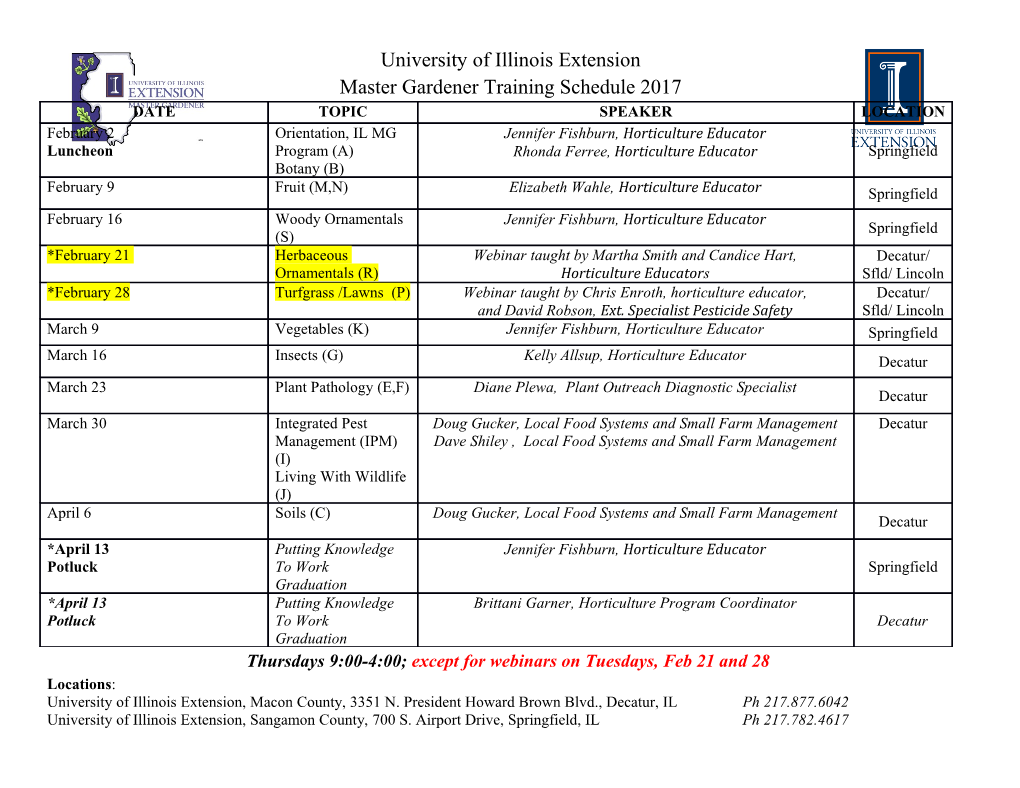
Diff-TTS: A Denoising Diffusion Model for Text-to-Speech Myeonghun Jeong1, Hyeongju Kim2, Sung Jun Cheon1, Byoung Jin Choi1, and Nam Soo Kim1 1Department of Electrical and Computer Engineering and INMC, Seoul National University, Seoul, South Korea 2Neosapience, Inc., Seoul, South Korea [email protected], [email protected], fsjcheon, [email protected] [email protected] Abstract TTS [19] are parameter-inefficient due to architectural con- straints imposed on normalizing flow-based models. Although neural text-to-speech (TTS) models have attracted a Meanwhile, another generative framework called denoising lot of attention and succeeded in generating human-like speech, diffusion has shown state-of-the-art performance on image gen- there is still room for improvements to its naturalness and ar- eration, and raw audio synthesis [20–24]. The denoising dif- chitectural efficiency. In this work, we propose a novel non- fusion models can be stably optimized according to maximum autoregressive TTS model, namely Diff-TTS, which achieves likelihood and enjoy the freedom of architecture choices. highly natural and efficient speech synthesis. Given the text, In light of the advantages of denoising diffusion, we pro- Diff-TTS exploits a denoising diffusion framework to transform pose Diff-TTS, a novel non-AR TTS that achieves robust, con- the noise signal into a mel-spectrogram via diffusion time steps. trollable, and high-quality speech synthesis. To train Diff- In order to learn the mel-spectrogram distribution conditioned TTS without any auxiliary loss function, we present a log- on the text, we present a likelihood-based optimization method likelihood-based optimization method based on denoising dif- for TTS. Furthermore, to boost up the inference speed, we lever- fusion framework for TTS. In addition, since Diff-TTS has age the accelerated sampling method that allows Diff-TTS to Markov-chain constraint, which accompanies slow inference generate raw waveforms much faster without significantly de- speed, we also introduce the accelerated sampling method grading perceptual quality. Through experiments, we verified demonstrated in [25]. Through the experiments, we show that that Diff-TTS generates 28 times faster than the real-time with Diff-TTS provides an efficient and powerful synthesis system a single NVIDIA 2080Ti GPU. compared to other best-performing models. The contributions Index Terms: speech synthesis, denoising diffusion, acceler- of our work are as follows: ated sampling • To the best of our knowledge, it is the first time that a denoising diffusion probabilistic model (DDPM) was 1. Introduction applied to non-AR TTS. The Diff-TTS can be stably Most of deep learning-based speech synthesis systems consist trained without any constraint on model architecture. of two parts: (1) a text-to-speech (TTS) model that converts text • We show that Diff-TTS generates high fidelity audios in into an acoustic feature such as mel-spectrogram [1–4] and (2) a terms of the Mean Opinion Score (MOS) with half pa- vocoder that generates time-domain speech waveform using this rameters of the Tacotron2 and Glow-TTS. acoustic feature [5–8]. In this paper, we address the acoustic • By applying the