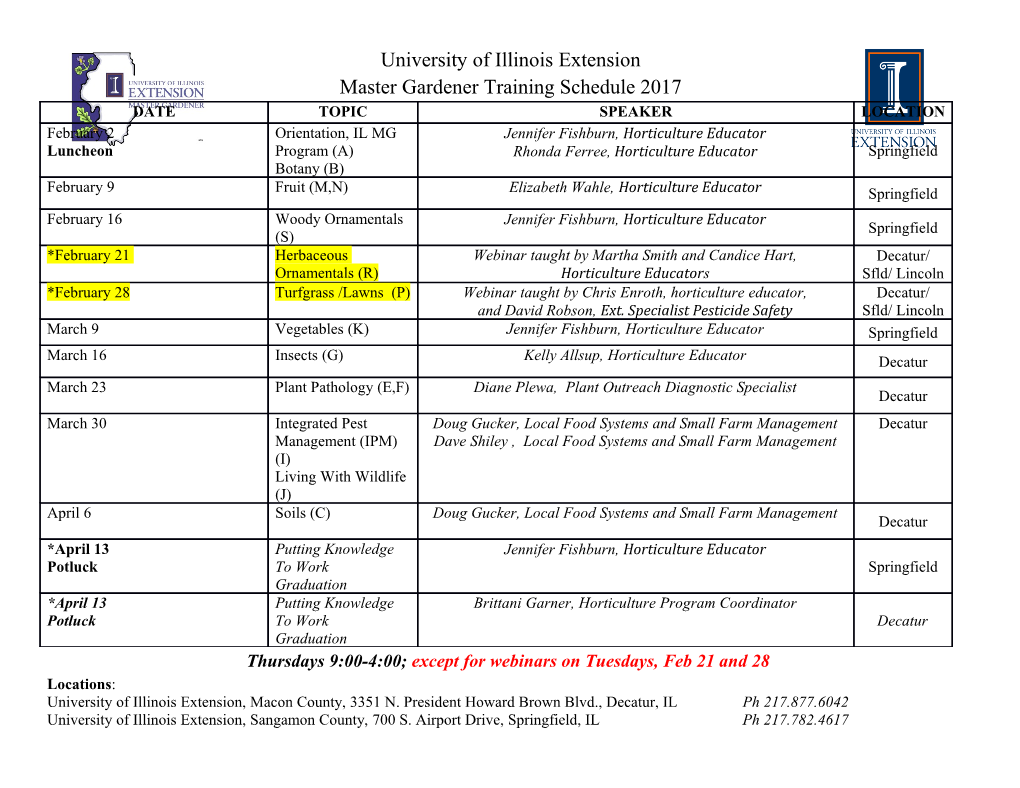
PDF hosted at the Radboud Repository of the Radboud University Nijmegen The following full text is a publisher's version. For additional information about this publication click this link. http://hdl.handle.net/2066/206297 Please be advised that this information was generated on 2021-10-08 and may be subject to change. M. U. DALMIŞ U. M. INVITATION AUTOMATED ANALYSIS to attend the public defense of my PhD thesis AUTOMATED ANALYSIS OF BREAST MRI BREAST OF ANALYSIS AUTOMATED OF BREAST MRI FROM TRADITIONAL METHODS AUTOMATED ANALYSIS INTO DEEP LEARNING OF BREAST MRI FROM TRADITIONAL METHODS INTO DEEP LEARNING Thursday 12 September 2019, at 12.30 in the Aula of the Radboud University, Comeniuslaan 2, 6525 HP Nijmegen. You are cordially invited to the reception after the defense ceremony PARANYMPHS FROM TRADITIONAL METHODS INTO DEEP LEARNING DEEP INTO METHODS TRADITIONAL FROM Jan-Jurre Mordang [email protected] Jules Jacobs [email protected] MEHMET UFUK DALMIŞ [email protected] MEHMET UFUK DALMIŞ Automated Analysis of Breast MRI: from Traditional Methods into Deep Learning Mehmet Ufuk Dalmı¸s This book was typeset by the author using LATEX2". Book by: Mehmet Ufuk Dalmı¸s Cover design by: Wendy Schoneveld & Mehmet Ufuk Dalmı¸s The research described in this thesis was carried out at the Diagnostic Image Analysis Group, Radboud University Medical Center (Nijmegen, the Netherlands). This work was supported by the European Union's Seventh Framework Programme for research, technological development and demonstration (grant agreement no. 601040). Financial support for publication of this thesis was kindly provided by Radboud Uni- versity Medical Center. ISBN 978-90-9032014-4 Printed by ProefschriftMaken.nl. Copyright ⃝c 2019 by Mehmet Ufuk Dalmı¸s. All rights reserved. No part of this publication may be reproduced or transmitted in any form or by any means, electronic or mechanical, including photocopy, recording, or any information storage and retrieval system, without permission in writing from the author. Automated Analysis of Breast MRI: from Traditional Methods into Deep Learning Proefschrift ter verkrijging van de graad van doctor aan de Radboud Universiteit Nijmegen op gezag van de rector magnificus prof. dr. J.H.J.M. van Krieken, volgens besluit van het college van decanen in het openbaar te verdedigen op donderdag 12 September 2019 om 12.30 uur precies door Mehmet Ufuk Dalmı¸s geboren op 6 May 1982 te Hınıs, Turkije Promotor: Prof. dr. N. Karssemeijer Copromotoren: Dr. R. M. Mann Dr. A. Gubern-M´erida Dr. J. Teuwen Manuscriptcommissie: Prof. dr. E. Marchiori Prof. dr. C. van Gils University Medical Center Utrecht Dr. K.G.A. Gilhuijs University Medical Center Utrecht Automated Analysis of Breast MRI: from Traditional Methods into Deep Learning Doctoral Thesis to obtain the degree of doctor from Radboud University Nijmegen on the authority of the Rector Magnificus prof. dr. J.H.J.M. van Krieken, according to the decision of the Council of Deans, to be defended in public on Thursday, September 12, 2019 at 12.30 hours by Mehmet Ufuk Dalmı¸s Born on May 6, 1982 in Hınıs (Turkey) Promotor: Prof. dr. N. Karssemeijer Copromotoren: Dr. R. M. Mann Dr. A. Gubern-M´erida Dr. J. Teuwen Manuscriptcommissie: Prof. dr. E. Marchiori Prof. dr. C. van Gils University Medical Center Utrecht Dr. K.G.A. Gilhuijs University Medical Center Utrecht to my aunt Suzan CONTENTS 1 TABLE OF CONTENTS 1 Introduction 3 1.1 Breast Cancer and Imaging ........................ 