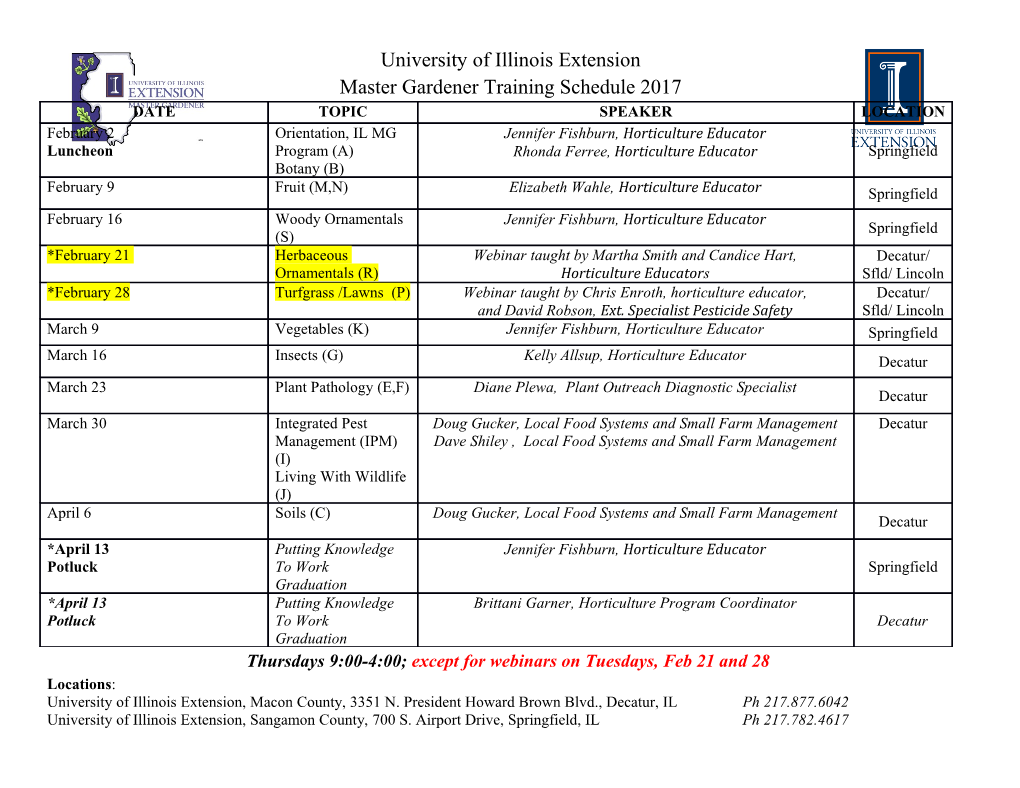
1840 MONTHLY WEATHER REVIEW VOLUME 133 Probabilistic Prediction of Tropical Cyclones. Part I: Position HARRY C. WEBER Meteorological Institute, University of Munich, Munich, Germany (Manuscript received 23 February 2004, in final form 14 December 2004) ABSTRACT A new objective aid for operational prediction of the positions of tropical cyclones is presented. Its method is based on a simple analysis of the performance of all operationally available numerical models during a training period. In a subsequent forecast period, the results of this analysis are used to produce both high-quality deterministic (as by-product) and probabilistic storm position forecasts in the form of geographical maps of strike probability for all prediction times out to 120 h from a given base date and time. The model was developed using operationally available position predictions of all global tropical-cyclone events of the years 2000, 2001, and 2002 as provided in the U.S. Navy’s Automated Tropical Cyclone Forecasting System (ATCF). Forecasts have been carried out for the years 2001 and 2002, with correspond- ing training periods 2000 and 2001. The global annual mean deterministic position errors for the years 2001 (2002) were 148 (136), 266 (235), 393 (329), 541 (435), and 733 (554) km at 24-, 48-, 72-, 96-, and 120-h prediction times, respectively. The deterministic mean errors and their corresponding standard deviations were found to be lower than those of most statistical and dynamical operational models and approximately equal to those of all consensus approaches currently in operational use. The main feature of the new method is the automatic production of geographical strike probability maps. For all tropical-cyclone events during the year 2001 (2002), the mean annual diameters of, for example, the 66% strike probability regions (i.e., the regions inside which future storm positions can be expected with a probability of 66%) at 24-, 48-, 72-, 96-, and 120-h prediction time were found to be 290 (280), 490 (480), 710 (660), 1020 (780), and 1310 (1020) km, respectively. At all prediction times, the predicted sizes of areas of given strike probability represent conservative estimates in that the observed percentages of storm positions inside these areas are larger than the corresponding expected percentages. 1. Introduction work of having to decide whether or not specific model predictions should be taken into consideration during The last decade has seen large progress in the quality the process of developing an official forecast. More- of the operational prediction of tropical cyclones. In over, as models normally provide only deterministic particular, statistical and numerical prediction models predictions of the track and intensity of tropical cy- have improved dramatically and form today’s basis for clones, it still lies mostly within the responsibility of the the high-quality track and intensity guidance provided experienced forecasters on duty to produce official by operational forecasters of national and international probabilistic forecasts in the form of geographical strike prediction centers. The reliability of even highly valued probability charts or intensity probability diagrams. prediction models may vary unpredictably, however, The present study aims to improve this situation by the from season to season as a consequence of model up- presentation and discussion of a simple automatic ob- dates or even from prediction to prediction, probably in jective aid based on an ensemble of all operationally response to the uncertainty in the initial conditions or available statistical and numerical prediction models for other, more obscure reasons. Thus, operational that can be utilized by operational forecasters to pro- forecasters still face the problem during their daily duce probabilistic forecasts of tropical cyclones. The generation of prediction ensembles for tropical- cyclone forecast models can be divided into two major Corresponding author address: Harry C. Weber, Meteorological Institute, University of Munich, Theresienstr. 37, 80333 Munich, categories: 1) following, for example, Leith (1974), a Germany. single numerical model can be integrated several times E-mail: [email protected] with respect to time, starting each time from an initial © 2005 American Meteorological Society Unauthenticated | Downloaded 09/29/21 03:56 AM UTC MWR2951 JULY 2005 W E B E R 1841 condition that differs slightly from all other initializa- of all global tropical-cyclone events during the years tions. The initial states can be generated in a variety of 2001 and 2002. In the second part of this paper (Weber ways, for example, by the application of singular vector 2005, hereafter Part II), the same approach is applied to decomposition methods (Molteni et al. 1996), breeding produce deterministic and probabilistic intensity fore- methods (Toth and Kalnay 1997), or Monte Carlo casts of tropical cyclones. methods (Mullen and Baumhefner 1994). Although a few case studies (e.g., Krishnamurti et al. 1997; Aber- son et al. 1998; Zhang and Krishnamurti 1999; Puri et 2. Datasets al. 2001) have