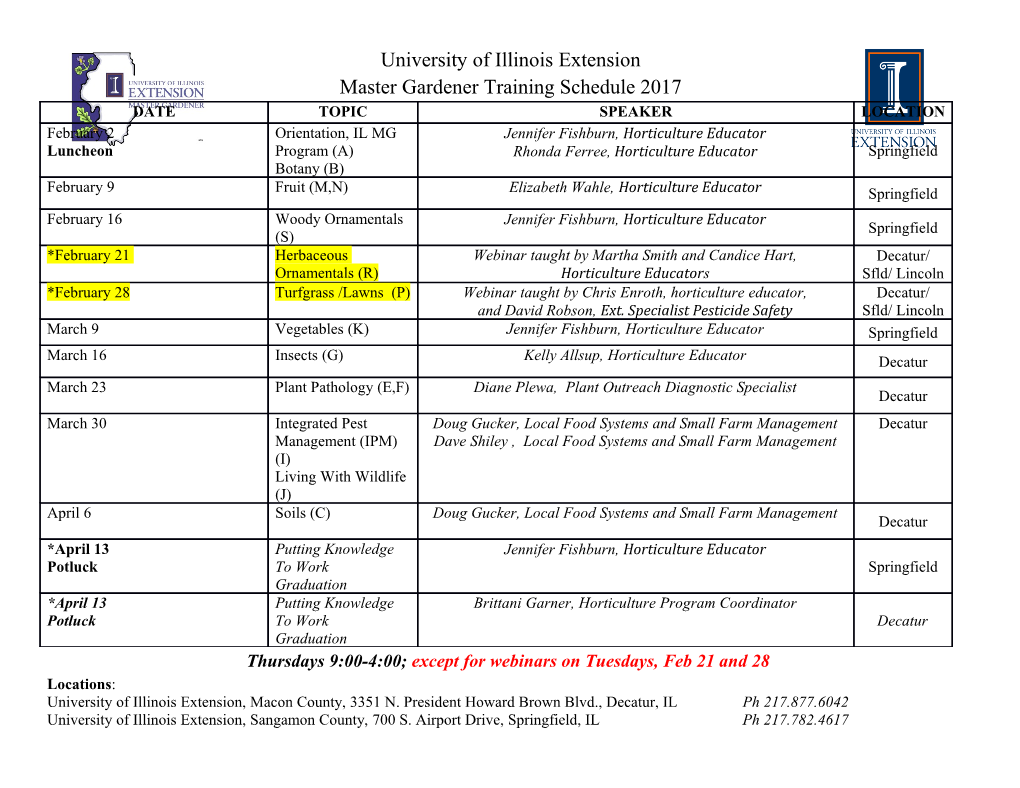
Research Skills for Psychology Majors: Everything You Need to Know to Get Started Analysis of Variance: General Concepts This chapter is designed to present the most basic ideas in analysis of variance in a non-statistical manner. Its intent is to communicate the general idea of the analysis and provide enough information to begin to read research result sections that report ANOVA analyses. Analysis of Variance is a general-purpose statistical procedure that is used to anal- ize a wide range of research designs and to investigate many complex problems. In this chapter we will only discuss the original, basic use of ANOVA: analysis of experiments that include more than two groups. When ANOVA is used in this simple sense, it follows directly from a still simpler procedure, the t-test. A Deeper Truth The t-test compares two groups, either in a between-subjects design (different subjects in the groups) or a repeated-measures design (same subjects assessed Actually, the t-test is a twice). ANOVA can be thought of as an extension of the t-test to situations in special case of ANOVA. ANOVA is the real thing. which there are more than two groups (one-way design) or where there is more than one independent variable (factorial design). These situations are the most common in research, so ANOVA is used far more frequently than t-tests. A Still Deeper Truth Variance is Analyzed Actually, ANOVA is a sim- The name “analysis of variance” is more representative of what the analysis is plification of very complex about than “t-test” because we are in fact focusing on analyzing variances. The correlations. Correlation is conceptual model for ANOVA follows the familiar pattern first introduced in the real thing. the Inferential Statistics chapter: a ratio is formed between the differences in the means of the groups and the error variance. In the same way that a variance (or standard deviation) can be calculated from a set of data, a variance can be calcu- lated from a set of means. So the differences among the means is thought of as their variance: higher variance among the means indicates that there are more differences (which is good, right?). The variance among the group means is called the between-groups variance. The ratio, then, is between-groups variance divided by error variance. A larger ratio indicates that the differences between the groups are greater than the error or “noise” going on inside the groups. If this ratio, the F statistic, is large enough given the size of the sample, we can reject the null hypothesis. The whole story in ANOVA is figuring out how to calculate (and understand) these two types of variance. ©2003 W. K. Gabrenya Jr. Version: 1.0 Page 2 A Visual Example Here is an example of a one-way, between-groups design that would be analyzed using ANOVA. Four groups of participants are randomly sampled from four ma- jors on campus. We will not identify the majors for the sake of interdepartmental harmony, but the identity of Group 4 is clear. Each sample includes five students. They are each administered the Wechsler Adult Intelligence Scale (WAIS-III) to obtain a measure if IQ. IQs have a mean of 100 in the population as a whole. Our question: which major is smarter? The following table presents the raw data (IQ scores), the means within each group, the standard deviation within each group, and the variance. The variance is simply the SD squared, a more useful number for certain aspects of the calcultions. It is normal that some of the SDs are larger than others. The gray bars below the scale represent the range of the IQs in each major, which is one indication of the within-group variability. (A wider range often produces a higher SD.) In the last column, the mean of the means (grand mean), the standard deviation of the means, and the variance of the means are presented. Group 1 Group 2 Group 3 Group 4 Grand Mean Data: 80, 85, 90, 90, 93, 96, 97, 100, 103, 105, 110, 115, (the group means 95, 100 99, 102 106, 109 120, 125 could go here) Mean: 90.0 96.0 103.0 115.0 101.0 Std. Dev.: 7.9 4.7 4.7 7.9 Variance: 62.5 22.5 22.5 62.5 Grand Mean 80 90 100 110 120 125 1 1 1 Gp 1 1 1 Group 4 Group 2 Group 3 What’s the null hypothesis? The null condition is that there is no difference be- tween the population means: H0: µ1 = µ2 = µ3 = µ4, where µ is “mu,” the population mean. Our task is to determine if the sample means, presented in the table above, are sufficiently different from each other compared to the error variance within the groups, to reject