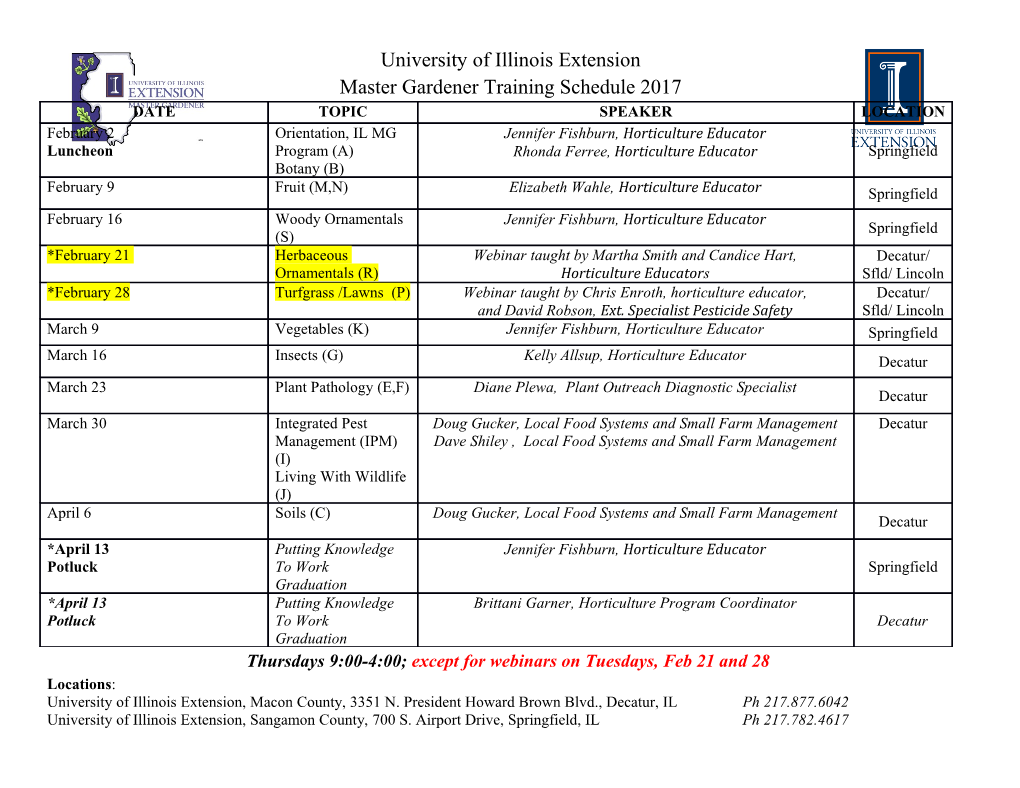
TREIMM 1345 No. of Pages 12 Review Solving Immunology? Yoram Vodovotz,1 Ashley Xia,2,17 Elizabeth L. Read,3 Josep Bassaganya-Riera,4 David A. Hafler,5 Eduardo Sontag,6 Jin Wang,7,8 John S. Tsang,9 Judy D. Day,10 Steven H. Kleinstein,11,12 Atul J. Butte,13 Matthew C. Altman,14 Ross Hammond,15 and Stuart C. Sealfon16,* Emergent responses of the immune system result from the integration of Trends molecular and cellular networks over time and across multiple organs. High- Unbiased high-throughput data-driven content and high-throughput analysis technologies, concomitantly with data- analysis approaches are identifying driven and mechanistic modeling, hold promise for the systematic interrogation molecular processes associated with of these complex pathways. However, connecting genetic variation and molec- immunological function and immune- related diseases, as well as candidate ular mechanisms to individual phenotypes and health outcomes has proven immune disease-modulating gene elusive. Gaps remain in data, and disagreements persist about the value of elements. mechanistic modeling for immunology. Here, we present the perspectives that Hypothesis-based mechanistic model- emerged from the National Institute of Allergy and Infectious Disease (NIAID) ing tests whether our understanding of workshop ‘Complex Systems Science, Modeling and Immunity’ and subsequent how a system works provides a pos- sible explanation for the existing immu- discussions regarding the potential synergy of high-throughput data acquisi- nological data, and helps focus tion, data-driven modeling, and mechanistic modeling to define new mecha- subsequent research. nisms of immunological disease and to accelerate the translation of these A variety of immunology-friendly com- insights into therapies. putational modeling tools have been developed. The Complexities of Immune Dysregulation Mechanistic modeling and simulations Dysregulation of immune and inflammatory pathways is at the core of many diseases facing are serving increasingly important roles in drug development and clinical trials. citizens of the 21st century. These diseases are multifactorial, exhibit great patient-to-patient variability, and are often intractable to both traditional therapy and reductionist insight. Immune regulation in healthy individuals is orchestrated by highly complex, massively and dynamically- interacting gene networks, immune signaling pathways, cellular networks, host–pathogen 1Departments of Surgery, interactions, and nutrition–microbiota–host interplay. Immune-mediated diseases, such as Immunology, Computational and Systems Biology, Clinical and those that stem from the response to viral or bacterial infection, as well as trauma-induced Translational Science, and inflammation, Crohn's disease (CD), type 1 diabetes mellitus (T1DM), or multiple sclerosis (MS), Communication Science and result when these homeostatic immunoregulatory mechanisms deviate or fail. Disorders, and McGowan Institute for Regenerative Medicine, University of Pittsburgh, Pittsburgh, PA 15213, USA Recent decades have brought tremendous progress in the application of ‘omics’ and associ- 2Division of Allergy, Immunology and ated bioinformatics methods to several immunological diseases. Genome and disease-mapping Transplantation, National Institute of Allergy and Infectious Diseases consortia have helped elucidate how genetic variation influences disease risk, and consortia over National Institutes of Health, 5601 the past 15 years have provided progressively finer resolution of immune disease-associated Fishers Lane, Room 7A78, MSC 9828, genetic variation [1,2]. Multidimensional and high-throughput technologies are facilitating a Rockville, MD 20852, USA 3Department of Chemical Engineering comprehensive and systematic interrogation of complex immune pathways and the identifica- & Materials Science, Department of tion of relations among their components at both the cellular and molecular levels, in both healthy Molecular Biology & Biochemistry, individuals and those with infections or immune-mediated diseases. Moreover, computational University of California, Irvine, CA 92697,[13_TD$IF] USA analyses in genome-wide association studies (GWAS) are identifying increasing numbers of 4Biocomplexity Institute of Virginia candidate immune disease-modulating genetic elements. For example, over 60% of the genetic Tech, 1015 Life Sciences Circle, variation predicting MS onset has been elucidated; these genetic variants largely overlap with Blacksburg, VA 24060, USA 5Departments of Neurology and other autoimmune diseases [3]. Immunobiology, Yale School of Trends in Immunology, Month Year, Vol. xx, No. yy http://dx.doi.org/10.1016/j.it.2016.11.006 1 © 2016 Published by Elsevier Ltd. TREIMM 1345 No. of Pages 12 A fundamental challenge for immunology is how