4 1.2 Breast MRI ................................. 5 1.3 Automated Analysis of Breast MRI .................... 8 1.4 Thesis Outline ................................ 11 2 A computer-aided diagnosis system for ultrafast breast DCE-MRI 13 2.1 Introduction ................................. 15 2.2 Materials and Methods ........................... 16 2.3 Results .................................... 29 2.4 Discussion .................................. 31 3 Using deep learning to segment breast and fibroglandular tissue in MRI volumes 35 3.1 Introduction ................................. 38 3.2 Materials and Methods ........................... 40 3.3 Results .................................... 47 3.4 Discussion .................................. 54 4 Automatically assessed FGT and BPE in relation to breast cancer risk 59 4.1 Introduction ................................. 61 4.2 Materials and Methods ........................... 62 4.3 Results .................................... 64 4.4 Discussion .................................. 66 5 Breast cancer detection in screening MRI using convolutional neural networks 83 5.1 Introduction ................................. 85 5.2 Materials and Methods ........................... 87 5.3 Results .................................... 94 5.4 Discussion .................................. 95 6 Artificial intelligence-based lesion classification in multi-parametric breast MRI 99 6.1 Introduction ................................. 101 6.2 Materials .................................. 102 2 CONTENTS 6.3 Results .................................... 108 6.4 Discussion .................................. 112 Summary and Discussion 117 Samenvatting 127 Publications 133 Bibliography 137 Acknowledgments 159 Curriculum Vitae 163 Introduction 1 4 Introduction 1.1 Breast Cancer and Imaging Breast cancer is the leading cause of cancer death in women worldwide1. Only in Europe, on average 421 000 women are diagnosed with breast cancer and 129 000 women die from the disease each year2. Some of the most important risk factors for developing breast cancer are known as breast density (BD), age, BRCA gene status and family history. Anatomy of a breast is illustrated in Fig. 1.1. Cancers that grow within milk ducts or lobules of the breast are called in situ cancers, namely ductal carcinoma in situ (DCIS) and lobular carcinoma in situ (LCIS). Cancers that infiltrate outside of ducts and lobules are called invasive cancers. Although there are several specific histological types defined for invasive cancers (such as tubular, lobular, medullary, mucoid, apocrine, anaplastic, etc.), most invasive cancers are not specifically classified but fall in the group of `no specific type' cancers and are commonly referred to as invasive ductal carcinomas (IDC). In general in situ cancers cannot metastasize and are therefore completely cured when resected. However in situ cancers possess the risk of becoming invasive. Treatment options