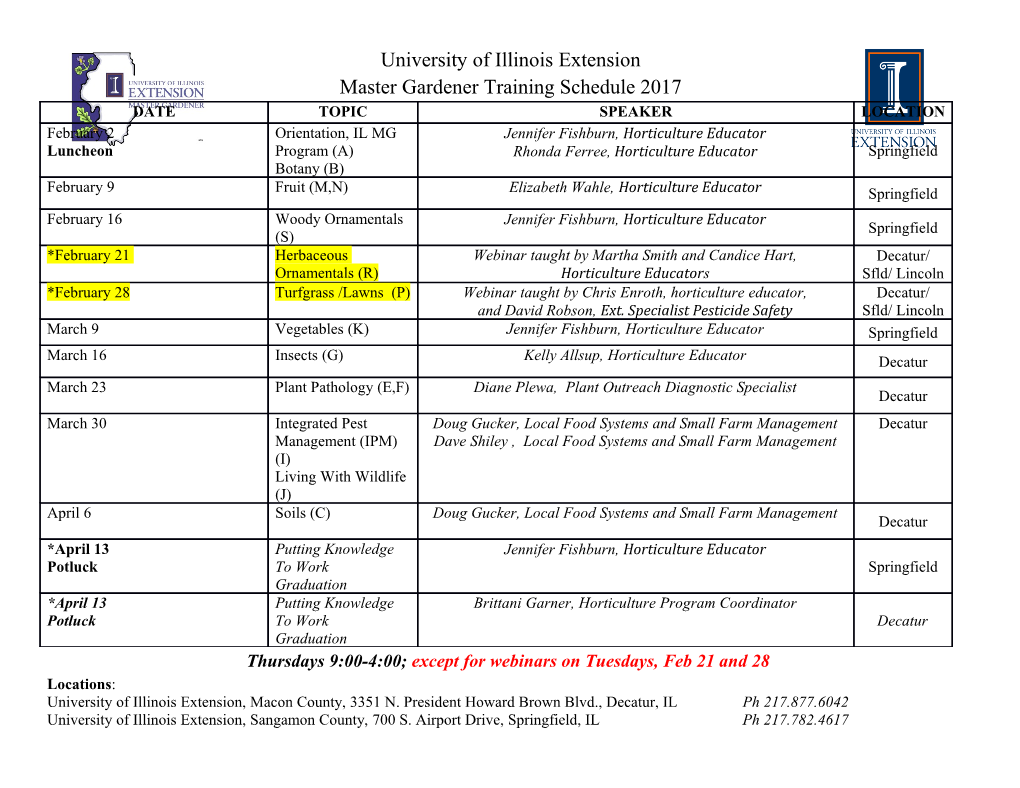
Céline Bonfils*, Kate Marvel, Benjamin Santer, Thomas Phillips Lawrence Livermore National Laboratory External influences on precipitation mean Ruby Leung state and variability Pacific Northwest National Laboratory Contact: [email protected] The relative contribution of drought Historical changes in zonal-mean Identification of human fingerprint Observed trend is compatible with precursors may change in precipitation in zonal-mean precipitation externally forced climate response to global warming Smooth and Zonally average DJF Precipitation Projection analysis Fingerprint = Spa6al pa7ern expected in response to external forcing (left) Wind circulation patterns in each hemisphere help transport moist equatorial air toward the poles. We project observed D(t) and T(t) anomalies onto fingerprint: Introduction These loops are driven by such properties as Earth’s How do we get it? size, rotation rate, atmospheric depth, and heating. = measure the similarity between 1980-2012 data and fingerprint 60°N (below) A smoothed map of satellite data reveals 1. Average over historical simulaons (world with us) à beat down noise Changes in global (ocean and land) precipitation are among the distinct zones of wet and dry land produced by the (uncorrelated noise across simulaons) àThe posi(ve trend in projec(on (me-series means that the fingerprint is circulation patterns. Livermore researchers used this Cool, dry air information to detect changes in the location and present and growing in the observaons. most important and least well-understood consequences of climate Desert 2. Fingerprint = first EOF of mulmodel average àextract mode of variability descends intensity of global precipitation over the past three change. decades. explaining most of the variance 30°N Equatorial tropical peak In an idealized world… Air cools, Midlatitude storm tracks Subtropical dry zones clouds 90°N § In a simple case (Collins et al. 2010)… form, Inflection points AVempt to remove ENSO first has rain falls no impact (mul(variate fingerprint 60°N Fingerprint expected in response to external is an efficient noise filter) Tropicsropics 30°N Thermal forcing 0° equator: warm, moist air 0° rises Latitude 30°S Desert 60°S Storm Thermod Wet track 30°S 90°S tropics Storm 0 1 2 3 4 5 6 track Smoothed precipitation, millimeters per day wet-get-wetter .: • Will#ENSO#change#in#the#future?# • Is#this#already#occurring#(detec3on)?## 60°S Observed trend cannot be explained by • Can#we#a8ribute#it#to#specific#external# • Will#ENSO#teleconnec3ons#change#in# forcings#(a8ribu3on)?# the#future,#invalida3ng#this#scenario?# internal variability dry zone dry zone We project D(t) and T(t) anomalies from hundreds 33-year periods of unforced climate Rendering by Kwei-Yu Chu (world without us) to obtain noise (me-series There is no reason for the fingerprint to project, except by chance ENSO: primary source of drought variability ENSO: primary source of drought variability in many Dynamic: poleward expansion à Typical Signal-to-noise problem inregions many of regions the globe via via “teleconnections”teleconnections Thermodynamic indicator T(t) We also project D(t) and T(t) anomalies Walker circulation Natural external forcing only from runs forced with natural forcings CMIP3 data with ozone depletion 0.5 CMIP3 data without ozone depletion alone, or various combinaons of CMIP5 data with ozone depletion Preindustrial control El!Niño! Primary noise mode (ENSO) natural and human forcings. Best fit 0.4 OBS ENSO mode cannot project on our fingerprint ! This multivariate fingerprint acts as an automatic noise filter •!Warmer/we)er!tropical! 