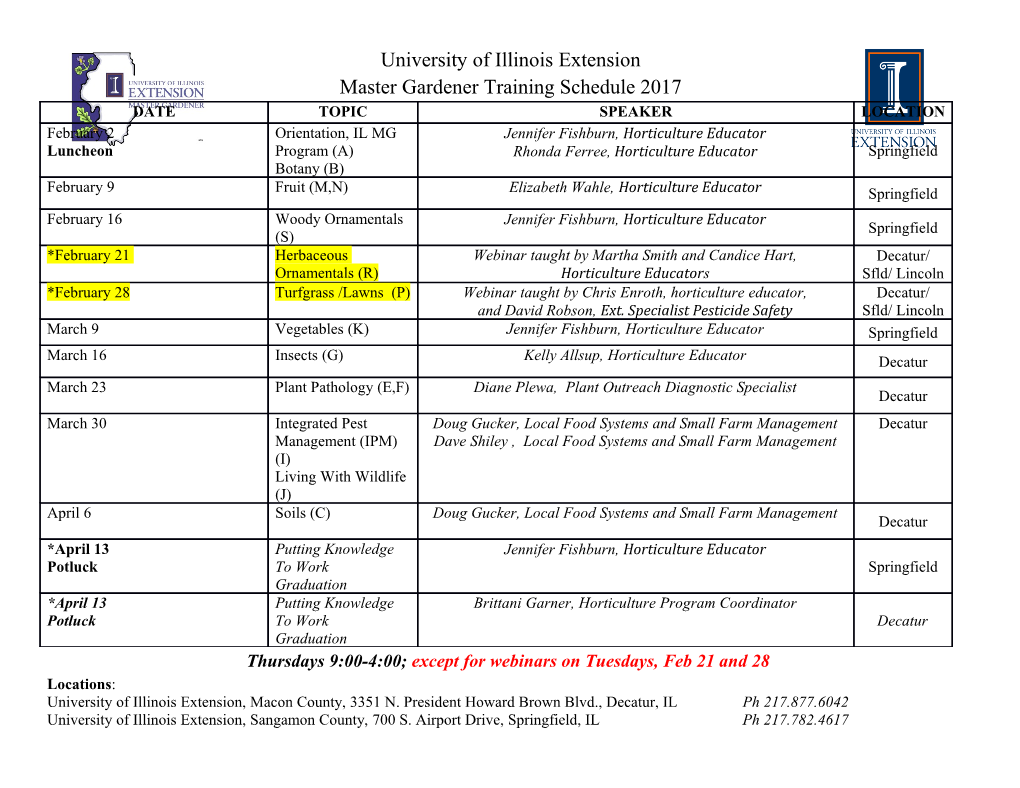
The Economic Impact of Administrative Annexation in Jiangsu Province, China -----Spatial Filtering Perspective Shaofei Chen ([email protected]) Melissa Rura ([email protected] ) 1 6/2/2008 Statement of the Problem With the rapid development of China, here specifically the Jiangsu province, spatial patterns of administration such as metropolises and their extensions have been formed in past decades. Researchers have been more concerned about economic study on these static patterns instead of the change of regional governance. Here we evaluate the impact of local administrative annexation in Jiangsu province from a spatial filtering perspective. 2 6/2/2008 Background The changes in city and county-level units’ administrative relationships have gone four stages in history after P. R. China was established. Here we consider the fourth stage. From 2000 to 2002, a large scale adjustment was performed in Jiangsu. Prefecture -level cities annexed counties or county-level cities and transformed the latter as the urban districts of the former. 3 6/2/2008 Background Zhang and Wu (2006) discuss this issue from a public policy point of view. Although they state this reconsolidation had some positive impact on economic development, they question whether annexation positive because confliction still exist. Brenner(1999) uses cases in EU to discuss this issue but because Chinese administrative structure and its political and economic regulation is unique, his result may not be suitable here. 4 6/2/2008 Research Question Has this wave of annexation resulted in a greater economic integration between central cities and peripheral areas? Will the change of economic development mechanism of the annexation regions differ from other places? 5 6/2/2008 Data Prefecture-level Annexed county-level city units Jiangning county Nanjing city Luhe county Jiangpu county Zhengjiang city Dantu county Changzhou city Wujin city Wuxi city Xishan city Suzhou city Wuxian city Yangzhou city Hanjiang county Yancheng city Yandu county In order to analyze the efforts of Huaiying city annexation, this study collected data before Huaian city Huaiying county the transition (1999) and after the transition (2003 and 2004). Suqian city Suyu county 6 6/2/2008 Model and Variables Using the Douglas production function (see Yu, 2006), modify the model to represent the regional development mechanism. ln(pGDPi )=+ββ01 *ln( pFIXINVi ) + β 2 *ln( pFDI i ) + β 3 *ln( pTSRS i ) + β 4 *ln( pFINEXP i ) + ε pGDP: GDP (Gross Domestic Product) Per capital pFIXINV: fixed asset investment Per capital pFDI: foreign direct investment Per capital pTSRS: total social retail sales Per capital pFINEXP: local financial expense Per capital 7 6/2/2008 Spatial Filtering Eigenvector spatial filtering extends a conventional regression equation by including orthogonal synthetic variables capturing map patterns that account for latent spatial autocorrelation in data. Constructed as linear combinations of eigenvectors extracted from a geographic connectivity matrix, defined in terms of adjacency or distance, representing the spatial structure of a dataset. Spatial filtering with covariate maps, includes interaction terms between eigenvectors and all covariates, as well as the intercept. 