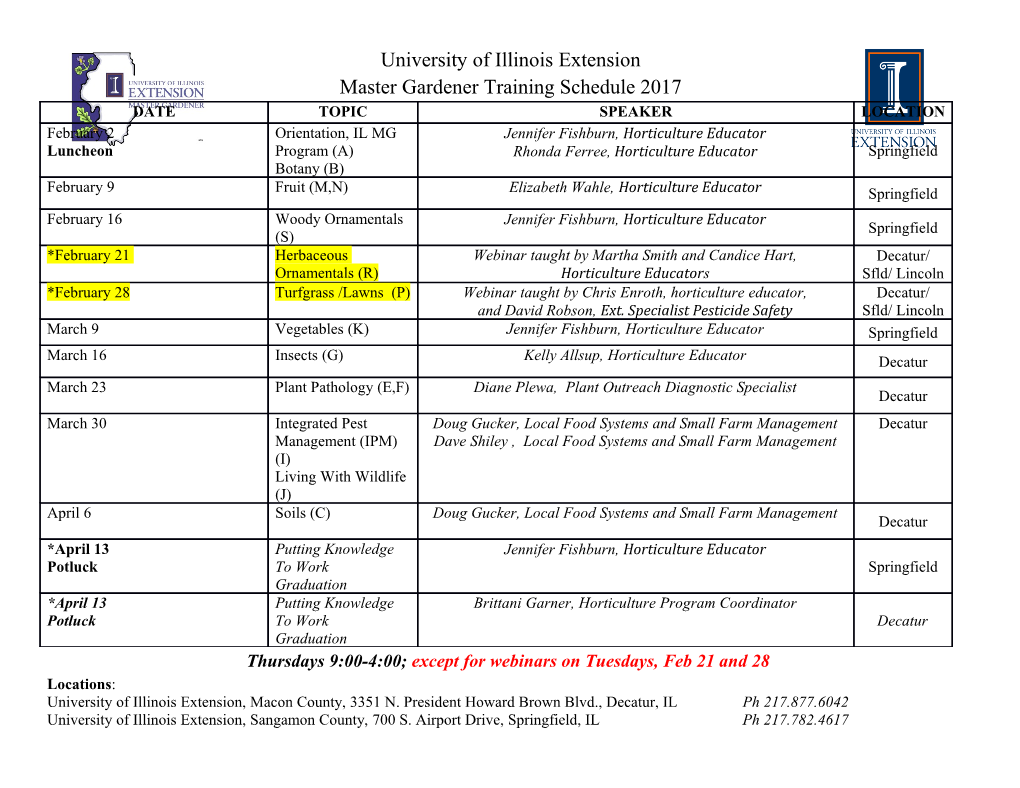
OFFPRINT Fractal boundaries of complex networks Jia Shao, Sergey V. Buldyrev, Reuven Cohen, Maksim Kitsak, Shlomo Havlin and H. Eugene Stanley EPL, 84 (2008) 48004 Please visit the new website www.epljournal.org TAKE A LOOK AT THE NEW EPL Europhysics Letters (EPL) has a new online home at www.epljournal.org Take a look for the latest journal news and information on: • reading the latest articles, free! • receiving free e-mail alerts • submitting your work to EPL www.epljournal.org November 2008 EPL, 84 (2008) 48004 www.epljournal.org doi: 10.1209/0295-5075/84/48004 Fractal boundaries of complex networks Jia Shao1(a),SergeyV.Buldyrev1,2, Reuven Cohen3, Maksim Kitsak1, Shlomo Havlin4 and H. Eugene Stanley1 1 Center for Polymer Studies and Department of Physics, Boston University - Boston, MA 02215, USA 2 Department of Physics, Yeshiva University - 500 West 185th Street, New York, NY 10033, USA 3 Department of Mathematics, Bar-Ilan University - 52900 Ramat-Gan, Israel 4 Minerva Center and Department of Physics, Bar-Ilan University - 52900 Ramat-Gan, Israel received 12 May 2008; accepted in final form 16 October 2008 published online 21 November 2008 PACS 89.75.Hc – Networks and genealogical trees PACS 89.75.-k – Complex systems PACS 64.60.aq –Networks Abstract – We introduce the concept of the boundary of a complex network as the set of nodes at distance larger than the mean distance from a given node in the network. We study the statistical properties of the boundary nodes seen from a given node of complex networks. We find that for both Erd˝os-R´enyi and scale-free model networks, as well as for several real networks, the boundaries have fractal properties. In particular, the number of boundaries nodes B follows a − power law probability density function which scales as B 2. The clusters formed by the boundary nodes seen from a given node are fractals with a fractal dimension df ≈ 2. We present analytical and numerical evidences supporting these results for a broad class of networks. Copyright c EPLA, 2008 Many complex networks are “small world” due to the role in several scenarios, such as in the spread of viruses very small average distance d between two randomly or information in a human social network. If the virus chosen nodes. Often d ∼ ln N,whereN is the number of (information) spreads from one node to all its nearest nodes [1–6]. Thus, starting from a randomly chosen node neighbors, and from them to all next nearest neighbors following the shortest path, one can reach any other node and further on until d,howmanynodesdonot get the in a very small number of steps. This phenomenon is called virus (information), and what is their distribution with “six degrees of separation” in social networks [4]. That is, respect to the origin of the infection? for most pairs of randomly chosen people, the