0.3 eastern!Pacific!! Probability, density Probability, •!Drier!than!usual!in! 0.2 absence of trends in either Do t or To t , if thermodynamic This method identifies physical effects that are robust across Thermod Indonesia!and!part!of! Wet ð Þ ð Þ Thermod changes and dynamic changes increasingly occur in tandem. multiple models, even in thetropics presence of model errors. We have Australia! Storm Moisture transport P!!! fi fi fi track It is, of course, possible to calculate single-variable ngerprints to identi ed a ngerprintStorm pattern that characterizes the simulta- 0.1 track • wet-get-wetter .: ! Equatorward!retreat!of!! examine changes in Do t (SI Appendix, S6.1)orTo t (SI Appendix, neous response of precipitation location and wet-get-wetter .: intensity to ex- subtropical!dry!zones! ð Þ ð Þ This graph shows the probability distribution of the signal fi fi S6.2)separately.Thenoise ltering aspect of the multivariate ternal forcing and acts as a noise lter. Observed changes in this (that is, similarity of the fingerprint relative to the noise from naturally occurring climate variability). The observed 0 fi –6 –4 –2 0 2 4 6 ngerprint is lost in the single-variable cases: natural variability, multivariate response are incompatible with our best estimates signal matches predictions generated by climate models 1980-2012 intensity anomaly at peaks/troughs dry zone that include both natural and human-induced external Signal-to-noise ratio ENSO in particular, will project onto these fingerprints and of natural variability and consistent with model predictions of forcings. How wet is the weVest latude? How dry is the driest? Rendering by Kwei-Yu Chu decrease the S/N ratio (SI Appendix,Fig.S8). However, con- externally forced change. The synchronicity of these changes is Alternative drought drought precursors precursors expected expected in a sidering each variable separatelyallowsforcomparisonswith key, however: considering either changedry zone in isolation does not lead warmingin a warming world world previous studies that have detected changes in the hydrological to detection and attribution (SI Appendix, Fig. S3). By focusing on Equatorward contraction cycle or atmospheric circulation. both the underlying mechanisms that drive changes in global Thermodynamic- Dynamic- Coupled- We find, considering the dynamical indicator alone, that the precipitation, and by restricting our analysis to the large scales Alexander 2011 fi observations do show a poleward shift in the main features of where we have some con dence in models’ ability to reproduce the Dynamic indicator D(t) This work, in a more global context global precipitation. As previous authors (14) have found, the current climate, we have shown that the changes observed in the observed trend is much larger than the trends found in forced satellite era