documented some potential of this ap- proach, a long-term evaluation of the results of en- The datasets used in the present study consist of the semble predictions of tropical cyclones using one nu- objective aid files of the Automated Tropical Cyclone merical model for a general assessment of their opera- Forecasting System (ATCF; Sampson and Schrader tional value has not been carried out to date; 2) Goerss 2000) and cover all global tropical-cyclone events of the (2000), Goerss et al. (2004), Krishnamurti et al. (2000), years 2000, 2001, and 2002. Besides tropical-cyclone ad- and Weber (2003) suggest the generation of forecast visory information on storm structure, location, and ensembles by forecasts from a set of tropical-cyclone motion (see discussion below) at 6-hourly intervals— prediction models. In the method applied by Goerss et that is, at base times 0000, 0600, 1200, and 1800 UTC— al., the predicted positions of a number of numerical the ATCF objective aid files contain the predicted storm positions of all existing statistical and numerical models are averaged in space to produce a so-called models out to a maximum prediction time of 120 h from consensus prediction. During a “training period,” the any given base date and time. In consultation with C. R. approach of Krishnamurti et al. subjects the forecasts of Sampson of the Naval Research Laboratory (NRL), the an ensemble of dynamical models to a linear multiple use of prediction models was restricted only to those regression relative to the known best-track information that were operationally available at given base dates for the derivation of statistical weights of the perfor- and times. A list of these models, including some ref- mance of each ensemble member. In a subsequent erences for further reading, is given in Table 1. In this “forecast period,” the weighted individual forecasts of context it should be noted that, in contrast to the all ensemble members are used to produce track and method presented in Weber (2003), tropical-cyclone intensity predictions. Both of the above methods have advisory information is not used in PEST, in an effort to proven, at least for the case of track prediction, that the minimize the amount of a priori information necessary resulting forecasts are, on average, significantly better for the production of reliable position predictions. than those of individual models. In particular, the high As mentioned in the introduction and shown in the quality of the consensus method of Goerss (2000) and next section, PEST is based on a statistical evaluation of Goerss et al. (2004) has been demonstrated by the ex- model position predictions relative to corresponding cellent operational performance at the Joint Typhoon observed positions. The latter are listed in ATCF ob- Warning Center and the U.S. National Hurricane Cen- jective aid datasets containing best-track information. ter during the last few years. Position errors were computed using spherical geom- The prediction model presented in this study [the etry. Probabilistic Ensemble System for the Prediction of Tropical Cyclones (PEST)] belongs to the second of the above categories of ensemble prediction. It is similar to 3. Model description the statistical model of Weber (2003) inasmuch as it uses the results of a statistical evaluation of the predic- Following the terminology of Krishnamurti et al. tions of all operationally available statistical and nu- (2000), forecasts of PEST are produced on the basis of merical models relative to best-track information dur- a statistical evaluation of all operationally available ing a training period for the automatic production of storm predictions of statistical and numerical models probabilistic (and deterministic) forecasts of tropical and consensus combinations thereof (e.g., CON_, cyclones during a subsequent forecast period. The ap- SCON, etc.; cf. Table 1) during a training period. The proach taken in PEST is much simpler, however, and inclusion of consensus predictions in the ensemble is the amount of a priori information necessary to obtain based on the philosophy that they themselves constitute high-quality guidance is much smaller than that of Web- a set of valuable forecasts and that every piece of in- er (2003). The first part of the present paper focuses on formation available at a given base date and time the deterministic and probabilistic position prediction should be used for the production of probabilistic fore- Unauthenticated | Downloaded 09/29/21 03:56 AM UTC 1842 MONTHLY WEATHER REVIEW VOLUME 133 TABLE 1. Acronyms of all operationally available models used in PEST. Acronym Model AFWI Fifth-generation Pennsylvania State University–National Center for Atmospheric Research Mesoscale Model (MM5), U.S. Air Force version, coarse resolution AVNI, JAVI Variants of the Aviation model (Lord 1993; Surgi et al. 1998) BAM(D, M, S), (F, M, S)BAM Variants of the Beta and Advection Model (Marks 1992) CLIM Climatology from Typhoon Analog model (TYAN78; Ocean Data Systems, Inc.
Details
-
File Typepdf
-
Upload Time-
-
Content LanguagesEnglish
-
Upload UserAnonymous/Not logged-in
-
File Pages13 Page
-
File Size-