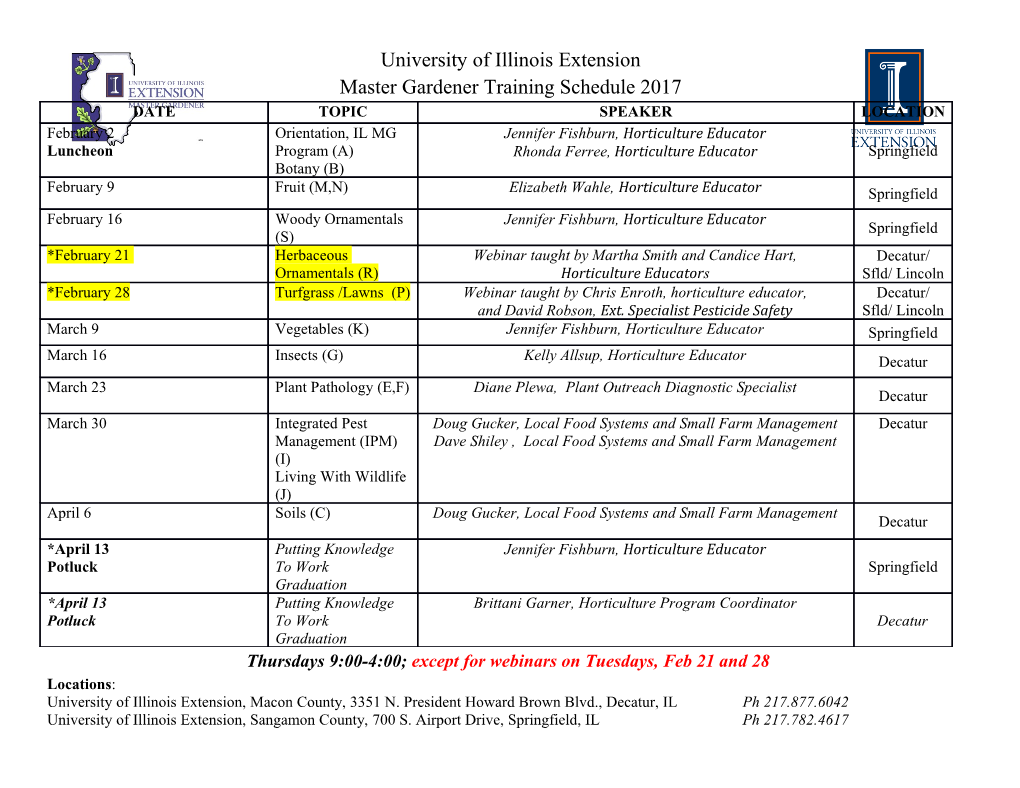
Poisson, birth and death Waiting queues Risk process Cat Bonds Birth and Poisson processes Olivier Scaillet University of Geneva and Swiss Finance Institute 1/31 Poisson, birth and death Waiting queues Risk process Cat Bonds Outline 1 Poisson, birth and death 2 Waiting queues 3 Risk process 4 Cat Bonds 2/31 Poisson, birth and death Waiting queues Risk process Cat Bonds Introduction Many natural phenomenons exhibit changes of value at random times rather than fixed times. We model this behavior with continuous-time models, that is, processes of the form fXt : t ≥ 0g, where t is continuous. The sample paths of the processes we consider here have integer values. 3/31 Poisson, birth and death Waiting queues Risk process Cat Bonds Poisson process The Poisson process is ideal to model the occurrence of successive events. Every event is such that, in a time interval (t; t + ∆t) with ∆t small, its probability of occurrence is proportional to ∆t, and that the probability of occurrence of two events in the same small interval is negligible. 4/31 Poisson, birth and death Waiting queues Risk process Cat Bonds Poisson process (cont’d) A Poisson process with intensity λ is a process N = fNt : t ≥ 0g, whose values belong to 0; 1; 2; 3; ::: and such that: 1 N0 = 0 and Ns ≤ Nt , if s < t, 2 if s < t , the number Nt − Ns of events in the time interval (s; t] is independent of the arrival times and the arrivals in the interval [0; s] (process with independent increments) 8 λ∆t + o(∆t) if i = 1 <> 3 P[Nt+∆t = n + ijNt = n] = o(∆t) if i > 1 :>1 − λ∆t + o(∆t) if i = 0 f (∆t) Notation: f (∆t) = o(∆t) is equivalent to lim∆t!0 ∆t = 0. 5/31 Poisson, birth and death Waiting queues Risk process Cat Bonds Poisson process (cont’d) The function f has a smaller asymptotic order than ∆t, and is negligible in comparison to ∆t. We say that Nt stands for the number of arrivals, occurrences, or events of the process until time t. The process fNt g is a counting process and is a simple example of continuous-time Markov chains. The intensity λ (or birth rate) corresponds to the probability of occurrence of one event per unit of time. The Poisson process Nt follows a Poisson distribution with (λt)i −λt parameter λt, P[Nt = i] = i! e , i = 0; 1; 2;::: . In particular, we have E[Nt ] = λt. 6/31 Poisson, birth and death Waiting queues Risk process Cat Bonds Poisson process (cont’d) We can also characterize the Poisson process using the duration between successive events. Let T0; T1;::: be given by T0 = 0, Tn = infft : Nt = ng. Tn is the date of the nth arrival. The durations (or intervals) are random variables dn = Tn − Tn−1. These variables d1; d2;::: are independent and follow an exponential distribution with parameter λ, whose density function is given by f (d) = λe−λd . The distribution function is given by F (d) = 1 − e−λd , and the 1 expectation of the durations is E[dt ] = λ . 7/31 Poisson, birth and death Waiting queues Risk process Cat Bonds Poisson process (cont’d) Remarks: one can rebuild the process N with durations d, using Pn Tn = i=1 di , Nt = maxfn : Tn ≤ tg. the estimation of the lambda parameter is trivial as the maximum likelihood estimator for an exponential law is the inverse of the empirical mean:λ^ = 1 , where d¯ is the empirical d¯ mean of the observed durations. 8/31 Poisson, birth and death Waiting queues Risk process Cat Bonds Birth process The Poisson process has a constant intensity (or birth rate). We may introduce a dependence between the number of previous events and the birth rate. A birth process with intensities λ0; λ1; λ2;::: is a process N = fNt : t ≥ 0g, whose values lie in f0; 1; 2; 3;:::g and such that: 1 N0 = 0 and Ns ≤ Nt , if s < t 2 if s < t , conditionally on the value of Ns , the number of events Nt − Ns in the time interval (s; t] is independent of all previous arrivals in the interval[0; s] 8 λ ∆t + o(∆t) if i = 1 <> n 3 P[Nt+∆t = n + ijNt = n] = o(∆t) if i > 1 > :1 − λn∆t + o(∆t) if i = 0 9/31 Poisson, birth and death Waiting queues Risk process Cat Bonds Birth process (cont’d) Particular cases: Poisson process: λn = λ, 8n Simple birth: λn = nλ. Population growth where each individual gives birth independently of the others, with probability λ∆t + o(∆t) during the time interval (t; t + ∆t), and where there is no death. Birth with immigration: λn = nλ + v. Birth process where the birth rate depends on an external immigration rate. 10/31 Poisson, birth and death Waiting queues Risk process Cat Bonds Birth and death process A more realistic model in the context of a population growth also allows for deaths. Let Nt be the number of alive individuals at time t in a particular population. This number follows a birth and death process if: 1 Nt is a Markov chain with values f0; 1; 2;:::g 2 P[Nt+∆t = n + ijNt = n] = 8 λ ∆t + o(∆t) if i = 1 > n > <µn∆t + o(∆t) if i = −1 o(∆t) if jij > 1 > > :1 − λn∆t − µn∆t + o(∆t) if i = 0 3 The birth rates λ0; λ1; λ2;::: and death rates µ0; µ1; µ2;::: are such that λi ≥ 0, µi ≥ 0, and µ0 = 0. 