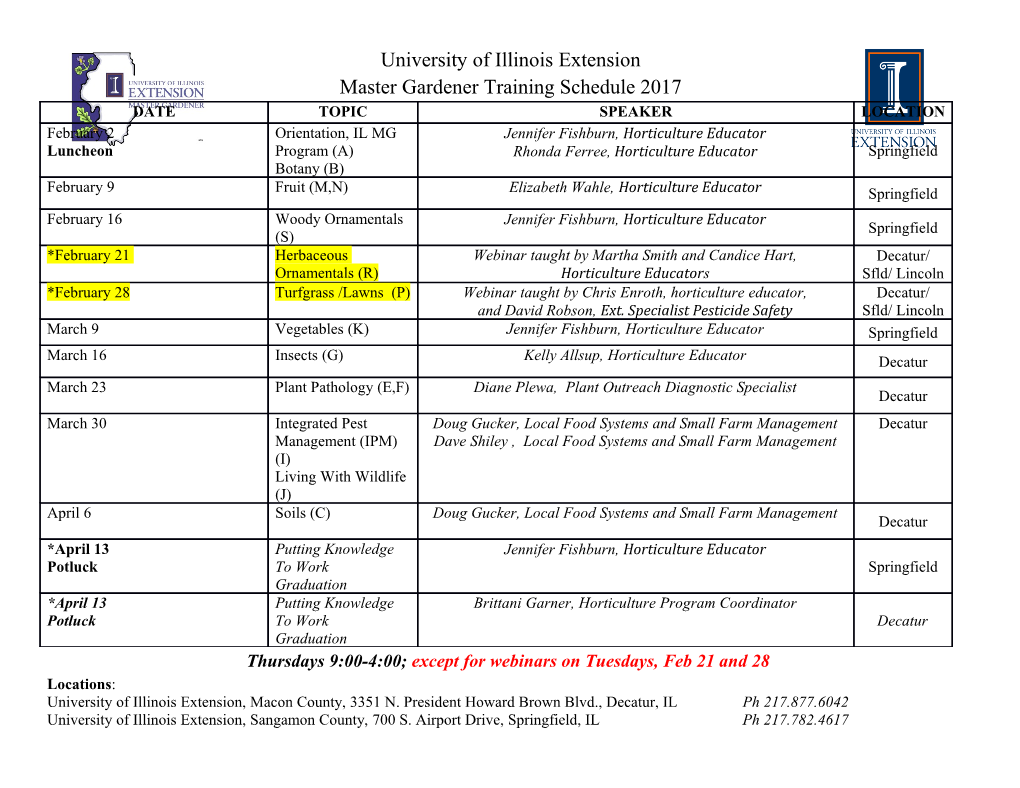
Asymptotic Theory of Robustness a short summary Matthias Kohl \Mathematical Statistics" University of Bayreuth Contents 1 Introduction2 2 L2 differentiability2 3 Local Asymptotic Normality4 4 Convolution Representation and Asymptotic Minimax Bound5 5 Asymptotically Linear Estimators7 5.1 Definitions....................................7 5.2 Cram´er-Rao Bound...............................9 6 Infinitesimal Robust Setup 10 7 Optimal Influence Curves 13 7.1 Introduction................................... 13 7.2 Bias Terms................................... 15 7.3 Minimum Trace Subject to Bias Bound................... 16 7.4 Mean Square Error............................... 17 References 18 2 L2 DIFFERENTIABILITY Author Index 19 Subject Index 21 1 Introduction In this technical report we give a short summary of results contained in Chapters 2 - 5 of [Rieder, 1994] where we restrict our considerations to the estimation of a finite- dimensional parameter in the one sample i.i.d. case (i.e., no testing, no functionals). More precisely, we assume a parametric family P = fPθ j θ 2 Θg ⊂ M1(A) (1.1) of probability measures on some sample space (Ω; A), whose parameter space Θ is an k open subset of some finite dimensional R . Sections2-5 contain a short abridge of some classical results of asymptotic statistics. For a more detailed introduction to this topics we also refer to Chapter 2 of [Bickel et al., 1998] and Chapters 6 - 9 of [van der Vaart, 1998], respectively. In the infinitesimal robust setup introduced in Sec- tion6, the family P will serve as ideal center model and at least under the null hypothesis Pθ 2 P the observations y1; : : : ; yn at time n 2 N are assumed to be i.i.d.. Finally, in Section7 we give the solutions (i.e., optimal influence curves) to the optimization prob- lems motivated in Subsection 7.1. For the derivation of optimal influence curves confer also [Hampel, 1968] and [Hampel et al., 1986]. 2 L2 differentiability To avoid domination assumptions in the definition of L2 differentiability, we employ the following square root calculus that was introduced by Le Cam. The following definition is taken from [Rieder, 1994]; for more details confer Subsection 2.3.1 of [Rieder, 1994]. Definition 2.1 For any measurable space (Ω; A) and k 2 N we define the following real k Hilbert space that includes the ordinary L2(P ) p k k L2(A) = fξ dP j σ 2 L2(P );P 2 Mb(A)g (2.1) On this space, an equivalence relation is given by p Z p p ξ dP ≡ ηpdQ () jξ p − η q j2dµ = 0 (2.2) k where j · j denotes the Euclidean norm on R and µ 2 Mb(A) may be any measure, depending on P and Q, so that dP = p dµ, dQ = q dµ. We define linear combinations 2 L2 DIFFERENTIABILITY with real coefficients and a scalar product by p p p αξ dP + βηpdQ = (αξ p + βη q )pdµ (2.3) p Z p hξ dP j ηpdQ i = ξτ η pq dµ (2.4) We fix some θ 2 Θ and define L2 differentiability of the family P at θ using this square root calculus; confer Definition 2.3.6 of [Rieder, 1994]. Here Eθ denotes expectation taken under Pθ. Definition 2.2 Model P is called L2 differentiable at θ if there exists some function k Λθ 2 L2(Pθ) such that, as t ! 