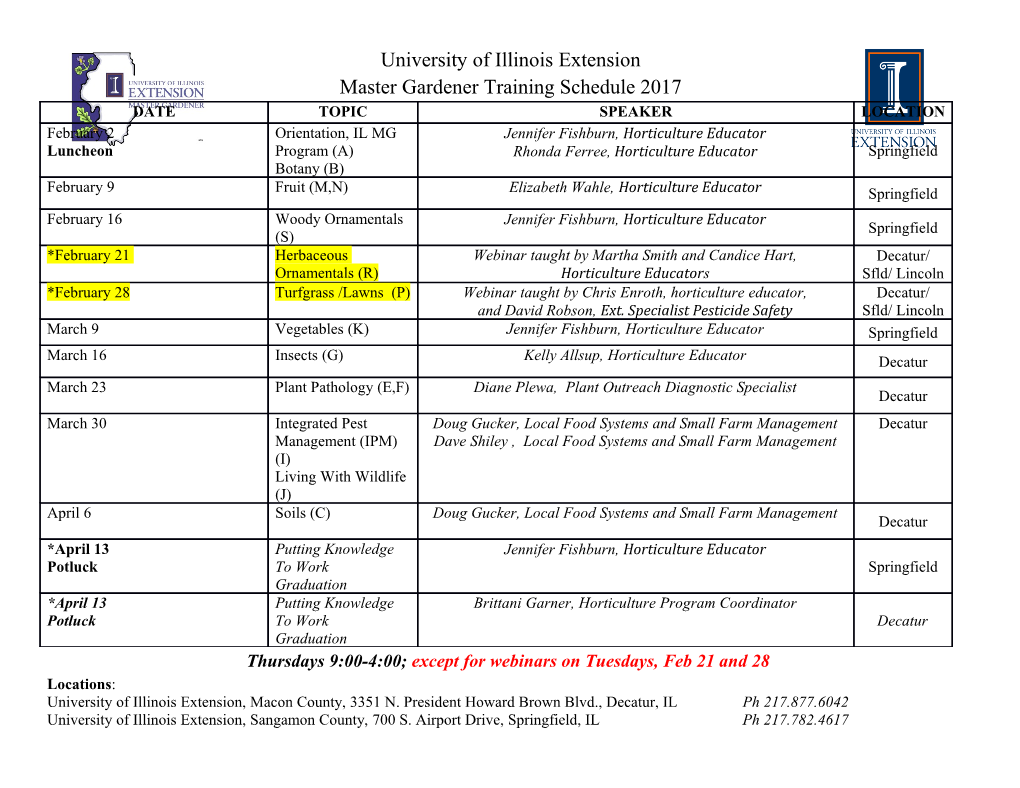
FAKULTÄT FÜR INFORMATIK DER TECHNISCHEN UNIVERSITÄT MÜNCHEN Dissertation in Informatik An Actual Causality Framework for Accountable Systems Amjad Ibrahim FAKULTÄT FÜR INFORMATIK DER TECHNISCHEN UNIVERSITÄT MÜNCHEN Lehrstuhl IV - Software and Systems Engineering An Actual Causality Framework for Accountable Systems Amjad Ibrahim Vollständiger Abdruck der von der Fakultät für Informatik der Technischen Universität München zur Erlangung des akademischen Grades eines Doktors der Naturwissenschaften (Dr. rer. nat.) genehmigten Dissertation. Vorsitzender: Univ.-Prof. Dr. Helmut Seidl Prüfer der Dissertation: 1. Univ.-Prof. Dr. Alexander Pretschner 2. Univ.-Prof. Dr. Joseph Halpern, Cornell University, USA Die Dissertation wurde am 03.11.2020 bei der Technischen Universität München eingereicht und durch die Fakultät für Informatik am 04.03.2021 angenommen. Acknowledgments One day, we‘ll be what we want. Âd§r A ¾Aw§ wknF The journey hadn’t yet begun, the road hadn’t ended. Yht Cd ¯¤ , dt  Tlr ¯ The wise men hadn’t yet reached their exile. uhatr ºAmk l"ba§ The exiled men hadn’t yet attained hatmk ºAr ul"ba§ Am their wisdom. Mural. Unfortunately, It Was Paradise: Selected Poems, (2000) L§¤C wmm T§Cd by Mahmoud Darwish et al., University of California Press. Throughout my life, I always come back to this verse as I close chapters, “did I attain my wisdom? Was it the journey” I still do not know. However, I know that this particular adventure was unique; it was very challenging, still rewarding in many ways. It made me a new person in many aspects, close to what I want to be; more humble, precise, critical, resilient and persistent. I owe my resilience and persistence to my late mother; to her, goes my utmost gratitude. Mom: you once joked, “a doctorate title goes well with your name, Amjad!” I like to think that this step would make you proud and happy; I did it for you, mom; and my father, who took part in shaping this thesis through our long conversations on the topic and around it. My deepest gratitude goes to my supervisor, Prof. Dr. Alexander Pretschner. Alex, it is not a coincidence that you have been my teacher for very long. From the very first lecture I attended with you in 2013 until now, I am learning from you. Thank you for preparing me for this, granting me the chance, challenging me, guiding me, and having my back every time things did not work as expected. Without all our discussions, I certainly would not have been writing this part of this thesis. Prof. Joseph Halpern, this whole thesis is an acknowledgment of the work you have done. I would also like to express my gratefulness for your insightful comments and support over the past three years. Thank you very much for your warm hospitality in Ithaca. Along this journey, I met a diverse and friendly group at the Chair of Software and Systems Engineering. To all my friends at the chair, thank you for sharing pain, tiredness, laughs, food, ice cream, beer, and wine with me. I am grateful to the Bachelor and Master’s students who helped in building parts of this thesis, especially Simon, Tobias, Stavros, and others. I am also grateful to Severin and Claudius for proofreading parts of this thesis. Finally, a