. iii OZET¨ OTIZM_ SPEKTRUM BOZUKLUGU˘ IC¸_ IN_ BILG_ I_ VERIC_ I_ ZAMAN-UZAMSAL SIN_ IR_ GELIS¸_ IM_ ARALIGI˘ VE GEN RISK_ I_ TAHMIN_ I_ O˘guzhanKarakahya Bilgisayar M¨uhendisli˘gi,Y¨uksekLisans Tez Danı¸smanı:A. Erc¨ument C¸i¸cek Ekim 2020 Otizm Spektrum Bozuklu˘gu(OSB), genetik sebeplerle ortaya ¸cıkabilen, zihin- sel geli¸simi olumsuz etkileyen karma¸sık bir hastalıktır. Karma¸sık do˘gasından dolayı, bu hastalı˘gasebep olan risk genlerinin sadece k¨u¸c¨ukbir y¨uzdesi,gen dizileme ¸calı¸smalarısayesinde tespit edilebilmi¸stir. Bu hastalı˘gasebep olan et- menleri anlamak i¸cin,mutasyon y¨uk¨uverisini gen ortak ifade ¸cizgeleri¨uzerinde kullanabilen bir derin ¨o˘grenmemimarisi tasarlandı. Ek olarak, hastalık i¸cin¨onem arz eden sinirsel geli¸simperiyotlarını tespit edebilmek i¸cinderin ¨o˘grenmemod- eline uzmanların karı¸sımımodeli de eklendi. Bu u¸ctanuca e˘gitilebilensistem ¸cizgeba¸sınabir a˘gırlık¨o˘grenerekb¨ut¨ungenler i¸cinbir olasılık atayabilmekte- dir. Modelimizin sonu¸cları,otizm geni risk tahmin g¨uc¨un¨unen geli¸smi¸smod- ellere kıyasla arttı˘gınıg¨ostermektedir. En y¨uksekrisk penceresi olarak talamus ve serebellum beyin b¨olgesininmediyodorsal ¸cekirde˘ginive yenido˘gan/erken be- beklikten orta/ge¸c¸cocukluk d¨onemine kadar olan periyot (0 ay - 12 ya¸s)belir- lenmi¸stir. Sonu¸clarımız, otizm ile alakalı bilinen anahtar biyolojik yollar ve gen hedefleri i¸cin iyi bir zenginle¸smeye de i¸saretetmektedir. OSB ile ili¸skilibir etiketi olmayan kopya sayısı de˘gi¸sikli˘gib¨olgelerinderisk geni olmaya aday birka¸cgen g¨ozlemlenmi¸stir. Yalancı-pozitif kesin referans genler, etiketlenmemi¸solmasına ra˘gmenOSB ile ili¸skiliolma olasılı˘gıy¨uksekgenler ve y¨ukseksıralamalı yalancı- negatif kesin referans genler incelenmi¸stir. Anahtar s¨ozc¨ukler: Otizm Spektrum Bozuklu˘gu,C¸izge Evri¸simselA˘glar,Derin O˘grenme.¨ iv Acknowledgement First of all, I would like to thank my advisor Asst. Prof. Erc¨ument C¸i¸cekfor his understanding and assistance throughout my study. It wouldn't be possible for me to neither conduct this study, nor becoming a researcher without his guidance and patience. Therefore, i am very grateful for his continuous support. I am also thankful to my jury members X and Y for reading my thesis and for accepting being in my thesis committee. I thank to Simons Foundation Autism Research Initiative for funding this research via the SFARI 640935 pilot grant awarded to Erc¨ument C¸i¸cek. I would like to thank Ilayda_ Beyreli for her support on this work. She helped me to design the architecture and implement the source code for this study. She also shared her invaluable feedbacks with me during the whole process. I feel very lucky for being in the community of Bilkent University for 7 years. During my studies, I earned invaluable friendships and collected wonderful mem- ories. I would like to thank Do˘gukan, Arda, Yusuf, Ozan and Muammer for their friendship for our good memories. I am also very grateful for all the good time we had with Ayberk, Sezernaz and Ya˘gmur, and for their precious friendship. I also would like to thank my colleague and friend Ilayda_ for all of her support, feedbacks and efforts. She has a great amount of contribution to this thesis. I also thank Mustafa for his friendship and introducing me to the life-long hobby of board games. Finally, I am endlessly thankful for all the efforts of my parents. They were always on my side, supporting me all the time. Without their love, support and belief, I wouldn't be where I am now in the first place. I cannot pay back for all of their efforts whatever I do in return. I will be doing my best to make them proud. v Contents 1 Introduction 1 2 Background Information 4 2.1 De Novo Gene Disrupting Mutation . .4 2.2 Biological Pathways and DNA/RNA Binding Proteins . .5 2.3 Copy Number Variation . .6 2.4 Gene Co-expression Network . .7 2.5 Protein-Protein Interaction Networks . .8 2.6 TADA Framework . .8 3 Related Work 10 3.1 DAWN . 