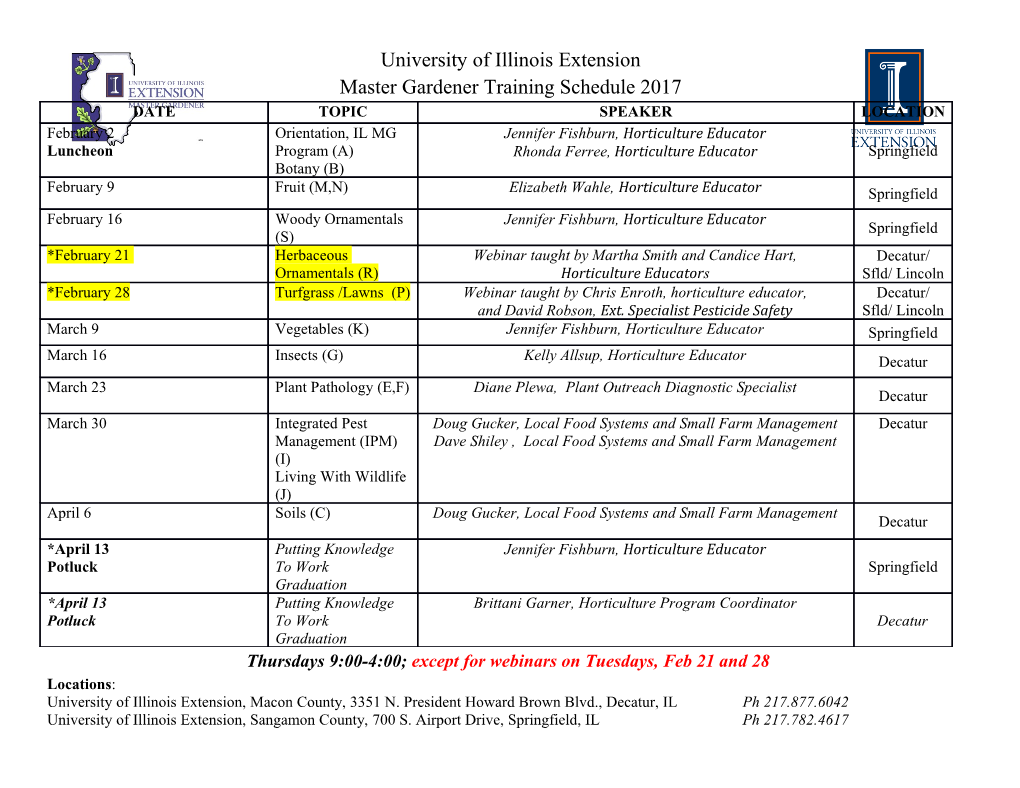
Jan Sprenger Stephan Hartmann Bayesian Philosophy of Science Variations on a Theme by the Reverend Thomas Bayes p(EjH) p(HjE) = p(H) p(E) Ich bin ein Esel, und will getreu, wie meine Väter, die Alten, an der alten, lieben Eselei, am Eseltume halten. Und weil ich ein Esel, so rat ich euch, den Esel zum König zu wählen; wir stiften das große Eselreich, wo nur die Esel befehlen. Wir sind alle Esel! I-A! I-A! Wir sind keine Pferdeknechte. Fort mit den Rossen! Es lebe—Hurra!— Der König vom Eselsgeschlechte! Heinrich Heine Contents Theme: Bayesian Philosophy of Science 1 Variation 1: Learning Conditional Information 31 1.1 The Kullback-Leibler Divergence and Probabilistic Updating 33 1.2 Three Challenges for Minimizing Divergence . 38 1.3 Meeting the Challenges . 40 1.4 Discussion . 48 1.5 Proofs of the Theorems . 51 Variation 2: Confirmation 57 2.1 Motivating Bayesian Confirmation Theory . 58 2.2 Confirmation as (Increase in) Firmness . 59 2.3 The Plurality of Bayesian Confirmation Measures . 69 2.4 Discussion . 73 Variation 3: The Problem of Old Evidence 77 3.1 The Dynamic POE: The GJN Approach . 79 3.2 Solving the Dynamic POE: Alternative Explanations . 83 3.3 The Static POE: A Counterfactual Perspective . 86 3.4 Solving the Hybrid POE: Learning Explanatory Relationships 88 3.5 Discussion . 92 3.6 Proofs of the Theorems . 96 Variation 4: The No Alternatives Argument 101 4.1 Modeling the No Alternatives Argument . 102 4.2 Results and Discussion . 107 4.3 Proof of the Theorems . 111 v vi Contents Variation 5: Scientific Realism and the No Miracles Argument 115 5.1 The Probabilistic No Miracles Argument . 116 5.2 Extending the No Miracles Argument to Stable Scientific Theories . 122 5.3 The Frequency-Based No Miracles Argument . 129 5.4 Discussion . 133 5.5 Proofs of the Theorems . 136 Variation 6: Causal Effect 141 6.1 The Framework: Causal Bayes Nets . 143 6.2 General Adequacy Conditions . 147 6.3 Causal Production and the Suppes-Pearl Measure . 150 6.4 The Multiplicativity Principle and the Difference Measure . 152 6.5 The No Dilution for Irrelevant Effects Principle and Proba- bility Ratio Measures . 154 6.6 Conjunctive Closure and the Logarithmic Ratio Measure . 157 6.7 Application: Quantifying Causal Effect in Medicine . 158 6.8 Discussion . 159 6.9 Proofs of the Theorems . 163 Variation 7: Explanatory Power 171 7.1 Toward a Statistical Relevance Account of Explanatory Power 173 7.2 Explicating Explanatory Power . 177 7.3 Discussion . 184 Variation 8: Intertheoretic Reduction 187 8.1 The Generalized Nagel-Schaffner Model . 188 8.2 Reduction and Confirmation . 191 8.3 Why Accept a Purported Reduction? . 197 8.4 Discussion . 199 8.5 Proofs of the Theorems . 201 Variation 9: Hypothesis Testing and Corroboration 205 9.1 Confirmation versus Corroboration . 209 9.2 Popper’s Measure of Degree of Corroboration . 212 9.3 The Impossibility Results . 216 9.4 Toward a New Explication of Corroboration . 223 9.5 Discussion . 227 Contents vii 9.6 Proofs of the Theorems . 230 Variation 10: Simplicity and Model Selection 237 10.1 Simplicity in Model Selection . 238 10.2 Curve Fitting and Estimation Error . 240 10.3 The Akaike Information Criterion (AIC) . 244 10.4 The Bayesian Information Criterion (BIC) . 247 10.5 Discussion . 251 10.6 Sketch of the Derivation of Akaike’s Information Criterion . 