heartfelt thanks to my dear family in “the lady of earth, the mother of all beginnings, the mother of all endings,” Palestine; my father, sisters, and little nephews, you were always a part of this adventure. To my bigger family of friends in Palestine and Germany and especially those in the intersection of the two circles, thanks for your support. v Zusammenfassung Mit dem stetigen Einzug von digitalen Systemen in alle Facetten des täglichen Lebens wächst die Notwendigkeit deren Handeln erklärbar zu machen. Da moderne Systeme Personen schaden, Assets kompromittieren oder über Kreditwürdigkeit entscheiden kön- nen, müssen sie accountable sein. Accountability eines Systems zu ermöglichen im Sinne der Entwicklung von forensischen Fähigkeiten, um Unfälle zu erklären und um mögliche fehlverhaltende Parteien verantwortlich zu machen, ist einer der Säulen dieser Doktorar- beit. Die drei Konzepte — Accountability, Erklärbarkeit, Verantwortlichkeit — basieren auf Kausalität; sie benötigen ein passendes Verständnis von Kausalität. Actual Causality, ein Konstrukt das seine Wurzeln in der Philosophie der Wissenschaft hat, ist formalisiert in Halpern und Pearls Kausalitätstheorie. Allerdings wurde — soweit uns bekannt — die Theorie und Operationalisierung der Actual Causality nicht explizit in automatisierter Form für soziotechnische Zwecke wie Accountability verwendet. Stattdessen wurden sie nur adaptiert und verwendet im Zusammenhang mit domänenspezifischen Konzepten wie der Lineage einer Databankanfrage. Dies ist eine Einschränkung, die teilweise auf die Berechnungskomplexität von Kausalität zurückzuführen ist. Obwohl Actual Causality in verschiedenen Disziplinen angewandt wird, fehlt trotzdem eine Methodik, um eine effiziente automatisierte Unterstützung zu entwickeln, welche Accountability moderner Systeme ermöglicht trotz ihrer Komplexität. In dieser Dissertation entwickeln wir ein umfassendes Framework, automatisiert durch eine Toolchain, um das Problem der Operationalisierung von Tatsächlicher Kausalität für Zwecke der Accountability zu adressieren. Das Framework fokussiert auf die Effizienz und Skalierbarkeit verschiedener Varianten automatisierter Kausalitätsschlussfolgerungen, sowie die Effektivität der Schlussfolgerungen durch praktische und domänenspezifische Ansätze für kausale Modellierung und Kontextualisierung. Da Wiederverwendung geför- dert wird, werden Schwierigkeiten bei der Einbettung von Kausalitätsschlussfolgerungen in neue Domänen reduziert. Auf der einen Seite wird eine Reihe an generellen Algorithmen beigetragen um das Schlussfolgern zu automatisieren, was die Wiederverwendung über mehrere Domänen ermöglicht, auf der anderen Seite wird die Wiederverwendung von domänenspezifischen Methodiken und Wissensquellen unterstützt um Modellierung und Kontextualisierung zu operationalisieren. Das führt dazu, dass der Fokus stärker auf die In- tegration dieser Lösungen zur Ermöglichung von Accountability in spezifischen Systemen wechselt, welche auch durch unser Framework adressiert werden, da es zur Lösung von Accountability-bezogene Probleme für eine diverse Menge von Systemen genutzt werden kann. Wir demonstrieren, wie man mit dem Framework als Ganzem interagiert, indem wir uns auf Fallbeispiele aus dem