10 3.2 Genome-wide Ranking by SVM-based Classifier . 11 3.3 DAMAGES Score . 12 3.4 ST-Steiner . 13 vi CONTENTS vii 4 Methods 14 4.1 Construction of Gene Co-Expression Networks . 14 4.2 Ground Truth Gene Sets . 16 4.3 DeepASD Model . 17 4.3.1 Graph Convolutional Network . 17 4.3.2 Early Stopping . 18 4.3.3 Weight Decay . 18 4.3.4 Dropout Regularization . 18 4.3.5 Mixture of Experts . 19 4.3.6 Cross-validation Setting . 19 4.3.7 Optimizer and Loss Function . 20 5 Results 22 5.1 Comparison against the State-of-the-art Methods . 22 5.2 Enrichment Analysis . 28 5.3 CNV Region Analysis . 32 5.4 Neurodevelopmental Period Analysis . 37 5.5 Evaluation of Edge Case Predictions . 39 5.6 Protein-Protein Interactions between ASD Genes . 42 CONTENTS viii 6 Conclusion 44 A Supplementary Tables 60 List of Figures 4.1 The architectural model of DeepASD for genome-wide ASD gene risk assessment. The model uses TADA features as well as 52 gene co-expression networks extracted from BrainSpan dataset. The feature set includes de novo loss of function (and missense) mu- tation counts, transmitted mutation counts for control and case groups, pLI value, de novo mutation frequency values and protein truncating variant counts. TADA dataset is used by all GCN mod- ules and the gating network. This whole system produces a single probability valuey ^ for each of the 25,825 genes and it is end-to-end trainable. 21 5.1 ROC and precision-recall curve distribution comparison between DeepASD and Krishnan et al. (a) Area under ROC curve distri- bution between the two methods. (b) Area under precision-recall curve distributions comparing the same methods as in (a). Both in (a) and (b), outlier points are depicted. The solid center line depicts the median, dashed line depicts the mean value for each panel. Box limits demonstrates lower and upper quartiles and whiskers denote 1.5 interquartile range. 22 ix LIST OF FIGURES x 5.2 Process of smoothing SVM output values. Using ten-fold cross- validation, 10 different isotonic regression model is fit. Then, we find the knots in each model and combine them to obtain (a). The transitions are not smooth and further smoothing is required. (b) Isotonic regression is applied once more on this knot collection to obtain a smoother curve. (c) Linear interpolation is used to obtain final mapping. 25 5.3 Probability value scatter plot of DeepASD and Krishnan et al. (a) Probability values of E1 genes against all other genes for both methods. (b) Non-mental-health related genes compared to all other genes. Both panels contain the same 25,825 genes. y = x line (gray) is also drawn for visual aid. 26 5.4 Precision-recall curves for DeepASD, DAWN (PFC-MSC3-5 and PFC-MSC4-6), Krishnan et al. and Zhang and Shen DAMAGES score. (a) The curve for E1 plus non-mental-health genes. (b) E1 + E2 genes are used. (c), all gold standard genes are used. All precision-recall curves have a cutoff rank of 2000. (c) has starting rank value of 5 whereas (a) and (b) have starting rank value of 1.
Details
-
File Typepdf
-
Upload Time-
-
Content LanguagesEnglish
-
Upload UserAnonymous/Not logged-in
-
File Pages96 Page
-
File Size-