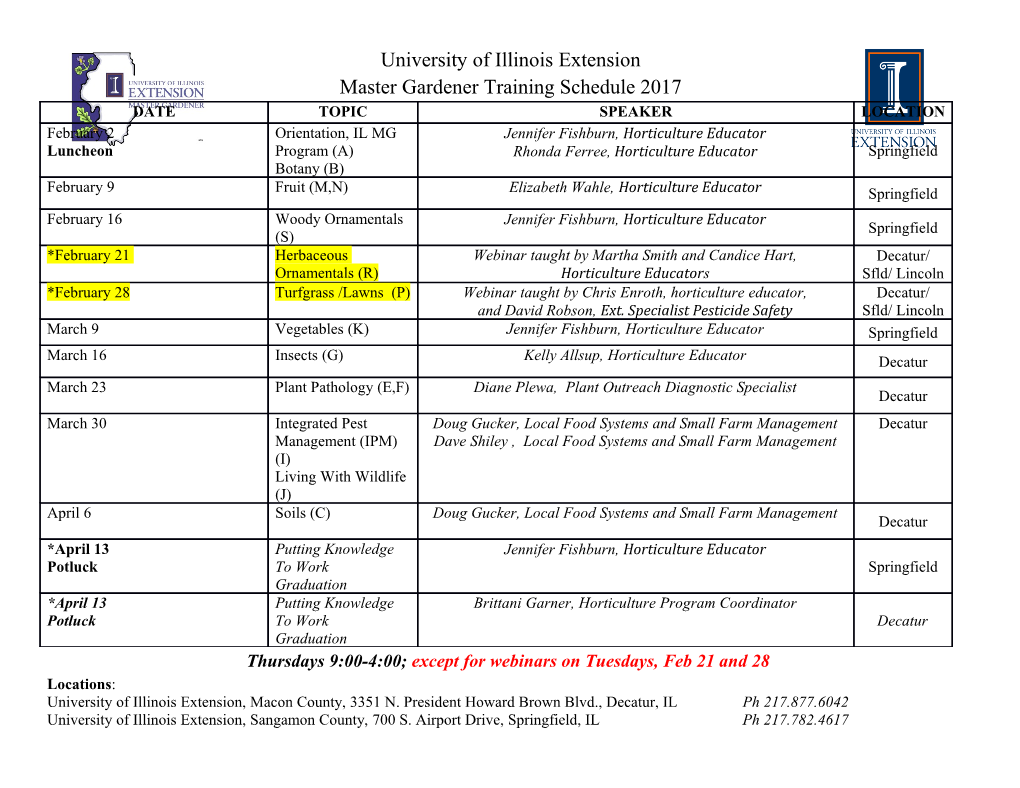
APUNet: Revitalizing GPU as Packet Processing Accelerator Younghwan Go, Muhammad Asim Jamshed, YoungGyoun Moon, Changho Hwang, and KyoungSoo Park School of Electrical Engineering, KAIST GPU-accelerated Networked Systems • Execute same/similar operations on each packet in parallel • High parallelization power • Large memory bandwidth CPU GPU Packet Packet Packet Packet Packet Packet • Improvements shown in number of research works • PacketShader [SIGCOMM’10], SSLShader [NSDI’11], Kargus [CCS’12], NBA [EuroSys’15], MIDeA [CCS’11], DoubleClick [APSys’12], … 2 Source of GPU Benefits • GPU acceleration mainly comes from memory access latency hiding • Memory I/O switch to other thread for continuous execution GPU Quick GPU Thread 1 Thread 2 Context Thread 1 Thread 2 Switch … … … … a = b + c; a = b + c; d =Inactivee * f; Inactive d = e * f; … … … … v = mem[a].val; … v = mem[a].val; … Memory Prefetch in I/O background 3 Memory Access Hiding in CPU vs. GPU • Re-order CPU code to mask memory access (G-Opt)* • Group prefetching, software pipelining Questions: Can CPU code optimization be generalized to all network applications? Which processor is more beneficial in packet processing? *Borrowed from G-Opt slides *Raising the Bar for Using GPUs in Software Packet Processing [NSDI’15] 4 Anuj Kalia, Dong Zhu, Michael Kaminsky, and David G. Anderson Contributions • Demystify processor-level effectiveness on packet processing algorithms • CPU optimization benefits light-weight memory-bound workloads • CPU optimization often does not help large memory workloads • GPU is more beneficial for compute-bound workloads • GPU’s data transfer overhead is the main bottleneck, not its capacity • Packet processing system with integrated GPU w/o DMA overhead • Addresses GPU kernel setup / data sync overhead, and memory contention • Up to 4x performance over CPU-only approaches! 5 Discrete GPU • Peripheral device communicating with CPU via a PCIe lane Host GPU PCIe Lanes CPU DRAM L2 Cache Streaming Multiprocessor (SM) High computation power Graphics Processing x N Scheduler Registers High memory bandwidth Cluster Fast inst./data access Fast context switch Instruction Cache GDDR Device Memory L1 Cache Shared Memory Require CPU-GPU DMA transfer! 6 Integrated GPU • Place GPU into same die as CPU share DRAM • AMD Accelerated Processing Unit (APU), Intel HD Graphics Host CPU Unified Northbridge DRAM No DMA transfer! GPU High computation power Graphics Northbridge L2 Cache Fast inst./data access Compute Compute Unit Fast context switch Unit Low power & cost Scheduler Registers L1 x N Cache APU 7 CPU vs. GPU: Cost Efficiency Analysis • Performance-per-dollar on 8 popular packet processing algorithms • Memory- or compute-intensive • IPv4, IPv6, Aho-Corasick pattern match, ChaCha20, Poly1305, SHA-1, SHA-2, RSA • Test platform • CPU-baseline, G-Opt (optimized CPU), dGPU w/ copy, dGPU