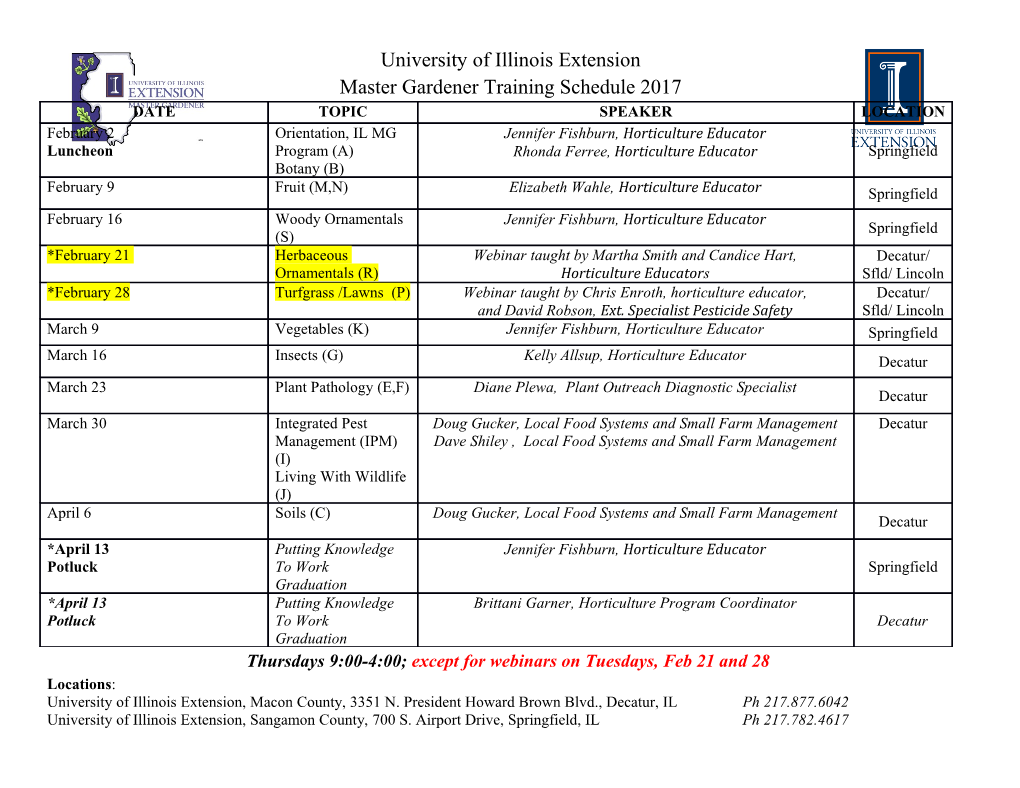
UNLV Theses, Dissertations, Professional Papers, and Capstones 5-1-2014 Smart Data Collection Using Mobile Devices To Improve Transportation Systems Tharindu Dasun Abeygunawardana University of Nevada, Las Vegas Follow this and additional works at: https://digitalscholarship.unlv.edu/thesesdissertations Part of the Computer Sciences Commons, Transportation Commons, and the Urban Studies and Planning Commons Repository Citation Abeygunawardana, Tharindu Dasun, "Smart Data Collection Using Mobile Devices To Improve Transportation Systems" (2014). UNLV Theses, Dissertations, Professional Papers, and Capstones. 2052. http://dx.doi.org/10.34917/5836071 This Thesis is protected by copyright and/or related rights. It has been brought to you by Digital Scholarship@UNLV with permission from the rights-holder(s). You are free to use this Thesis in any way that is permitted by the copyright and related rights legislation that applies to your use. For other uses you need to obtain permission from the rights-holder(s) directly, unless additional rights are indicated by a Creative Commons license in the record and/ or on the work itself. This Thesis has been accepted for inclusion in UNLV Theses, Dissertations, Professional Papers, and Capstones by an authorized administrator of Digital Scholarship@UNLV. For more information, please contact [email protected]. SMART DATA COLLECTION USING MOBILE DEVICES TO IMPROVE TRANSPORTATION SYSTEMS by Tharindu D. Abeygunawardana Bachelor of Science (B.Sc.) University of Nevada, Las Vegas 2010 A thesis submitted in partial fulfillment of the requirements for the Master of Science – Computer Science Department of Computer Science Howard R. Hughes College of Engineering The Graduate College University of Nevada, Las Vegas May 2014 c Tharindu D. Abeygunawardana, 2014 All Rights Reserved Abstract Travel time is a matter that a↵ects most of us, especially those that live in highly congested cities. Ideally, we want to reduce travel time as much as possible, thereby freeing up more of our time and enabling a higher quality of life. Data collection of transportation metrics helps us get a clearer picture of the transportation system, and helps us make smarter choices when it comes to improving the existing system. The rapid emergence of interconnected mobile devices carried along by travelers opens up many possibilities for gathering data as they travel, and also to serve them relevant data so that they can make smarter choices when it comes to their traveling. It is estimated that the market share of smartphones would continue to grow for the foreseeable future, making it a rich source for data collection at large scale. Therefore, with the goal of minimizing travel time in mind, we explore various means of making use of these mobile devices to collect data and ultimately improve transportation systems. In particular, we report on the evolution of data collection in the area of transportation research, report on novel case studies in this area, and report in depth on one implementation of a smart data collection application. iii Acknowledgements I would like to thank Dr. Taghva for his guidance, patience, and support throughout the project. Dr. Taghva gave me the basis to start working on the application that is discussed in this paper, and was helpful in working through the challenges in the project. I would also like to thank my father for showing me the value of education, discipline, and humility. I would like to thank my mother for her continued support and encouragement to complete my studies. Finally, I would like to thank many friends that listened to what I was working on, gave back encouragement and ideas, and helped me proofread the paper. Tharindu D. Abeygunawardana University of Nevada, Las Vegas May 2014 iv Table of Contents Abstract iii Acknowledgements iv Table of Contents v List of Tables vii List of Figures viii Chapter 1 Introduction 1 Chapter 2 Background 3 2.1 Smart Data Collection . 3 2.2 Manual Data Collection Strategies . 5 2.3 Traffic Monitoring using Cell Tower Information . 8 2.4 DOTImplementations .................................. 9 Chapter 3 Case Studies 11 3.1 MobileMillennium&MobileCenturyFieldExperiment . 11 3.2 Google Maps TrafficEstimates ............................. 13 3.3 Nericell .......................................... 14 3.4 ParkSense......................................... 16 3.5 PredictingBusArrivalTime. 18 Chapter 4 NDOT Smart Data Collection Application 21 4.1 Design&Implementation ................................ 22 4.1.1 Application Architecture Overview . 23 4.1.2 Tools and Technologies . 24 4.1.3 Client Side Mobile Application . 25 4.1.4 ServerSideWebService ............................. 27 v 4.1.5 Live Incident Reports Map . 33 4.2 TestSetup......................................... 