and prognosis for invasive cancers depend on whether the cancer has spread into the lymph nodes or other organs. Therefore early detection of breast cancer has a primal importance in reducing the mortality rates. Breast cancers are also classified according to their histopathological grades and molecular subtypes. Histopathological grade is defined with values 1, 2 or 3; higher grade meaning more aggressiveness and faster growth. Molecular subtypes are related to the genetic profile of the cancer cells. Some of the most often used molecular markers that determine the molecular subtype are estrogen receptors (ER), progesterone recep- tors (PR) and human epidermal growth factor receptor 2 (HER2). These subtypes may help in identifying possible targeted therapies, such as tamoxifen for ER+ cancers or trastuzumab for HER2+ cancers. Since the life expectancy largely depends on the stage of the cancer when it is de- tected, screening programs have been introduced for detecting breast cancer as early as possible. Currently screening programs are performed with mammography. Although benefits of mammography-based screening have been shown3,4, it has serious disadvan- tages. It has the lowest sensitivity for the women with dense breasts5, which is the group of women with the highest risk of developing breast cancer, four to six times higher compared to the women that have low density breasts6. Compared to mammog- raphy, breast magnetic resonance imaging (MRI) is known to have very high sensitivity levels, as high as 91% according to a meta-analysis7, which increases further with the recent advances, especially with the introduction of 3T MRI scanners8. Moreover, it has been shown that mammography has a low sensitivity for high-grade DCIS lesions, 1.2 Breast MRI 5 Figure 1.1: Illustration of the tissues in breast and in its surrounding. Most of the breast cancers develop in milk ducts or lobules. (Image from http://nbcf.org.au). while MRI has a high sensitivity for such lesions9. Therefore, breast MRI is superior to mammography in detecting breast cancer. However, its high cost and the necessity of the use of a contrast agent limits wide use of breast MRI. Currently, screening with breast MRI is performed only for the women with increased breast cancer risk. In the recent years there have been several studies on novel abbreviated breast MRI proto- cols and ultrafast dynamic contrast-enhanced MRI (DCE-MRI) sequences to reduce the cost, which may help in application of breast MRI for general screening. 1.2 Breast MRI The main component of a breast magnetic resonance imaging (MRI) protocol is the dynamic contrast enhanced MRI (DCE-MRI) series, which is typically based on ac- quisition of T1-weighted (T1w) volumes and administration of a contrast agent. The most commonly used contrast agents for DCE-MRI are Gadolinium (Gd) based, which shortens T1 relaxation time of the tissue that uptakes this contrast agent. This makes the tissue appear brighter in the T1w MRI volumes. Although malignant lesions share the same T1w properties with healthy breast parenchyma (also referred to as
Details
-
File Typepdf
-
Upload Time-
-
Content LanguagesEnglish
-
Upload UserAnonymous/Not logged-in
-
File Pages177 Page
-
File Size-