w/o copy, iGPU CPU / Discrete GPU APU / Integrated GPU CPU Intel Xeon E5-2650 v2 (8 @ 2.6 GHz) CPU AMD RX-421BD (4 @ 3.4 GHz) GPU NVIDIA GTX980 (2048 @ 1.2 GHz) GPU AMD R7 Graphics (512 @ 800 MHz) RAM 64 GB (DIMM DDR3 @ 1333 MHz) RAM 16 GB (DIMM DDR3 @ 2133 MHz) Cost CPU: $1143.9 dGPU: $840 Cost iGPU: $67.5 8 Cost Effectiveness of CPU-based Optimization • G-Opt helps memory-intensive, but not compute-intensive algorithms • Computation capacity as bottleneck with more computations IPv6 table lookup AC pattern matching SHA-2 2 1.8 2.5 4 3.5 Detailed analysis on CPU-based optimization2.0 in the paper 1.5 2 3 1.5 1.0 2 1 1.0 1 1.0 1.0 1 0.5 0.5 0 Normalized perf per dollar Normalized perf per dollar Normalized perf per dollar Normalized perf per dollar 0 0 CPU G-Opt dGPU CPU G-Opt CPU G-Opt w/ copy 9 Cost Effectiveness of Discrete/Integrated GPUs • Discrete GPU suffers from DMA transfer overhead • Integrated GPU is most cost efficient! IPv4 table lookup ChaCha20 2048-bit RSA decryption 3.5 20 16 14.4 3.08 Our approach: 17.0 3 12 2.5 Use integrated GPU15 to accelerate packet processing! 2 10 8 1.5 1.00 1.08 4.8 1 5 4.3 4 0.5 1.0 1.0 0 0 0 Normalized perf perNormalized dollar perf per dollar Normalized perf per dollar G-Opt dGPU dGPU Normalized perf per dollar G-Opt dGPU iGPU G-Opt dGPU iGPU w/ copy w/o copy w/o copy w/o copy 10 Contents • Introduction and motivation • Background on GPU • CPU vs. GPU: cost efficiency analysis • Research Challenges • APUNet design • Evaluation • Conclusion 11 Research Challenges Set input • Frequent GPU kernel setup overhead Redundant Launch kernel • Overhead exposed w/o DMA transfer overhead! Teardown kernel Retrieve result • High data synchronization overhead APU • CPU-GPU cache coherency CPU Bottleneck! Explicit DRAM GPU Sync! BW: 10x↓ • More contention on shared DRAM Cache • Reduced effective memory bandwidth APUNet: a high-performance APU-accelerated network packet processor 12 Persistent Thread Execution Architecture • Persistently run GPU threads without kernel teardown • Master passes packet pointer addresses to GPU threads CPU Shared Virtual Memory (SVM) GPU Master Thread 0 Thread 1 Workers Packet Thread 2 Packet I/O Packet Thread 3 Packet NIC Packet Pool Pointer Array Persistent Threads Packet 13 Data Synchronization Overhead • Synchronization point for GPU threads: L2 cache • Require explicit synchronization to main memory Shared Virtual Memory P P P P P Master CPU GPU Thread 0 Thread 1 Graphics L2 Thread 2 Thread 3 Update result? Northbridge Cache Thread 4 Thread 5 Need explicit Can process one Thread 6 Thread 7 sync! request at a time! 14 Solution: Group Synchronization • Implicitly synchronize group of packet memory GPU threads processed • Exploit LRU cache replacement policy Shared Virtual Memory Master P P P P P Group 1 Group 2 32 0 For more details andVerify tuning/optimizations, please refer to our CPUpaper correctness! GPU GPU Thread