254 Variation 11: Scientific Objectivity 257 11.1 Forms of Scientific Objectivity . 258 11.2 Challenge 1: The Choice of the Prior Distribution . 260 11.3 Challenge 2: Belief vs. Evidence . 263 11.4 Challenge 3: Neglect of Experimental Design . 266 11.5 Discussion: A Digression on Scientific Objectivity . 270 The Theme Revisited 275 Bibliography 287 List of Figures 321 List of Tables 323 viii Theme: Bayesian Philosophy of Science Among the greatest achievements of science are the laws, models and the- ories it has came up with. Newton’s laws of mechanics, Bohr’s model of the atom and Einstein’s Theory of Relativity gave us unprecedented insights into the nature of physical reality. Similarly, Mendel’s laws of inheritance, Darwin’s theory of natural selection, and Crick and Watson’s innovations in molecular biology elucidated how species develop and how traits and properties are passed on from one generation to the next. Pi- oneers of rational choice theory like Von Neumann, Savage and Arrow explained behavior in terms of beliefs and preferences and came up with powerful axioms for rational decision-making under uncertainty. This enumeration does not intend to depreciate the value of experi- mental work in science. Rather, we would like to motivate why theories and models are central for understanding phenomena, predicting future events and transferring scientific knowledge to other domains. Therefore the assessment of scientific theories and models is a central part of sci- entific reasoning. This book investigates how Bayesian inference can contribute to this goal. While we do not want to claim that scientific reasoning is essentially Bayesian, we claim that Bayesian models can elucidate diverse aspects of scientific reasoning, increasing our understanding of how science works and why it is so successful. The book is written a cycle of variations on this theme; it applies Bayesian inference to eleven different aspects of scientific reasoning. In this introduction, we explain the constitutive principles and philo- sophical foundations of Bayesian inference, as well as some particular rea- 1 2 Contents soning techniques (e.g., Bayesian networks) that we need in the remainder of the book. Then we describe our methodological approach—Bayesian philosophy of science—in slightly more detail. The level is introductory; no knowledge of calculus or higher mathematics is required. The Static Dimension of Bayesian Inference: Probability and Degrees of Belief In science as well as in ordinary life, we routinely make a distinction be- tween more and less credible hypotheses. Consider, for example, the ques- tion which nation will win the 2016 European Football Cup. We may con- sider Albania a very unlikely candidate, England not completely implausi- ble, and we may find it likely that the winner will be either France or Ger- many. This example illustrates that the epistemic standing of a hypothesis is no all-or-nothing affair, but a matter of gradation. Here the Bayesians step in: they use the concept of degree of belief to describe epistemic at- titudes about uncertain propositions, and they represent these degrees of belief by a particular mathematical structure: probability functions. These two modeling assumptions are the central elements of Bayesian inference. In other words, Bayesians regard probabilities as expressions of subjective