the null hypothesis. Of course the sample size will also affect the outcome because larger samples allow for better tests of the null hypothesis. In the language of ANOVA, we will look at the ratio of the between-group variance to the within-group (error) variance. Between-groups variance F = Error Variance Within Groups Page 3 In the example, we have included the Calculating the Variances individual data for group 1 as circles inside the group 1 gray bar. The SD of group Within-Groups (Error) Variance: 1, 7.9, is computed from these 5 values. The overall amount of error variance is the combined variances of the Recall that the SD is the variability of the four groups. Combining the variances from several groups together is individual data based on how distant each called pooling, so the resulting combined variance is termed the pooled one is from the group mean (90.0). In variance. Averaging the variances in this study produces a pooled error other words, it is a measure of the extent variance of 6.522 =42.5. to which the five students sampled for that Between-Groups Variance: major are not exactly of the same intel- ligence. The student in group 2 are more Calculation of the between-groups variance is not as intuitive as the similar to each other and produce a SD whithin-groups variance. Conceptually, it seems that the SD of the four of 4.7. The overall error variance for the group means would be a good measure. (The SD of the means is 10.7.) sample is computed by combining these However, the actual between-groups SD is 24.0, so the between-groups 2 four SDs (see sidebar). variance is 24 = 577. The between-groups variability is com- puted in the same way, but we look at how much the group means vary from the grand mean (the mean of the means). The higher this variability, the more the means differ from each other and the more the null hyothesis looks “rejectable.” (See sidebar.) Finally, the ANOVA ANOVA Source Table The ANOVA focuses on the ratio of the 1 2 3 4 5 6 between group variance to the within- Sum of df Mean F Sig. groups variance. SPSS produces an Squares Square ANOVA source table to report the result Between 1730.000 3 576.667 13.569 .0001 of the analysis.This table is called a source Groups table because it identifies the sources of Within 680.000 16 42.500 variability in the data. As explained above, Groups there are two kinds of variability, vari- Total 2410.000 19 ability between group means, and variabil- ity within groups (error variance). The source table provides information about these two sources. The column numbers Degrees of Freedom in ANOVA have been added for our use. All statistics, such as F, t, and chi-square, are evaluated in the context of the sample size: larger samples allow lower statistical values to reach the Column 3, reflecting the number of groups magic .05 level of confidence. The sample size is expressed in terms of and the sample size, is discussed in the degrees of freedom (df). Your statistics class has more to say about df. In sidebar. Column 4 presents the variance a t-test, the df is the sample size minus 2 (N-2). In ANOVA, we use two associated with the mean differences df values. The df-error is based on the sample size: (between groups) and within-group er- df = ∑(n -1), where n is the size of each of the group samples ror. These numbers are discussed in the e g g Variances sidebar. Column 5 is the ratio of 16 = (5-1) + (5-1) + (5-1) + (5-1) these two values, the F statistic. Column 6 presents the p-value (see Inferential Statis- ANOVA also requires a df for the number of groups: tics chapter) of the F statistic based on the dfbg = g-1, where g is the number of groups sample size. Because our normal criterion for rejecting the null hypothesis is p<.05, The F statistic is always presented along with these df values, e.g., this p value is very good (good = low), and F(3,19)=13.6, p<.0001. Page 4 we can reject the null hypothesis. What has been rejected? By rejecting the null hypothesis, we conclude that the four means are not equal in the population, that is, all majors are not created equal. However, what it does not tell us is exactly which major is smarter than which other major. Is Group 4 smarter than Group 2, or just smarter than the hapless Group 1? Just eyeballing the means is not good enough: we need to know if the differences between particular pairs of means are really significantly different.
Details
-
File Typepdf
-
Upload Time-
-
Content LanguagesEnglish
-
Upload UserAnonymous/Not logged-in
-
File Pages8 Page
-
File Size-