shortest In this letter, we find theoretically and numerically “distance” between them is not more than six. Many that the nodes at the boundaries, which are of order random network models, such as Erd˝os-R´enyi network N, exhibit similar fractal features for many types of (ER) [1], Watts-Strogatz network (WS) [5] and scale-free networks, including ER and SF models as well as several network (SF) [3,6–8], as well as many real networks, have real networks. Song et al. [9] found that some networks been shown to possess this small-world property. have fractal properties while others do not. Properties Much attention has been devoted to the structural of fractal networks were also studied [10,11]. Here we properties of networks within the average distance d from a show that almost all model and real networks includ- given node. However, almost no attention has been given ing non-fractal networks have fractal features at their to nodes which are at distances greater than d from a boundaries. given node. We define these nodes as the boundaries of Figure 1 demonstrates our approach and analysis. For the network and study the ensemble of boundaries formed each “root” node, we call the nodes at distance ℓ from it by all possible starting nodes. An interesting question is: “nodes in shell ℓ”. We choose a random root node and howmany“friendsoffriendsoffriendsetc....”hasoneat count the number of nodes Bℓ at shell ℓ.Weseethat a distance greater than the average distance d?Whatis B1 = 10, B2 = 11, B3 = 13, etc. We estimate the aver- their probability distribution and what is the structure age distance (diameter) d ≈ 2.9 by averaging the distances of the boundaries? The boundaries have an important between all pairs of nodes. After removing nodes with ℓ<d≈ 2.9, the network is fragmented into 12 clusters, (a) E-mail: [email protected] with sizes s3={1, 1, 2, 5, 1, 3, 1, 1, 8, 1, 2, 3}. Note that the 48004-p1 Jia Shao et al. 0 10 l=11 1- 10 l=12 β= 0.1 l 2- 10 l=13 P(B ) -3 10 4- )a( ER 10 0 2 4 6 shell 1 10 10 10 10 Bl 0 shell 2 10 1- 10 cluster of 8 nodes β= 0.1 l 2- 10 P(B ) 3- l=8 Fig. 1: (Color online) Illustration of shells and clusters origi- 10 l=11 nating from a randomly chosen root node, which is shown in l=10 4- l=9 the center (red). Its neighboring nodes are defined as shell 1 10 )b( SF (green), the nodes at distance ℓ are defined as shell ℓ.When 0 2 4 6 10 10 10 10 removing all nodes with ℓ<3, the remaining network (purple) Bl becomesfragmentedinto12clusters. 