to extend this rapidly growing, individualized Medicine, New Haven, [15_TD$IF] fi CT 06520, USA data acquisition. More speci cally: what is the optimal way to leverage focused, hypothesis- 6Department of Mathematics, Institute driven immune research to understand how immune system behavior in health and disease for Quantitative Biology, and Cancer emerges from molecular, genetic, epigenetic, cellular, and environmental modulatory elements? Institute of NJ, Rutgers University, New Brunswick, NJ 08903, USA The NIAID convened a workshop of computational and experimental immunologists in October 7State Key Laboratory of 2015 to address this challenge. At the workshop and in subsequent discussions, different Electroanalytical Chemistry, viewpoints separated experimental immunologists, data-driven modelers, and mechanistic Changchun Institute of Applied Chemistry of Chinese Academy of modelers (Figure 1). Although researchers from divergent backgrounds approach fundamental Sciences, Changchun 130022, China questions in immunology from different and sometimes clashing perspectives, they share a 8Department of Chemistry and common interest in reducing the burden of immune disease by improving the predictability of Physics, Stony Brook University, Stony Brook, NY 11794-3400, USA immunological responses. 9Systems Genomics and Bioinformatics Unit, Laboratory of A further common view shared by experimental and computational immunology researchers is Systems Biology, National Institute of ‘ ’ Allergy and Infectious Diseases, that, despite the explosion of omics and related techniques, such as mass cytometry (CyTOF), Trans-NIH Center for Human in experimental immunology, a dearth of key data is a major impediment to an improved Immunology, National Institutes of mechanistic and predictive understanding of immunology. The ability to infer novel interactions, Health, Bethesda, MD 20892, USA 10Departments of Mathematics and principal drivers, and interconnected networks using data-driven modeling, including Electrical Engineering & Computer approaches such as bioinformatics, machine-learning classifiers, or mathematical modeling Science, National Institute for of signaling networks or cell dynamics, is now well accepted [4]. However, mechanistic Mathematical and Biological Synthesis, University of Tennessee, mathematical modeling has been incorporated less well into experimental immunology research. Knoxville, TN 37996, USA Some researchers are skeptical that mechanistic models can provide predictive power, espe- 11Department of Pathology and cially in the absence of key quantitative data to anchor the model fully. However, modelers point Department of Immunobiology, Yale [17_TD$IF] [20_TD$IF] School of Medicine, New Haven, CT out that even relatively simple mathematical models can improve insight from existing data, and 06511, USA that better integration of mechanistic modeling approaches into immunology research can 12Interdepartmental Program in optimize the choice of what and when to measure, sharpen hypothesis testing, and help make Computational Biology and Bioinformatics, Yale University, New the resulting data sets more informative (Box 1). Haven, CT[17_TD$IF]06511, USA 13Institute for Computational Health The initial process of formally developing a simple and perhaps initially incorrect model that Sciences, University of California, San Francisco, Mission Hall 550 16th includes only a few entities often proves invaluable in revealing hidden assumptions, formalizing Street, 4th Floor, Box 0110, San hypotheses, and identifying key missing measurements and experiments. Importantly, data Francisco, CA 94158-2549, USA 14 mining and mechanistic modeling are synergistic approaches that are not intended to replace Department of Allergy and Infectious Diseases, University of Washington, Seattle, WA 98109, USA Figure 1. Different Perspectives of 15Center on Social Dynamics & Policy, Immunology Researchers. Generally, Economic Studies Program, The Experimental Mechanisc immunologists seek to understand the Brookings Institution, Washington DC[17_TD$IF] immunologists modelers role of immune system in health and dis- 20036, USA Damage ease, focusing on how genetic, epige- 16Center for Advanced Research on netic, and environmental variations affect Diagnostic Assays, Immunology M immune function and influence individual Institute, Friedman Brain Institute, and disease expression and response to treat- Departments of Neurology, ment. Data-driven modelers seek to map Neuroscience, Pharmacology, and TNF IL-6 predictive relations between multiple data Systems Therapeutics, Icahn School variables and disease in individuals, of Medicine at Mount Sinai, New York, IL-10 NY 10029, USA reconstructing
Details
-
File Typepdf
-
Upload Time-
-
Content LanguagesEnglish
-
Upload UserAnonymous/Not logged-in
-
File Pages12 Page
-
File Size-