Group 1 GPU Thread Group 2 GPU Thread 0 Thread 31 Thread 0 L2 Cache Poll Poll Poll DP DP DP Process Process Dummy Mem I/O DP DP DP Barrier Barrier Barrier 15 Zero-copy Based Packet Processing Option 1. Traditional method with discrete GPU Option 2. Zero-copy between CPU-GPU NIC CPU COPY GPU NIC COPY CPU GPU Standard (e.g., mmap) GDDR (e.g., cudaMalloc) Standard (e.g., mmap) Shared (e.g., clSVMAlloc) High overhead! High overhead! • Integrate memory allocation for NIC, CPU, GPU NIC CPU GPU No copy overhead! Shared (e.g., clSVMAlloc) 16 Evaluation APUNet (AMD Carrizo APU) 40 Gbps NIC Client (packet/flow generator) RX-421BD (4 cores @ 3.4 GHz) Mellanox ConnectX-4 Xeon E3-1285 v4 (8 cores @ 3.5 GHz) R7 Graphics (512 cores @ 800 MHz) 32GB DRAM 16GB DRAM • How well does APUNet reduce latency and improve throughputs? • How practical is APUNet in real-world network applications? 17 Benefits of APUNet Design • Workload: IPsec (128-bit AES-CBC + HMAC-SHA1) Packet Processing Latency Synchronization Throughput GPU-Copy GPU-ZC GPU-ZC-PERSIST (64B Packet) 6 20 5.31 5 16 4 12 3 5.7x 8 2 5.4x Throughput (Gbps) 0.93 Packet (us) latency Packet 4 1 1.5x 0 0 64 128 256 512 1024 1451 Atomics Group sync Packet size (bytes) 18 Real-world Network Applications • 5 real-world network applications • IPv4/IPv6 packet forwarding, IPsec gateway, SSL proxy, network IDS IPsec Gateway SSL Proxy CPU Baseline G-Opt APUNet CPU Baseline G-Opt APUNet 20 5000 16.4 4241 3583 15 4000 2x 3000 1801 1540 2.75x 10 7.7 8.2 1791 5.3 2000 1539 5 2.6 2.8 1000 HTTP trans/sec HTTP Throughput (Gbps) 0 0 64 1451 256 8192 Packet size (bytes) Number of concurrent connections 19 Real-world Network Applications • Snort-based Network IDS Network IDS • Aho-Corasick pattern matching CPU Baseline G-Opt APUNet DFC 12 9.710.4 • No benefit from CPU optimization! 10 • Access many data structures 8 6 4x • Eviction of already cached data 3.6 3.6 4.2 4 2.6 2.3 2.4 2 • DFC* outperforms AC-APUNet Throughput (Gbps) 0 • CPU-based algorithm 64 1514 • Cache-friendly & reduces memory access Packet size (bytes) *DFC: Accelerating String Pattern Matching for Network Applications [NSDI’16] Byungkwon Choi, Jongwook Chae, Muhammad Jamshed, KyoungSoo Park, and Dongsu Han 20 Conclusion • Re-examine the efficacy of GPU-based packet processor • GPU is bottlenecked by PCIe data transfer overhead • Integrated GPU is the most cost effective processor • APUNet: APU-accelerated networked system • Persistent thread execution: eliminate kernel setup overhead • Group synchronization: minimize data synchronization overhead • Zero-copy packet processing: reduce memory contention • Up to 4x performance improvement over CPU baseline & G-Opt APUNet High-performance, cost-effective platform for real-world network applications 21 Thank you. Q & A.
Details
-
File Typepdf
-
Upload Time-
-
Content LanguagesEnglish
-
Upload UserAnonymous/Not logged-in
-
File Pages22 Page
-
File Size-