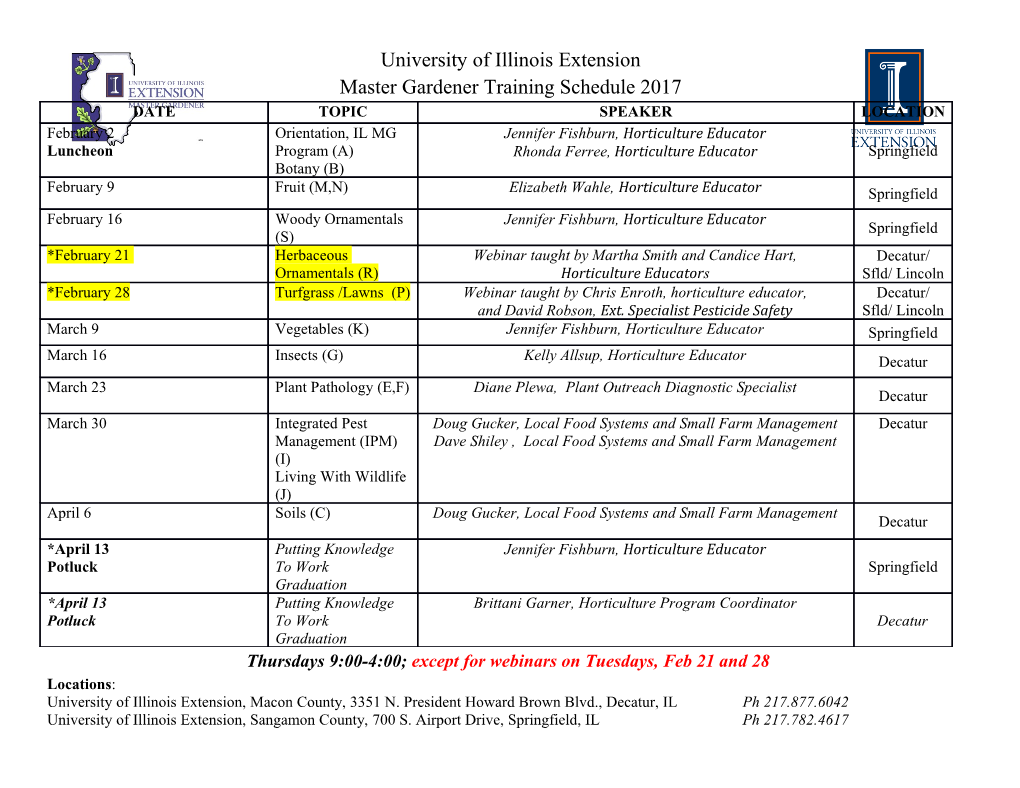
Bayes Risk as an Alternative to Fisher Information in Determining Experimental Designs for Nonparametric Models David S. Bayard, Roger Jelliffe, and Michael Neely Laboratory of Applied Pharmacokinetics Children’s Hospital Los Angeles Los Angeles, CA, USA Supported by NIH Grants GM 068968 and HD 070886 1 Methods of Optimal Design • D-optimality (traditional) maximizes the determinant of the Fisher Information Matrix (Fedorov 1972 [20], Silvey 1980 [19] ) – Intended for parameter estimation problems [11][12] – Modifications for robustness: ED, EID, API, etc. • Multiple Model Optimal (MMOpt) Design (new) minimizes an overbound on the Bayes risk – Can be evaluated without numerical integration or Monte Carlo simulation/stochastic analysis – Intended for multiple model (MM) parametrizations which form the basis of the USC BestDose software • The multiple model representation corresponds to the support points in the nonparametric population model – Based on recent theoretical overbound on Bayes Risk (Blackmore et. al. 2008 [4]) 2 3 D-Optimal Design for Nonlinear Problems • D-Optimal design tells you where to place samples so that they are most sensitive to small changes in model parameter values – max |M|, where M is Fisher Information Matrix, and |(.)| is determinant – Useful tool has become standard in design of clinical experiments • Problem with D-optimal design - Circular reasoning (Silvey 1980): – You need to know the true patient’s parameters before D-optimal design can be used to compute the best experiment to find these same parameters • To robustify D-optimal design, an expectation is taken with respect to prior information (c.f., Chaloner [13]), Pronzato [14][15], Tod [16], D’Argenio [17]), ED: max E{ |M| } EID: min E{ 1/|M| } ELD (or API): max E{ log|M| } • MMopt comparison will be made here relative to ED, EID and API. Not exhaustive - other experiment design criteria are possible (cf., Chaloner [13], Merle and Mentre [18]) 4 Definition of ED, EID, API • All above design metrics require Fisher Matrix M to be nonsingular, and hence require at least p samples to be taken, where p=# parameters 5 Multiple Model Optimal Design • USC BestDose software [3] is based on a multiple model (MM) parametrization of the Bayesian prior (i.e., discrete support points in the nonparametric population model) 10 Model Prior – Nonparametric Maximum Likelihood (NPML) 0.02 MM Prior estimation of a population model has the form 0.015 0.01 of a MM prior (Mallet [5], Lindsay [6]). Prob 0.005 – Software for population NPML modeling is 0 2.5 available, e.g., NPEM (Schumitzky [7][11]), 0.12 0.1 2 0.08 K 0.06 NPAG (Leary [8], Baek [9]), USC*PACK (Jelliffe 0.04 V 1.5 0.02 [10]). Kel Vs • Experiment design for MM (i.e., discrete) models is a subject found in classification theory – How do we sample the patient to find out which support point he best corresponds to? – Classifying patients is fundamentally different from trying to estimate patient’s parameters • Treating MM experiment design in the context of classification theory leads to the