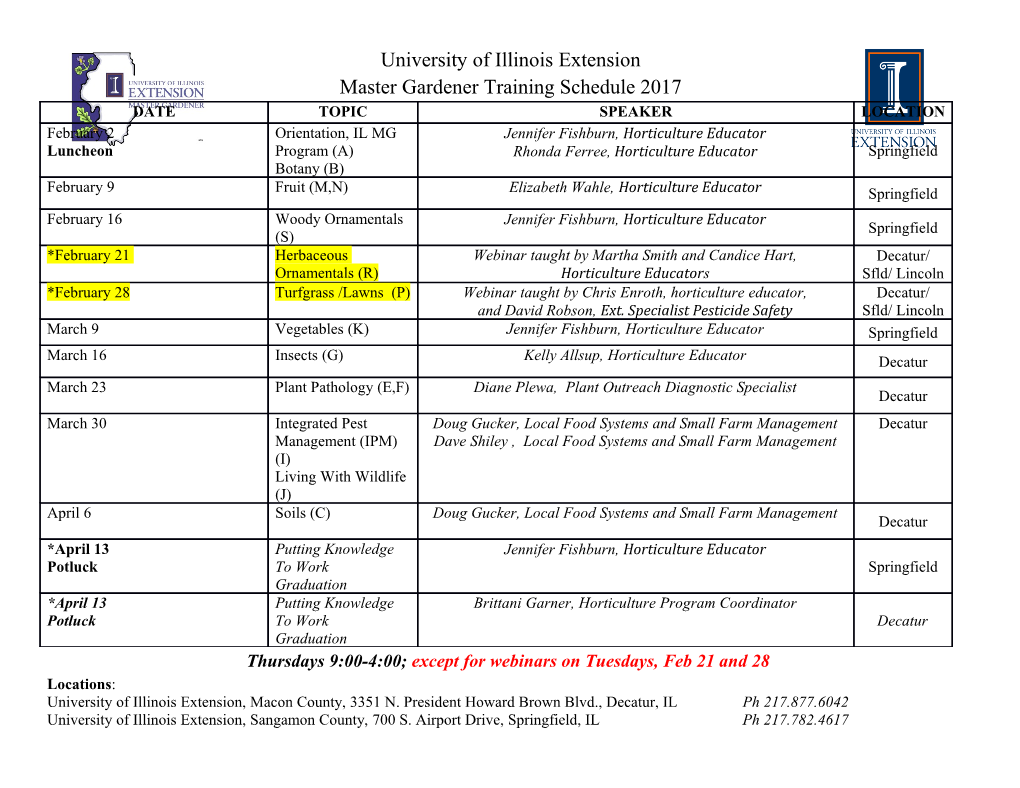
BINARY GOVERNANCE: LESSONS FROM THE GDPR’S APPROACH TO ALGORITHMIC ACCOUNTABILITY MARGOT E. KAMINSKI* Algorithms are now used to make significant decisions about individuals, from credit determinations to hiring and firing. But they are largely unregulated under U.S. law. A quickly growing literature has split on how to address algorithmic decision-making, with individual rights and accountability to nonexpert stakeholders and to the public at the crux of the debate. In this Article, I make the case for why both individual rights and public- and stakeholder-facing accountability are not just goods in and of themselves but crucial components of effective governance. Only individual rights can fully address dignitary and justificatory concerns behind calls for regulating algorithmic decision-making. And without some form of public and stakeholder accountability, collaborative public-private approaches to systemic governance of algorithms will fail. In this Article, I identify three categories of concern behind calls for regulating algorithmic decision-making: dignitary, justificatory, and instrumental. Dignitary concerns lead to proposals that we regulate *. Associate Professor of Law, Colorado Law School; Faculty Privacy Director at Silicon Flatirons; Affiliated Fellow, Information Society Project at Yale Law School; Faculty Fellow, Center for Democracy and Technology. Many thanks to Jef Ausloos, Jack Balkin, Michael Birnhack, Frederik Zuiderveen Borgesius, Bryan H. Choi, Kiel Brennan-Marquez, Giovanni Comandé, Eldar Haber, Irene Kamara, Derek H. Kiernan-Johnson, Kate Klonick, Mark Lemley, Gianclaudio Maglieri, Christina Mulligan, W. Nicholson Price, Andrew Selbst, Alicia Solow-Niederman, and Michael Veale for reading and for detailed comments. Thanks to the Fulbright-Schuman program, Institute for Information Law (“IViR”) at the University of Amsterdam, and Scuola Sant’Anna in Pisa for the time and resources for this project. Thanks to the faculty of Tel Aviv University, the Second Annual Junior Faculty Forum on the Intersection of Law and Science, Technology, Engineering, and Math (STEM) at the Northwestern Pritzker School of Law, and my own Colorado Law School faculty for excellent workshop opportunities. Extra thanks to Matthew Cushing, whose incredible support made this project possible, and to Mira Cushing for joy beyond words. 1529 1530 SOUTHERN CALIFORNIA LAW REVIEW [Vol. 92:1529 algorithms to protect human dignity and autonomy; justificatory concerns caution that we must assess the legitimacy of algorithmic reasoning; and instrumental concerns lead to calls for regulation to prevent consequent problems such as error and bias. No one regulatory approach can effectively address all three. I therefore propose a two-pronged approach to algorithmic governance: a system of individual due process rights combined with systemic regulation achieved through collaborative governance (the use of private-public partnerships). Only through this binary approach can we effectively address all three concerns raised by algorithmic decision- making, or decision-making by Artificial Intelligence (“AI”). The interplay between the two approaches will be complex. Sometimes the two systems will be complementary, and at other times, they will be in tension. The European Union’s (“EU’s”) General Data Protection Regulation (“GDPR”) is one such binary system. I explore the extensive collaborative governance aspects of the GDPR and how they interact with its individual rights regime. Understanding the GDPR in this way both illuminates its strengths and weaknesses and provides a model for how to construct a better governance regime for accountable algorithmic, or AI, decision-making. It shows, too, that in the absence of public and stakeholder accountability, individual rights can have a significant role to play in establishing the legitimacy of a collaborative regime. TABLE OF CONTENTS INTRODUCTION .................................................................................. 1531 I. WHY REGULATE ALGORITHMIC DECISION-MAKING? ......... 1537 II. THE BINARY APPROACH ............................................................. 1552 A. AN INDIVIDUAL RIGHTS REGIME ................................................... 1553 B. COLLABORATIVE GOVERNANCE .................................................... 1557 1. Collaborative Governance .......................................................... 1559 2. The Collaborative Governance Toolkit ...................................... 1564 3. The Collaborative Governance of Algorithms ........................... 1570 C. COMBINING THE TWO APPROACHES .............................................. 1577 1. The Two Systems as Complementary ........................................ 1578 2. The Two Systems in Tension ..................................................... 