Bereich der Microservice-basierten Systeme, sowie auf Beispiele von Flugzeugunglücken, Versagen von cyber-physikalischen Systemen und Systemen der künstlichen Intelligenz konzentrieren, da wir überzeugt sind, dass diese Systeme reprä- sentativ für moderne, komplexe Systeme sind. Weiterhin zeigen wir, dass das Framework vii generisch genug ist um Accountability und verwandte Konzepte wie Responsibility einzu- binden und dass es mit Toolunterstützung in verschiedene Domänen eingebunden werden kann. Des Weiteren präsentieren wir verschiedene Experimente um darzulegen, dass unsere Ansätze für Kausalitätsschlussfolgerungen effizienter und allgemeingültiger sind als andere aktuelle Techniken. viii Abstract With the rapid deployment of digital systems into all aspects of daily life, the need to understand their actions grows. As modern systems might harm people, compromise assets, or decide loan adequacy, they ought to be accountable. Enabling accountability of a system in the sense of developing its forensic capabilities to explain mishaps and possibly hold misbehaving parties responsible for violations, is the pillar of this doctoral thesis. The three concepts —accountability, explanation, and responsibility— are inherently causal; they require a notion of causality to enable them. Actual causality, a construct rooted in the philosophy of science, is well formalized within the Halpern and Pearl theory of causality. However, to the best of our knowledge, explicit actual causality theories and operationalizations have not been utilized, in an automated fashion, to enable socio- technical purposes such as accountability. Instead, they are adapted and used only in correspondence with domain-specific concepts such as a lineage of a database query. A restriction that is partially attributed to the computational complexity of causality. While used across different disciplines, actual causality still lacks a methodology to devise efficient automated assistance that enables modern systems’ accountability, given their complexity. In this thesis, we develop a unifying framework, automated by a set of tools, to address the problem of operationalizing actual causality for purposes related to accountability. The framework tackles the efficiency, and scalability of different notions of automated causal reasoning. Also, it considers the effectiveness of this reasoning through practical domain-specific approaches to causal modeling and contextualization. Thus, the frame- work diminishes the barrier to embedding causality reasoning in new domains because it promotes reuse. On the one hand, it contributes a set of general algorithms to automate reasoning so that it can be reused among different domains. It supports, on the other hand, the reuse of domain-specific methodologies and knowledge sources to operationalize modeling and contextualization. As a result, the focus then shifts to how all these solutions are integrated to serve the goal of enabling accountability in a specific system. To that end, our framework can be used to solve accountability-based problems for a wide range of systems.
Details
-
File Typepdf
-
Upload Time-
-
Content LanguagesEnglish
-
Upload UserAnonymous/Not logged-in
-
File Pages229 Page
-
File Size-