11/31 Poisson, birth and death Waiting queues Risk process Cat Bonds Birth and death process (cont’d) Particular cases: Birth process: µn = 0, 8n. Simple death with immigration: λn = λ, µn = nµ, 8n. Population that does not give birth but with some immigration corresponding to a Poisson process, and for which each individual has a probability µ∆t + o(∆t) to die in the time interval (t; t + ∆t). Simple birth and death: λn = nλ, µn = nµ, 8n. Each invidividual that is alive may either die during the time interval (t; t + ∆t) with a probability µ∆t + o(∆t), or split with a probability λ∆t + o(∆t). 12/31 Poisson, birth and death Waiting queues Risk process Cat Bonds Waiting queues Waiting queues theory is an important application of the stochastic process theory. Practical examples: Connections to a server. Call center capacity. Number of counters in a bank. 13/31 Poisson, birth and death Waiting queues Risk process Cat Bonds Inflows A waiting queue has an input stream that stands for the client arrivals (“client” has a broad meaning, e.g., cars, memory calls of a computer, phone call in a call center). As a first approximation, we assume that the durations between arrivals are i.i.d. random variables. The input stream is then a so-called stationary renewal process. We get the simplest case by using a duration following the exponential law: the input flow follows a Poisson process. 14/31 Poisson, birth and death Waiting queues Risk process Cat Bonds Service A waiting queue generally has a service provider that is specified by The duration of the service, whose distribution is assumed to be known. The number of ticket offices (or counters). 15/31 Poisson, birth and death Waiting queues Risk process Cat Bonds Service (cont’d) A waiting queue is also characterized by a rule of service that defines the service behavior: System with or without waiting queue (i.e., in a system without queue, clients are lost if they are not served immediately); system with a limited capacity (i.e., clients are lost when the queue is too long). The order in which clients are served: first in first out (FIFO), last in first out (LIFO). Multiple client priority classes. Contingent probability of client loss: the client may be lost with a probability depending on the length of the queue. 16/31 Poisson, birth and death Waiting queues Risk process Cat Bonds Kendall notation For a mathematical study, we classify the waiting queues following the standardized Kendall notation A/B/C/D. The letters have the following meaning: A: input stream B: duration of service C: number of ticket offices D: additional information, as follows. The letter M (for Markov) for input streams stands for a Poisson stream; for the duration of service, it stands for an exponential duration. The letter D (for Determinist) for input streams stands for regular interval durations; for the duration of service, it stands for a fixed time for any client. The letter G stands for the general case. 17/31 Poisson, birth and death Waiting queues Risk process Cat Bonds Example: M/M/1 queue It is the simplest example of a waiting queue: The input stream follows a Poisson process with intensity λ, or, equivalently, the durations between the arrivals follow an i.i.d. exponential distribution with parameter λ. The service durations are i.i.d. and follow an exponential law with parameter µ. There is only one ticket office and client are served according to a first in first out rule; the capacity of the queue is infinite. Remark: the M/M/1 queue is equivalent to a birth and death process whose birth and death rates are given by λn = λ, 8n and µ0 = 0, µn = µ, 8n > 0, respectively. 18/31 Poisson, birth and death Waiting queues Risk process Cat Bonds Example: M/M/1 queue (cont’d) If the ticket office is open without interruption, the average service rate is equal to µ.
Details
-
File Typepdf
-
Upload Time-
-
Content LanguagesEnglish
-
Upload UserAnonymous/Not logged-in
-
File Pages31 Page
-
File Size-