accelerated sampling, Diff-TTS allows modeling of neural TTS. users to control the trade-off between sample quality and TTS models have attracted a lot of attention in recent years inference speed based on a computational budget avail- due to the advance in deep learning. Modern TTS models can able on the device. Furthermore, Diff-TTS achieves a be categorized into two groups according to their formulation: significantly faster inference speed than real-time. (i) autoregressive (AR) models and (ii) non-AR models. The AR models can generate high-quality samples by factorizing • We analyze how Diff-TTS controls speech prosody. The the output distribution into a product of conditional distribu- pitch variability of Diff-TTS depends on variances of la- tion in sequential order. The AR models such as Tacotron2 and tent space and additive noise. Diff-TTS can effectively Transformer-TTS produce natural speech, but one of their criti- control the pitch variability by multiplying a temperature cal limitations is that inference time increases linearly with the term to variances. arXiv:2104.01409v1 [eess.AS] 3 Apr 2021 length of mel-spectrograms [2, 9]. Furthermore, the AR model This paper is organized as follows: we first introduce the lacks robustness in some cases, e.g., word skipping and repeat- denoising diffusion framework for TTS. Then, we present the ing, due to accumulated prediction error. Recently, various non- simple architecture of Diff-TTS. In the experiments, we analyze AR TTS models have been proposed to overcome the short- a number of advantages of Diff-TTS compared to other state-of- comings of the AR models [10–14]. Although non-AR TTS the-art TTS models. models are capable of synthesizing speech in a stable way and their inference procedure is much faster than AR models, the 2. Diff-TTS non-AR TTS models still have some limitations. For instance, since feed-forward models such as FastSpeech1,2 [15, 16] and In this section, we first present the probabilistic model of Diff- Speedyspeech [17] cannot produce diverse synthetic speech TTS for a mel-spectrogram generation. Then we formulate a since they are optimized by a simple regression objective func- likelihood-based objective function for training Diff-TTS. Next, tion without any probabilistic modeling. Additionally, the flow- we introduce two kinds of sampling methods for synthesis: (1) based generative models such as Flow-TTS [18] and Glow- normal diffusion sampling based on the Markovian diffusion ........ reverse process 푥푇 푥푇−1 푥푇−2 푥푇−3 푥푇−4 푥푇−5 푥푇−6 푥푇 푥푇−1 ........ 푥1 푥0 훾 = 1 diffusion process 훾 = 2 훾 = 3 Figure 1: Graphical model for the reverse process and the dif- fusion process. Figure 2: Graphical model for the accelerated sampling with γ = 1; 2; 3. process and (2) accelerated version of diffusion sampling. Fi- where t is uniformly taken from the entire diffusion time-step. nally, we present the model architecture of Diff-TTS for high Diff-TTS does not need any auxiliary losses except L1 loss fidelity synthesis. function between the output of model θ(·) and Gaussian noise ∼ N (0;I). During inference, Diff-TTS retrieves a mel- 2.1. Denoising diffusion model for TTS spectrogram from a latent variable by iteratively predicting the Diff-TTS converts the