0, p p 1 τ k dPθ+t − dPθ (1 + t Λθ)k k = o(jtj) (2.5) 2 L2 and τ Iθ = Eθ ΛθΛθ 0 (2.6) The function Λθ is called the L2 derivative and the k × k matrix Iθ Fisher Information of P at θ. Remark 2.3 A concise definition of L2 differentiability for arrays of probability mea- sures on general sample spaces may be found in Section 2.3 of [Rieder, 1994]. //// We now consider a parameter sequence (θn) about θ of the form t θ = θ + pn t ! t 2 k (2.7) n n n R Corresponding to this parametric alternatives (θn) two sequences of product measures are defined on the n-fold product measurable space (Ωn; An) n n n O n O Pθ = Pθ Pθn = Pθn (2.8) i=1 i=1 Theorem 2.4 If P is L2 differentiable at θ, its L2 derivative Λθ is uniquely determined k in L2(Pθ). Moreover, Eθ Λθ = 0 (2.9) and the alternatives given by (2.7) and (2.8) have the log likelihood expansion dP n τ n θn t X 1 τ 0 log = p Λθ(yi) − t Iθt + oP n (n ) (2.10) dP n n 2 θ θ i=1 where n ! 1 X n p Λ (y ) P −!w N (0; I ) (2.11) n θ i θ θ i=1 Proof: This is a special case of Theorem 2.3.7 in [Rieder, 1994]. //// 3 LOCAL ASYMPTOTIC NORMALITY 3 Local Asymptotic Normality We first state a result of asymptotic statistics that is known as Le Cam's third lemma. Theorem 3.1 Let Pn;Qn 2 M1(An) be two sequences of probabilities with log likeli- hoods L 2 log dQn , and S a sequence of statistics on (Ω ; A ) taking values in some n dPn n n n p p p p×p finite-dimensional (R¯ ; B¯ ) such that for a; c 2 R , σ 2 [0; 1) and C 2 R , Sn a C c (Pn) −!w N 2 ; τ 2 (3.1) Ln −σ =2 c σ then Sn a + c C c (Qn) −!w N 2 ; τ 2 (3.2) Ln σ =2 c σ (3.3) Proof: [Rieder, 1994], Corollary 2.2.6. //// The following definition corresponds to Definition 2.2.9 of [Rieder, 1994]. Definition 3.2 A sequence (Qn) of statistical models on sample spaces (Ωn; An), Qn = fQn;t j t 2 Θng ⊂ M1(An) (3.4) k k with the same finite-dimensional parameter space Θn = R (or at least Θn " R ) is called asymptotically normal, if there exists a sequence of random variables Zn : (Ωn; An) ! k k (R ; B ) that are asymptotically normal, Zn(Qn;0) −!w N (0;C) (3.5) k×k k with positive definite covariance C 2 R , and such that for all t 2 R the log likelihoods L 2 log dQn;t have the approximation n;t dQn;0 1 L = tτ Z − tτ Ct + o (n0) (3.6) n;t n 2 Qn;0 The sequence Z = (Zn) is called the asymptotically sufficient statistic and C the asymp- totic covariance of the asymptotically normal models (Qn). We now state Remark 2.2.10 of [Rieder, 1994] where we add a part (c) and (d). Remark 3.3 (a) The covariance C is uniquely defined by (3.5) and (3.6). And (3.6) implies that another sequence of statistics W = (Wn) is asymptotically sufficient iff 0 Wn = Zn + oQn;0 (n ). (b) Neglecting the approximation, the terminology of asymptotically sufficient may be justified in regard to Neyman's criterion; confer Proposition C.1.1 of [Rieder, 1994]. One speaks of local asymptotic normality if, as in section2, asymptotic normality depends on suitable local reparametrizations. 