8 6/2/2008 Link Matrix and Eigenvectors ABCD A 0 1 1 0 B 1 0 0 1 C 1 0 0 1 D 0 1 1 0 The eigenvectors are calculated from (I – 11 T/n) C(I – 11 T/n) 9 6/2/2008 Spatial Filtering with Coefficient Maps – Normal Linear Model (Tiefelsdorf and Griffith, 2007) Spatial filters 10 6/2/2008 Spatial filter theorem Eigenvectors are extracted from a spatial link matrix exhibit distinctive spatial patterns with associated spatial autocorrelation levels. Furthermore, these eigenvectors are mutually orthogonal and uncorrelated. The linear combination of the chosen eigenvectors captures the hidden spatial pattern in a stochastic component of a model. P K Y = β 0 1 + ∑ Xpβ p + ∑Ekβ E + ε k p=1 k=1 11 6/2/2008 GWR and its critique yi = β 0 (ui ,vi ) + ∑ β k (ui ,vi )xik +ε i k ˆ T −1 T b(u i ,vi ) = (X W(ui ,vi )X) X W(ui ,vi )y 1 exp[ − (d / b) 2 ] 2 ij 2 2 [1− (dij / b) ] if d ij < b Otherwise W ( u i , v i ) =0 Problems related with GWR: 1. high levels of multicollinearity amongst estimates (Wheeler and Tiefelsdorf, 2005) 2. loss of degrees of freedom (Griffith, 2008 in press) 12 6/2/2008 SF-GWR P K Y = β0 1 + ∑ X pβ p + ∑Ekβ E + ε conventional SF model k p=1 k=1 P ˆ (Griffith, 2008 in press) Y = β0, GWR + ∑Xp • βp., GWR p=1 K0 P Kp ≈ (β0 1+ ∑Ek βk ) + ∑(βp 1+ ∑Ek βk ) •Xp , 0 0 p p k0 =1 p=1 kp =1 P K P K Y = β 0 1 + ∑ X p • 1β p + ∑Ekβ E + ∑∑ Xp • Ekβ pE + ε k k p=1 k=1 p=1 k =1 global effects local effects 13 6/2/2008 Results R-Square (Compare with GWR) OLS SF-GWR GWR.gauss GWR.bi-sqaure 1999 0.8792 0.9451 0.8953 0.8962 2003 0.9349 0.9685 0.9609 0.9501 2004 0.9308 0.971 0.9361 0.9336 14 6/2/2008 Result 15 6/2/2008 Result not significant 16 6/2/2008 Result 17 6/2/2008 Result not significant 18 6/2/2008 Result not significant not significant 19 6/2/2008 Result 99-03FDI 99-04FDI 99-03FIX 99-04FIX Zhengjiang City 97.25 -54.12 14.18 33.35 Nanjing City 79.97 -25.52 17.55 95.55 Dantu County 121.1 -18.93 14.59 91.55 Jiangning City 90.66 -36.35 13.63 75.80 Danyang City 127.95 -29.85 7.31 40.34 Jiangpu County 78.46 -48.62 15.00 48.67 Yangzhong City 54.95 -1.28 38.04 88.33 Luhe County 64.58 -49.26 17.59 45.52 Jurong City 120.68 -20.96 8.45 100 Lishui City 105.30 -38.35 10.09 67.64 Yangzhou City 51.32 -47.52 29.09 50.07 Gaochun City 97.47 -50.56 10.82 39.11 Hanjiang City 22.36 -26.91 38.63 80.35 Wuxi City 144.06 -44.06 -23.40 -88.62 Baoying County 109.41 -95.24 -13.60 -38.04 Xishan City 183.70 -42.04 -42.67 -168.95 Yizhen City 45.25 -39.4 25 64.74 Jiangyin City 174 -43.27 -28.63 -130.57 Gaoyou City 44.18 -48.01 27.37 51.23 Yixing City 129.68 -38.86 -9.39 -7.53 