mathematical problem of minimizing Bayes risk (Duda et. al. [21]) 6 7 8 Simple 2-Model Example Model Responses 1 Model1 Model2 0.8 Dopt 1 Dopt 2 0.6 0.4 0.2 0 0 2 4 6 8 10 Time 9 Model Response Separation r(t) Model Responses • Model Response Separation r(t) is the 1 separation between two model responses at a Model 1 Reponse Model 2 Response given time t 0.8 0.6 •Defines natural statistic for discriminating between two models 0.4 r(t) = response separation • Bayes Risk is shown in gray area 0.2 r(t)=response separation 0 0 2 4 6 8 10 Time Two-Model Classification Problem 2 • Bayes Risk (gray area) decreases as response 1.5 separation r(t) increases Pull Gaussians 1 Bayes apart to minimize • Models are best Risk gray area discriminated by sampling 0.5 at a time t that maximizes r(t) 0 10 -1 -0.5 0 0.5 1 1.5 Bayes Optimal Design Model Responses 1 Model 1 Reponse Model 2 Response 0.8 0.6 0.4 0.2 Bayes Optimal 0 0 2 4 6 8 10 Design Time Maximal Response Separation At t=1.188 t=1.188 11 MMopt for 2-Model Example Bayes Optimal design 1 For 2-Model problem, MMopt 0.8 gives identical solution as Bayes |(t,a )-(t,a )| Optimal Design at t=1.188 1 2 Model 1: (t,a ) 0.6 1 Both designs maximize response Model 2: (t,a ) separation (green curve) 2 0.4 True Bayes Risk MMopt Cost Bayes Optimal Sample 0.2 0 0 2 4 6 8 10 Time t=1.188 12 ED Design for 2-Model Example ED design at t=4 sec is very different from Bayes optimal design of t=1.188 ED Design compared to Bayes Optimal 25 20*(t,a ) 1 20*(t,a ) 20 2 |M(a )| 1 |M(a )| 15 2 ED Criterion is blind ED Cost to model response ED Optimal separation: 10 Bayes Optimal 5 0 0 2 4 6 8 10 Note: model responses have been rescaled t=1.188 Time to enable showing everything on one plot t=4 13 2-Model Results Design Metric Sample Time Bayes Risk Bayes Optimal 1.188 0.1393 MMOpt 1.188 0.1393 ED 4.000 0.2701 EID 0.5600 0.1827 API 0.8890 0.1462 14 2-Models with Close Parameters Model Responses 1 Model 1 Reponse Model 2 Response 0.8 0.6 0.4 0.2 0 0 2 4 6 8 10 Time 15 Results for 2-Models with Close Parameters Design Metric Sample Time Bayes Risk Bayes Optimal 0.9530 0.4767 MMOpt 0.9530 0.4767 ED 0.9570 0.4767 EID 0.9480 0.4767 API 0.9520 0.4767 D-Optimal 0.9524 0.4767 • MMopt agrees exactly with Bayes optimal design for this problem • Designs (ED, EID, API, D-Optimal) are close to Bayes Optimal design • Not coincidence: for two model responses close enough to differ by a first- order approximation in a Taylor expansion: – Maximizing Model response separation is similar to maximizing Fisher information – Goals of classification and parameter estimation are very similar 16 4-Model Example • Example where Bayes Optimal Design is too difficult to compute • Demonstrates usefulness of MMopt design Model Responses 3 2.5 Model Responses • Grid points t=0.05 apart 2 • Designs optimized over time grid 1.5 1 Model Parameters # a b 0.5 1 2 2.625 0 2 1 0.6 0 0.5 1 1.5 2 2.5 3 3.5 4 3 0.7 0.6 time (s) 4 0.5 0.6 17 4-Model Results Design Metric 2-Sample Times Bayes Risk Bayes Risk 99%Conf * MMOpt 0.45 1.4 0.32839 +/- 0.00070 ED 0 0.8 0.37028 +/- 0.00070 EID 0 