1580 III. THE TWO FACES OF THE GDPR ................................................ 1582 A. A PRIMER ON THE GDPR ............................................................... 1585 B. INDIVIDUAL RIGHTS ....................................................................... 1586 1. Notification and Access Rights .................................................. 1587 2. Other Checks on Data Processing .............................................. 1590 3. Individual Algorithmic “Due Process” Under Article 22 ........... 1592 C. COLLABORATIVE GOVERNANCE .................................................... 1595 2019] BINARY GOVERNANCE 1531 1. The GDPR as Collaborative Governance ................................... 1596 2. Formal Coregulation................................................................... 1599 3. Informal Collaborative Governance ........................................... 1601 4. The GDPR’s Accountability Problem ........................................ 1607 D. INTERACTION BETWEEN THE TWO PRONGS ................................... 1611 1. Where the Two Prongs Are Complementary ............................. 1611 2. Where the Two Prongs Are in Tension ...................................... 1613 CONCLUSION ...................................................................................... 1615 INTRODUCTION In 2011, fifth-grade teacher Sarah Wysocki was fired by a machine.1 Wysocki had been a star teacher, receiving rave reviews from both parents and her principal. But these positive reviews from humans were outweighed by a low score Wysocki received from an algorithm (a computer program), and the school district was required by policy to fire her, along with 205 other teachers. When Wysocki and others questioned this outcome and asked how the scores were calculated, they were told that the algorithm was too complicated for them to understand. Wysocki suspected, however, that the model relied heavily on standardized test scores and that due to cheating, over half of her students started the school year with artificially inflated exam scores. When she tried to make her case to the school district, however, she was told that the decision was final.2 Algorithms—that is, computer programs, including AI—are now used to make a host of decisions about human beings that have significant impacts on human welfare and dignity.3 Decisions about whether to extend credit, whether to hire or fire somebody, how to price discriminate, and what 1. CATHY O’NEIL, WEAPONS OF MATH DESTRUCTION 11 (2016); Bill Turque, ‘Creative . Motivating’ and Fired, WASH. POST (Mar. 6, 2012), https://www.washingtonpost.com/local/education/c reative--motivating-and-fired/2012/02/04/gIQAwzZpvR_story.html. 2. Recently, Houston-area teachers who were similarly fired based on the use of a secret computer algorithm successfully argued that their dismissals may have violated their procedural due process rights. Houston Federation of Teachers, Local 2415 v. Hous. Indep. Sch. Dist., 251 F. Supp. 3d 1168, 1180 (S.D. Tex. 2017). This ruling, however, applies only to government actions and to dismissals during the term of a contract or a continuing contract. Id. at 1173–74. Thanks to Mark MacCarthy for identifying this case. 3. For more extended descriptions and definitions of decision-making algorithms, see Jessica M. Eaglin, Constructing Recidivism Risk, 67 EMORY L.J. 59, 67–88 (2017) (discussing the actuarial algorithms used in recidivism risk assessment); David Lehr & Paul Ohm, Playing with the Data: What Legal Scholars Should Learn About Machine Learning, 51 U.C. DAVIS L. REV. 653, 658–62 (2017) (discussing machine-learning algorithms). 1532 SOUTHERN CALIFORNIA LAW REVIEW [Vol. 92:1529 educational track to put a student on now can all involve algorithmic decision-making, or a heavy reliance on suggestions arrived at by algorithms. These decisions, when made by the private sector, go largely unregulated in the United States.4 The types of algorithms used can present significant challenges to our legal system, as they are often secret, impossible to predict, hard to explain to nonexperts, and continuously changing over time. Earlier calls for algorithmic accountability propose addressing algorithmic decision-making through both individual “due process” rights and an array of systemic accountability measures.5 The systemic measures include public disclosure of source code, agency oversight, expert boards, and stakeholder input. More recent literature, however, moves away from these proposals, questioning both the value of individual rights and stakeholder input by nonexperts and the costs of public disclosure. Public- facing accountability and individual due process in particular
Details
-
File Typepdf
-
Upload Time-
-
Content LanguagesEnglish
-
Upload UserAnonymous/Not logged-in
-
File Pages88 Page
-
File Size-