are externally forced, and likely to be anthropogenic Future changes in P will be increasingly driven by human- model runs, although including anthropogenic stratospheric ozone in nature. Acknowledgements induced mechanisms superimposed to intensified historical depletion reduces the discrepancy. Santer et al 2007 ACKNOWLEDGMENTS. We are very grateful to Ben Santer and Karl Taylor Seidel et al 2007 Although other studies (7, 26) have found evidence for thermo- ENSO-driven P variability. for helpful comments and infinite patience. This work was supported by the dynamic changes in the hydrological cycle, we do not detect a trend Intensifica7on-of-current-zonal- La7tudinal-redistribu7on-of- Global Climate Modeling Program of the US Department of Energy (DOE) wet;dry-pa<erns-- global-precipita7on-- in the thermodynamic indicator alone. This is due to differences in Office of Science and was performed under the auspices of the US DOE Reduc7on-in-local-soil- datasets and time periods considered (SI Appendix,Figs.S4–S6). Lawrence Livermore National Laboratory (LLNL) (Contract DE-AC52- ! Clausius;Clapeyron:-water-vapor- ! Ozone-deple7on-and-increasing- moisture-and-P-recycling-- increases-~-exp(T)- GHG-both-expected-to-shiQ- Our method is designed to detect changes in the zonal-mean 07NA27344). K.M. was supported by an LLNL Laboratory Directed Research circula7on-poleward- and Development award (tracking code 13-ERD-032). C.B. was supported by ! Held-&-Soden-2006:-wet-regions-get- structure of global precipitation; other metrics, designed to capture we<er,-dry-regions-get-drier- ! Observa7onal-evidence-that-major- her DOE Early Career Research Program award. We acknowledge the World features-are-shiQing-poleward- more local changes, have found evidence for regional thermody- Climate Research Programme’sWorkingGrouponCoupledModelling,which namic changes (27) that exceed model predictions. is responsible for CMIP, and we thank the climate modeling groups (listed in SI Appendix,TableS2of this paper) for producing and making available Can we see theses changes emerge in the observations? Conclusions their model output. For CMIP the US DOE’s Program for Climate Model Di- agnosis and Intercomparison provides coordinating support and led devel- Results In this paper, we have presented a simple method to track opment of software infrastructure in partnership with the Global Organization thermodynamic and dynamic changes in global precipitation. for Earth System Science Portals. Methods 1980-2012 latude anomaly at peaks/troughs This study provides evidence that human activities are affecting precipitation over Where are the weVest and driest latudes? Alexander, L. (2011) Extreme heat rooted in dry soils, Nature Geoscience, 4, 12–13. land and oceans. Anthropogenic increases in greenhouse gases and stratospheric Observaons: Global Precipitaon Climatology Project (GPCP); 33 1. Stott PA, et al. (2010) Detection and attribution of climate change: A regional per- Collins,15. Seager M. et al. (2010) R, Naik The impact N, Vecchi of global GAwarming (2010) on the Thermodynamictropical Pacific ocean and
Details
-
File Typepdf
-
Upload Time-
-
Content LanguagesEnglish
-
Upload UserAnonymous/Not logged-in
-
File Pages1 Page
-
File Size-