1 0.36044 +/- 0.00072 API 0 0.95 0.36234 +/- 0.00072 Design Metric 3-Sample Times Bayes Risk Bayes Risk 99% Conf * MMOpt 0.45 1.4 1.4 0.28065 +/- 0.00067 ED 0 0.7 0.9 0.32048 +/- 0.00067 EID 0 0 1 0.36034 +/- 0.00072 API 0 0.85 0.105 0.3099 +/- 0.00069 • MMopt has smallest Bayes Risk of all designs studied • Bayes optimal design is too difficult to compute for this problem * evaluated based on Monte Carlo analysis using 1,400,000 runs per estimate 18 Experiment Design under Reparametrization 19 PK Population Model with 10 Multiple Model Points - First-Order PK Model Dose Input 400 350 300 Dose input = 300 units for 250 200 1 hour, starting at time 0 Units 150 100 50 0 0 5 10 15 20 Time (hr) All subject responses 3.5 Model Parameters Model Responses 3 # K V • Grid points 15 min apart 1 0.090088 113.7451 2.5 • MMopt optimized over time grid 2 0.111611 93.4326 2 3 0.066074 90.2832 4 0.108604 89.2334 1.5 Concentration 5 0.103047 112.1093 1 6 0.033965 94.3847 7 0.100859 109.8633 0.5 8 0.023174 111.7920 0 9 0.087041 108.6670 0 5 10 15 20 25 Time (hr) 10 0.095996 100.3418 20 Example of MMopt Design with 10 Models 1-Sample-Time Case All subject responses with optimal 1 sample times Bayes Risk 4 overbound • Optimal 3.5 Sampling 3 Times (hr) 4.2500 2.5 2 Concentration 1.5 1 0.5 0 0 5 10 15 20 25 Time (hr) t=4.25 21 Example of MMopt Design with 10 Models 2-Sample-Time Case All subject responses with optimal 2 sample times • Optimal 3.5 Sampling Times (hr) 3 1.0000 9.5000 2.5 2 1.5 Concentration 1 0.5 0 0 5 10 15 20 25 Time (hr) t=1 t=9.5 22 Example of MMopt Design with 10 Models: 3-Sample-Time Case AllSamples subject responses 1 & with2 (duplicated optimal sample times at 1 hr) • Optimal 3.5 Sampling Times Sample 3 (10.5 hr) (hr) 3 1.0000 2.5 1.0000 10.5000 2 1.5 Concentration 1 0.5 0 0 5 10 15 20 25 Time (hr) t=1 t=10.5 23 Example of MMopt Design with 10 Models: 4-Sample-Time Case • Optimal AllSamples subject responses 1,2&3 with optimal(triplicate 4 sample at times 1 hr) 3.5 Sampling Times (hr) 3 Sample 4 (10.75 hr) 1.0000 1.0000 2.5 1.0000 2 10.7500 1.5 Concentration 1 0.5 0 0 5 10 15 20 25 Time (hr) t=1 t=10.75 24 Comparison Table ED EID API MMopt Invariant under regular linear Yes Yes Yes Yes reparametrization* Invariant under regular No No Yes Yes nonlinear reparametrization* Allows taking fewer than p No No No Yes samples, p= # of parameters Can handle heterogeneous No No No Yes model structures Gives known optimal solution No No No Yes to 2-model example Captures main elements of No No No Yes minimizing Bayes risk *Proved in Appendix 25 Summary • Multiple Model Optimal Design (MMOpt) provides an alternative to Fisher-Information Matrix based design – Particularly attractive for Nonparametric Models (MM discrete prior) – Based on true MM formulation of the problem (i.e., classification theory) – Has many advantages relative to ED, EID and API (see Table summary) – Based on recent theoretical overbound on Bayes Risk (Blackmore et.
Details
-
File Typepdf
-
Upload Time-
-
Content LanguagesEnglish
-
Upload UserAnonymous/Not logged-in
-
File Pages35 Page
-
File Size-