Jiangdu City -32.23 -8.83 54.48 101.62 Changzhou City 129.75 -51.23 -4.31 -37.58 Yancheng City 124.31 -88.18 -18.61 -54.71 Wujin City 169 -27.18 -25.1 -68.64 Yandu County 125.46 -90.61 -21.08 -61.28 Jingtan City 145.98 -23.73 -4.24 48.28 Xiangshui County 84.99 -143.98 0.17 -6.24 Liyang City 121.6 -33.25 2.64 59 Binghai County 108.92 -125.96 -13.58 -35.12 Suzhou City 120.45 -55.39 -4.77 -51.72 Funing County 120.48 -139.19 -29.96 -66.05 Wuxian City 165.26 -46.12 -34.66 -151.94 Sheyang County 131.98 -107.61 -31.49 -78.46 Zhangjianggang 162.78 -50.88 -20.04 -103.61 Jianhu County 131.23 -111.63 -36.01 -84.22 Kunshan City 154.85 -50.11 -21.06 -111.64 Dongtai City 15.6 -46.99 39.93 46.71 Wujiang City 120.45 -55.39 -4.77 -51.72 Dafeng City 108.08 -78.23 -2.08 -27.52 Taicang City 135.79 -55.35 -5.66 -56.39 Huaian City 113.8 -139.3 -26.5 -56.24 Changshu City 187.48 -45.89 -38.63 -170.98 Huaiying County 57.39 -141.90 11.42 17.98 Suqian City 51.36 -60 24.54 35.48 Huaiying City 95.29 -123.3 -4.55 -13.79 Suyu County -136.75 -65 60.12 103.97 Hongze County 102.66 -97.22 -6.49 -20.64 Suyang County -127.13 -195.17 53.63 97.58 Xuyu County 78.65 -67.19 13.53 15.44 Siyang County -11.84 -95.68 38.99 66.51 Jinghu County 88.22 -71.75 6.75 4.04 Sihong County 6.06 -55.05 37.41 60.38 20 6/2/2008 Conclusion Spatial filtering model is a powerful analysis tool to study regional sciences. It furnishes a way to include non-spatially varying coefficients, such as regional indicator variables or gradient (i.e., trend surface) components. In this study, it reveals that the administrative annexations happened in Jiangsu province are mostly for political reasons. The changes of those areas are not significant. 21 6/2/2008 References Brenner, N. (1999) Globalization as reterritorialisation: the re-scaling of urban governance in the European Union, Urban Studies 36, 431–452 Griffith, D. A. (2008) Spatial filtering-based contributions to a critique of geographically weighted regression (GWR), Environment and Planning A, forthcoming Tiefelsdorf, M. and Griffith, D. A. (2007) Semiparametric filtering of spatial autocorrelation: the eigenvector approach, Environment and Planning A , 39 (1), 193 - 1221 Wheeler , D. and Tiefelsdorf, M. (2005), Multicollinearity and correlation among local regression coefficients in geographically weighted regression. Journal of Geographic Systems 7: 161 -187 Yu, D.L. (2006) Spatially varying development mechanisms in the Greater Beijing Area: a geographically weighted regression investigation, Ann Reg Sci 40:173–190 Zhang, J.X. and Wu, F.L. (2006) China’s Changing Economic Governance: Administrative Annexation and the Reorganization of Local Governments in the Yangtze River Delta, Regional Studies, 40(1), 3–21 22 6/2/2008 Thank you very much! Any questions? 23 6/2/2008.
Details
-
File Typepdf
-
Upload Time-
-
Content LanguagesEnglish
-
Upload UserAnonymous/Not logged-in
-
File Pages23 Page
-
File Size-