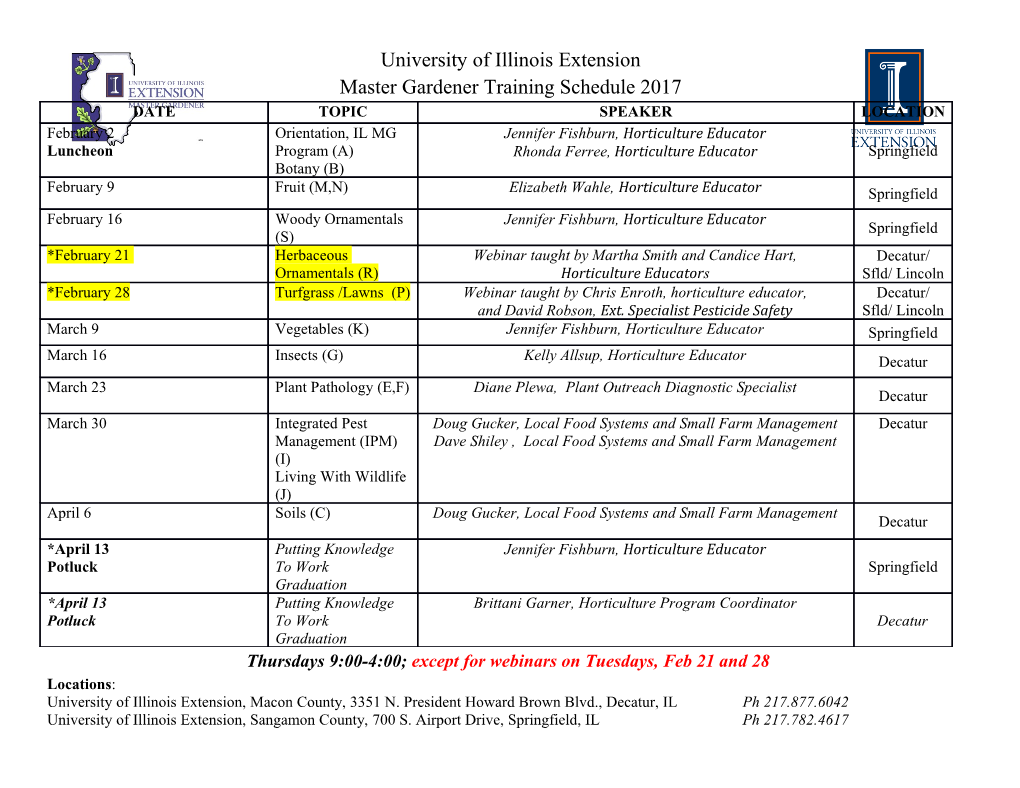
Graphical Models Zoubin Ghahramani University of Cambridge, UK Uber AI Labs, San Francisco, USA [email protected] http://learning.eng.cam.ac.uk/zoubin/ MLSS 2017 T¨ubingen Representing knowledge through graphical models A B C D E • Nodes correspond to random variables • Edges represent statistical dependencies between the variables Why do we need graphical models? • Graphs are an intuitive way of representing and visualising the relationships between many variables. (Examples: family trees, electric circuit diagrams, neural networks) • A graph allows us to abstract out the conditional independence relationships between the variables from the details of their parametric forms. Thus we can answer questions like: \Is A dependent on B given that we know the value of C?" just by looking at the graph. • Graphical models allow us to define general message-passing algorithms that implement probabilistic inference efficiently. Thus we can answer queries like \What is p(AjC = c)?" without enumerating all settings of all variables in the model. Graphical models = statistics × graph theory × computer science. Directed Acyclic Graphical Models (Bayesian Networks) A B C D A DAG Model / Bayesian network1 corresponds to a factorization of the joint probability distribution: E p(A; B; C; D; E) = p(A)p(B)p(CjA; B)p(DjB; C)p(EjC; D) In general: n Y p(X1;:::;Xn) = p(XijXpa(i)) i=1 where pa(i) are the parents of node i. 1\Bayesian networks" can and often are learned using non-Bayesian (i.e. frequentist) methods; Bayesian networks (i.e. DAGs) do not require parameter or structure learning using Bayesian methods. Also called \belief networks". Directed Acyclic Graphical Models (Bayesian Networks) A B Semantics: X??Y jV if V d-separatesCX from Y Definition: V d-separates X from Y if every undirected path2 between X and Y is blocked by V. A path is blocked by V if there is aD node W on the path such that either: E 1. W has converging arrows along the path (! W )3 and neither W nor its descendants are observed (in V), or 2. W does not have converging arrows along the path (! W ! or W !) and W is observed (W 2 V). Corollary: Markov Boundary for X: fparents(X) [ children(X) [ parents-of-children(X)g. 2An undirected path ignores the direction of the edges. 3Note that converging arrows along the path only refers to what happens on that path. Also called a collider. Directed Graphs for Statistical Models: Plate Notation Consider the following simple model. A data set of N points is generated i.i.d. from a Gaussian with mean µ and standard deviation σ: N Y p(x1; : : : ; xN ; µ, σ) = p(µ)p(σ) p(xnjµ, σ) n=1 This can be represented graphically as follows: μ σ μ σ ≡ xn x1 x2 ... xN N Inference in a graphical model A B Consider the following graph: which represents: p(A; B; C; D; E) = p(A)p(B)p(CjA; B)p(DjB; C)p(EjC; D) C Inference: evaluate the probability distribution over