35 4.3 TestResults........................................ 36 4.4 Analysis.......................................... 37 4.5 FutureWorks....................................... 38 Chapter 5 Conclusion 40 Appendix A NDOT Application Screenshots 42 Appendix B NDOT Application Source Code 46 Bibliography 47 Vita 50 vi List of Tables 4.1 Testresultsforincidentinvolvingtwoactors. 36 4.2 Testresultsforincidentinvolvingthreeactors. 36 4.3 Test results for incident involving one actor. 36 vii List of Figures 2.1 InductiveLoopDetector. .................................. 6 3.1 Google Maps TrafficEstimates................................ 14 4.1 SR1 Form, Report of TrafficAccident. ........................... 22 4.2 Architectural overview of the application. 23 4.3 Location View Markup. 25 4.4 Codesnippettoopenanewview. ............................. 26 4.5 Client side mobile application sequence diagram. 27 4.6 Serversidewebservicesequencediagram. 28 4.7 Sr1ClientFormDatamodelobject. 28 4.8 NVDriverLicensebarcodeencoding.. 30 4.9 Unit test to test form gets processed correctly for a valid request. 31 4.10 Live incident reports map on a mobile phone. 33 4.11 Code snippet showing server calling JavaScript client method. 34 4.12 Chart showing data entry method vs completion time. Data from table 4.1. 37 4.13 Chart showing average completion time for each of the data entry methods as a per- centage. Data from table 4.2. 38 A.1 Home screen of the app, start point for the SR1 form. 42 A.2 Location screen where the user inputs location of the incident. 43 A.3 Actors screen showing all of the actors involved in the trafficincident. 43 A.4 Actor detail screen where the user inputs details of the actor. 44 A.5 User can manually input details of the actor as well. 44 A.6 History screen showing a summary of previously submitted form data. 45 A.7 History detail screen showing the full form data. 45 viii Chapter 1 Introduction The prevalence of smart mobile devices among motorists opens up possibilities for improving trans- portation systems. These mobile devices are equipped with many instruments for data gathering, some of which include the GPS chip, proximity sensor, ambient light sensor, accelerometer, mag- netometer, and gyroscope [1]. Some areas for improvement we consider in this paper include using the phone directly as a data input device instead of using paper forms, using real time data from the phone’s GPS to monitor traffic instead of expensive sensors installed on roadways, and usage of smartphone sensors to get a more realistic and accurate picture of the transportation system. The approach taken in this study was to research and report on case studies involving the usage of smartphone sensor data to improve transportation systems, and then describe one implementation of a smart data collection application. This area of research is interesting because smartphone usage is increasing, the cost of data connection is decreasing, the speed of data connection is increasing, and roadway congestion con- tinues to be a major problem in highly populated areas. Even a small improvement in travel time can have a huge impact on the overall transportation system, and can bring big savings in resources for the entire population. It is relatively expensive to install physical devices on roadways, while smartphone data can reduce the costs and also give more accurate predictions. Furthermore, the usage of smartphone data to replace already existing roadside sensors can reduce maintenance costs for the governing authorities in the long run. Additionally the increase in the amount of sensor data available increases the demand for smarter ways of collecting the data and processing it for useful purposes. There are still too many cases where pen and paper are used as the means of data gathering, when it is much more efficient to make use of mobile devices. In chapter two we give a summary of previous work in the literature, and explore the sub- ject of smart data collection as it pertains to transportation research. We briefly explore various means of smart data collection methods that have been used in the past, including the usage 1 of radio frequency identification transponders, automatic license plate recognition systems, GPS and/or Automatic Vehicle Location technology built into existing fleets of vehicles, GPS enabled smartphone, and wireless sensor nodes. Manual data collection strategies that were used for many decades in the past are also discussed to compare and contrast with the newer methods that are being implemented. Traffic monitoring using existing cell tower information is also consid- ered because of its potential to be used for traffic monitoring even without smartphones with data
Details
-
File Typepdf
-
Upload Time-
-
Content LanguagesEnglish
-
Upload UserAnonymous/Not logged-in
-
File Pages60 Page
-
File Size-