uncertainty—an interpretation that goes back to the English mathemati- cian, reverend and philosopher Thomas Bayes (1701–1761) (Bayes, 1763). The probability calculus has a long history as a tool for handling sub- jective uncertainty. In particular, it is one of the dominant paradigms in the psychology of human reasoning (e.g., Oaksford and Chater, 2000). Other scientific applications abound, such as Bayesian inference in phylogenet- ics, Bayesian interpretations of quantum mechanics, statistical inference and causal induction (e.g., Bernardo and Smith, 1994; Spirtes et al., 2000). Bayesian reasoning is also widely applied in philosophy: it is a standard tool in various branches of epistemology (e.g., Bovens and Hartmann, 2003; Pettigrew, 2015) and in the foundations of decision theory and ratio- nal choice (e.g., Jeffrey, 1971; Savage, 1972). The prominence of Bayesian inference in established scientific and philosophical theories recommends it as the default model for modeling uncertain reasoning. We do not claim that it is on foundational grounds superior to alternatives such as rank- ing functions (Spohn, 2012) or Dempster-Shafer theory (Shafer, 1976): we only claim that the past successes and the wide scope of Bayesian mod- Contents 3 els recommend them as an excellent tool for studying scientific reasoning. Practical considerations, such as the simplicity of the probability axioms and the existence of a well-developed mathematical theory underneath them, support the case for Bayesian philosophy of science, too. The distinctive feature of Bayesian inference is the central role of degree of belief. More traditional descriptions of epistemic attitudes, e.g., theories that just distinguish between belief, disbelief and suspension of judgment, struggle to adequately describe graded epistemic attitudes. This fails to account for many cases of scientific reasoning where we do hold graded beliefs. But psychological realism is not the only force that pulls into the direction of a graded theory of epistemic attitudes. An all-or-nothing account of epistemic attitudes also leads into philosophical trouble, such as in the famous lottery paradox (Kyburg, 1961). For instance, if just one out of 1.000.000 tickets in a lottery is winning, then for each single ticket #i (i 2 f1, 2, . , 1.000.000g), we seem to be entitled to believe that it is not the winning one. However, we also believe that there is a winning ticket in the lottery. Hence, the set of the propositions that we believe (“There is a winning ticket”, “ticket #1 does not win”, “ticket #2 does not win”, etc.) is inconsistent, which is at least prima facie an undesirable consequence. For theories of graded belief, no such inconsistency arises (for discussion of the full vs. graded belief relationship, see Leitgeb, 2014; Fitelson et al., 2016). If we want to use the concept of degree of belief to describe which scientific theories are more credible than others, we have to say something about the rules that rational degrees of belief have to satisfy. After all, the objects of degrees of belief—propositions—are related to each other in manifold ways, and these relations constrain the set of degrees of belief that we can rationally entertain.
Details
-
File Typepdf
-
Upload Time-
-
Content LanguagesEnglish
-
Upload UserAnonymous/Not logged-in
-
File Pages331 Page
-
File Size-