4 CONVOLUTION REPRESENTATION AND ASYMPTOTIC MINIMAX BOUND (c) The notion of local asymptotic normality { in short, LAN { was introduced by [Le Cam, 1960]. (d) The sequence of statistical models Q = fQ j Q = P n g given by the alterna- n n;t n;t θn p1 Pn tives (2.7) and (2.8) is LAN with asymptotically sufficient statistic Zn = n i=1 Λθ(yi) and asymptotic covariance C = Iθ. //// 4 Convolution Representation and Asymptotic Minimax Bound In this section we present the convolution and the asymptotic minimax theorems in the parametric case; confer Theorems 3.2.3, 3.3.8 of [Rieder, 1994]. These two mathematical results of asymptotic statistics are mainly due to Le Cam and H´ajek. Assume a sequence of statistical models (Qn) on sample spaces (Ωn; An), Qn = fQn;t j t 2 Θng ⊂ M1(An) (4.1) k k with the same finite-dimensional parameter space Θn = R (or Θn " R ). The parameter of interest is Dt for some p × k-matrix D of full rank p ≤ k. Moreover we consider asymptotic estimators p p S = (Sn) Sn : (Ωn; An) ! (R ; B ) (4.2) The following definition corresponds to Definition 3.2.2 of [Rieder, 1994]. Definition 4.1 An asymptotic estimator S is called regular for the parameter transform p k D, with limit law M 2 M1(B ), if for all t 2 R , (Sn − Dt)(Qn;t) −!w M (4.3) k that is, Sn(Qn;t) −!w M ∗ IDt as n ! 1, for every t 2 R . Remark 4.2 For a motivation of this regularity assumption we refer to Example 3.2.1 of [Rieder, 1994]. The Hodges estimator introduced there is asymptotically normal but superefficient. However it is not regular in the sense of Definitions 4.1. Moreover, in the light of the asymptotic minimax theorem (Theorem 4.5), the Hodges estimator has maximal estimator risk; confer [Rieder, 1994], Example 3.3.10. //// We now may state the convolution theorem. Theorem 4.3 Assume models (Qn) that are asymptotically normal with asymptotic p×k covariance C 0 and asymptotically sufficient statistic Z = (Zn). Let D 2 R be a matrix of rank p ≤ k. Let the asymptotic estimator S be regular for D with limit law p M. Then there exists a probability M0 2 M1(B ) such that −1 τ M = M0 ∗ N (0; Γ) Γ = DC D (4.4) and −1 (Sn − DC Zn)(Qn;0) −!w M0 (4.5) 4 CONVOLUTION REPRESENTATION AND ASYMPTOTIC MINIMAX BOUND An asymptotic estimator S∗ is regular for D and achieves limit law M ∗ = N (0; Γ) iff ∗ −1 0 Sn = DC Zn + oQn;0 (n ) (4.6) Proof: Three variants of the proof are given in [Rieder, 1994], Theorem 3.2.3.
Details
-
File Typepdf
-
Upload Time-
-
Content LanguagesEnglish
-
Upload UserAnonymous/Not logged-in
-
File Pages22 Page
-
File Size-