some set of variables, given the values of another set of variables. D For example, how can we computeE p(AjC = c)? Assume each variable is binary. Naive method: X p(A; C = c) = p(A; B; C = c; D; E) [16 terms] B;D;E X p(C = c) = p(A; C = c) [2 terms] A p(A; C = c) p(AjC = c) = [2 terms] p(C = c) Total: 16+2+2 = 20 terms have to be computed and summed Inference in a graphical model A B Consider the following graph: which represents: p(A; B; C; D; E) = p(A)p(BC)p(CjA; B)p(DjB; C)p(EjC; D) Computing p(AjC = c). D More efficient method: E X p(A; C = c) = p(A)p(B)p(C = cjA; B)p(DjB; C = c)p(EjC = c; D) B;D;E X X X = p(A)p(B)p(C = cjA; B) p(DjB; C = c) p(EjC = c; D) B D E X = p(A)p(B)p(C = cjA; B) [4 terms] B Total: 4+2+2 = 8 terms Belief propagation methods use the conditional independence relationships in a graph to do efficient inference (for singly connected graphs, exponential gains in efficiency!). Factor graph propagation Algorithmically and implementationally, it's often easier to convert directed and undirected graphs into factor graphs, and run factor graph propagation. p(x) = p(x1)p(x2jx1)p(x3jx2)p(x4jx2) ≡ f1(x1; x2)f2(x2; x3)f3(x2; x4) Singly connected vs Multiply connected factor graphs: x3 x3 f 2 f2 f4 f 1 x f1 2 x2 x1 f x4 x x 3 1 f3 4 Factor Graphs In a factor graph, the joint probability distribution is written as a product of factors. Consider a vector of variables x = (x1; : : : ; xn) 1 Y p(x) = p(x ; : : : ; x ) = f (x ) 1 n Z j Sj j where Z is the normalisation constant, Sj denotes the subset of f1; : : : ; ng which participate in factor fj and xSj = fxi : i 2 Sjg. x3 f2 f4 f1 x2 x1 f x4 variables nodes: we draw open circles for each variable xi in the distribution.3 factor nodes: we draw filled dots for each factor fj in the distribution. Propagation in Factor Graphs Let n(x) denote the set of factor nodes that are neighbors of x. Let n(f) denote the set of variable nodes that are neighbors of f. We can compute probabilities in a factor graph by propagating messages from variable nodes to factor nodes and viceversa. message from variable x to factor f: Y µx!f (x) = µh!x(x) h2n(x)nffg message from factor f to variable x: 0 1 X Y µf!x(x) = @f(x) µy!f (y)A xnx y2n(f)nfxg P where x are the variables that factor f depends on, and xnx is a sum over all variables neighboring factor f except x. Propagation in Factor Graphs n(x) denotes the set of factor nodes that are neighbors of x. n(f) denotes the set of variable nodes that are neighbors of f. message from variable x to factor f: Y µx!f (x) = µh!x(x) h2n(x)nffg message from factor f to variable x: 0 1 X Y µf!x(x) = @f(x) µy!f (y)A xnx y2n(f)nfxg If a variable has only one factor as a neighbor, it can initiate message propagation. Once a variable has received all messages from its neighboring factor nodes, one can compute the probability of that variable by multiplying all the messages and renormalising: Y