noise distribution to a mel-spectrogram diffusing noise added at each forward transition with θ(xt; t; c) distribution corresponding to the given text. As shown in Fig. 1, and removing the corrupted part as follows: the mel-spectrogram is gradually corrupted with Gaussian noise 1 1 − αt and transformed into latent variables. This process is termed xt−1 = p (xt − p θ(xt; t; c)) + σtzt; (5) αt 1 − α¯t the diffusion process. Let x1; : : : ; xT be a sequence of vari- ables with the same dimension where t = 0; 1;:::;T is the q 1−α¯t−1 where zt ∼ N (0;I) and σt = η βt. The temperature index for diffusion time steps. Then, the diffusion process trans- 1−α¯t term η is a scaling factor of the variance. Note that diffusion forms mel-spectrogram x0 into a Gaussian noise xT through a chain of Markov transitions. Each transition step is predefined time-step t is also used as an input to Diff-TTS, which allows shared parameters for all diffusion time-steps. As a result, the with a variance schedule β1; β2; :::; βT . More specifically, each transformation is performed according to the Markov transition final mel-spectrogram distribution p(x0jc) is obtained through iterative sampling over all of the preset time steps. probability q(xtjxt−1; c) assumed to be independent of the text c and it is defined as follows: 2.2. Accelerated Sampling p q(xtjxt−1; c) = N (xt; 1 − βtxt−1; βtI): (1) Although the denoising diffusion is a parallel generative model, the inference procedure can take a long time if the number of The whole diffusion process q(x1:T jx0; c) is the Markov pro- diffusion steps is large. To mitigate this problem, the denoising cess and can be factorized as follows: diffusion implicit model (DDIM) [25] introduces a new sam- T pling method called accelerated sampling for diffusion mod- Y q(x1 : : : ; xT jx0; c) = q(xtjxt−1): (2) els that boosts up the inference speed without model retrain- t=1 ing. The accelerated sampling generates samples over the sub- sequence of the entire inference trajectory. In particular, it The reverse process is a mel-spectrogram generation procedure is a quite efficient technique that the sample quality does not that is exactly backward of the diffusion process. Unlike the deteriorate significantly even with the reduced number of re- diffusion process, the goal of the reverse process is to recover verse transitions. To improve the synthesis speed while pre- a mel-spectrogram from Gaussian noise. The reverse process serving the sample quality, we also leverage the accelerated is defined as the conditional distribution pθ(x0:T −1jxT ; c), and sampling for Diff-TTS. For implementation, the reverse tran- it can be factorized into multiple transitions based on Markov sition is skipped out by a decimation factor γ. As shown chain property: in Fig. 2, the new reverse transitions are composed of peri- odically selected transitions of the original reverse path. Let T Y τ = [τ1; τ2; :::; τM ](M < T ) be a new reverse path sampled pθ(x0 : : : ; xT −1jxT ; c) = pθ(xt−1jxt; c): (3) from the time steps 1; 2; :::; T . The accelerated sampling equa- t=1 tion for i > 1 is described in Eq.
Details
-
File Typepdf
-
Upload Time-
-
Content LanguagesEnglish
-
Upload UserAnonymous/Not logged-in
-
File Pages5 Page
-
File Size-