0 10 l =9 l=10 boundary of the network is always seen from a given node, 1- 10 β= 0.1 thus not a unique set of nodes in the network. l l =11 We begin by simulating ER and SF networks, and l=12 P(B ) 2- then we present analytical proofs. Figure 2a shows 10 simulation results for the number of nodes Bℓ reached from a randomly chosen origin node for an ER network. -3 )c( HEP The results shown are for a single network realization 10 0 1 2 3 4 of size N =106, with average degree k =6 and d ≈ 7.9 10 10 10 10 10 Bl (see footnote 1). For ℓ<d, the cumulative distribution 0 function, P (Bl), which is the probability that shell 10 ℓ has more than Bℓ nodes, decays exponentially for ∗ ∗ B >B ,whereB is the maximum typical size of shell ℓ l=4 ℓ ℓ ℓ 1- l=6 l=5 (see footnote 2). However, for ℓ>d, we observe a clear 10 transition to a power law decay behavior, where l β= 0.1 −β -2 l=7 P (Bℓ) ∼ Bℓ ,withβ ≈ 1andthepdfofBℓ is 1P(B ) 0 ˜ −2 P (Bℓ) ≡ dP (Bℓ)/dBℓ ∼ Bℓ . For different networks, the 3- emergence of the power law can occur at shell ℓ = d +1 or 10 (d) AS ℓ = d + 2. Thus, our results suggest a broad “scale-free” 0 1 2 3 4 10 10 10 10 10 distribution for the number of nodes at distances larger B than d. This power law behavior demonstrates that there l is no characteristic size and a broad range of sizes can Fig. 2: (Color online) The cumulative distribution function, appear in a shell at the boundaries. P (Bℓ), for two random network models: (a) ER network In SF networks, the degrees of the nodes, k, follow with N =106 nodes and k = 6, and (b) SF network with −λ a power law distribution function q(k) ∼ k ,wherethe N =106 nodes and λ =2.5, and two real networks: (c) the minimum degree of the network, kmin,ischosentobe2. High Energy Particle (HEP) physics citations network and Figure 2b shows, for SF networks with λ =2.5, similar (d) the Autonomous System (AS) Internet network. The shells −β power law results, P (Bℓ) ∼ Bℓ ,withβ ≈ 1forℓ>d with ℓ>d are marked with their shell number. The thin lines from left to right represent shells ℓ =1, 2, ..., respectively, with ℓ<d.Forℓ>d, P (Bℓ) follows a power law distribution 1Different realizations yield similar results. In one realization, − − P (B ) ∼ B β , with β ≈ 1 (corresponding to P˜(B ) ∼ B 2 for a certain fraction of nodes are randomly taken to be origin. The ℓ ℓ ℓ ℓ the pdf). The appearance of a power law decay only happens histogram is obtained from Bℓ belonging to different origin nodes. 2 The behavior of the pdf of Bℓ for ℓ<d will be discussed later for ℓ larger than d ≈ 7.9 for ER and d ≈ 4.7 for the SF network. and is shown in fig. 3c. The straight lines possess slopes of −1. 48004-p2 Fractal boundaries of complex networks 0 10 N=200 8 a( ) ER N=400 10 l=5 1- N=800 l=6 10 N=8000 6 l N=32000 10 θ= .3 0 =7 2- N=128000 6 l 10 N=10 4 l 10 3- n(s ) 10 <B >/N 2 10 4- 10 0 10 )a( SF 5- 10 0 2 4 6 6- -4 2- 0 2 4 6 8 10 10 10 10 l (- lnN nl/ <k )> sl 4 8 10 10 ER N=128000 l=5 6 l=7 3 ER N=10 6 l=8 10 SF N=128000 10 θ=3.0 6 1 SF N=10 l 4 2 1l 0 10 ~ k + n(s ) 2 1 10 10 ( )b 0 )b( HEP 0 10 10 0 1 2 3 4 1 2 3 4 5 6 7 8 9 10 11 12 13 10 10 10 10 10 l sl Fig.
Details
-
File Typepdf
-
Upload Time-
-
Content LanguagesEnglish
-
Upload UserAnonymous/Not logged-in
-
File Pages8 Page
-
File Size-