p(x) / µh!x(x) h2n(x) Inference in Hidden markov models and Linear Gaussian state-space models ¢ ¡ X 1 X 2 X 3 X T ¢ ¡ Y1 Y2 Y 3 YT T Y p(X1;:::;T ;Y1;:::;T ) = p(X1)p(Y1jX1) [p(XtjXt−1)p(YtjXt)] t=2 • In HMMs, the states Xt are discrete. • In linear Gaussian SSMs, the states are real Gaussian vectors. • Both HMMs and SSMs can be represented as singly connected DAGs. • The forward{backward algorithm in hidden Markov models (HMMs), and the Kalman smoothing algorithm in SSMs are both instances of belief propagation / factor graph propagation. Software for Graphical Models • BUGS and WinBUGS: inference via Gibbs sampling, not very scalable • HUGIN: widely used, commercial, focus on exact inference • Kevin Murphy's Bayes Net Toolbox: Matlab, widely used • Microsoft's Infer.NET: advanced scalable libraries implementing factor graph propagation, EP, and variational message passing. • Jeff Bilmes' GMTK: very good at HMMs and related time series models • many others, see http://people.cs.ubc.ca/∼murphyk/Software/bnsoft.html • Much of this is subsumed by general Probabilistic Programming frameworks: e.g. Church, WebPPL, Turing, Anglican, Edward, STAN,... Summary • inference consists of the problem of computing p(variables of interestjobserved variables) • for singly connected graphs, belief propagation/ factor graph propagation solves this problem exactly. • well-known algorithms such as Kalman smoothing and forward-backward are special cases these general propagation algorithms. • for multiply connected graphs, the junction tree algorithm solves the exact inference problem, but can be very slow (exponential in the cardinality of the largest clique). • one approximate inference algorithm is \loopy belief propagation"|run propagation as if graph is simply connected; often works well in practice. Learning parameters x1 x2 θ2 x2 0.2 0.3 0.5 x x3 1 x4 0.1 0.6 0.3 p(x1)p(x2jx1)p(x3jx1)p(x4jx2) Assume each variable xi is discrete and can take on Ki values. The parameters of this model can be represented as 4 tables: θ1 has K1 entries, θ2 has K1 × K2 entries, etc. These are called conditional probability tables (CPTs) with the following semantics: 0 p(x1 = k) = θ1;k p(x2 = k jx1 = k) = θ2;k;k0 If node i has M parents, θi can be represented either as an M + 1 dimensional Q table, or as a 2-dimensional table with j2pa(i) Kj × Ki entries by collapsing all P the states of the parents of node i. Note that k0 θi;k;k0 = 1. (n) N Assume a data set D = fx gn=1. How do we learn θ from D? Learning parameters x 1 (n) N Assume a data set D = fx gn=1. How do we learn θ from D? x2 p(xjθ) = p(x1jθ1)p(x2jx1; θ2)p(x3jx1; θ3)p(x4jx2; θ4) x3 Likelihood: x N 4 Y p(Djθ) = p(x(n)jθ) n=1 Log Likelihood: N X X (n) (n) log p(Djθ) = log p(xi jxpa(i); θi) n=1 i This decomposes into sum of functions of θi.
Details
-
File Typepdf
-
Upload Time-
-
Content LanguagesEnglish
-
Upload UserAnonymous/Not logged-in
-
File Pages48 Page
-
File Size-