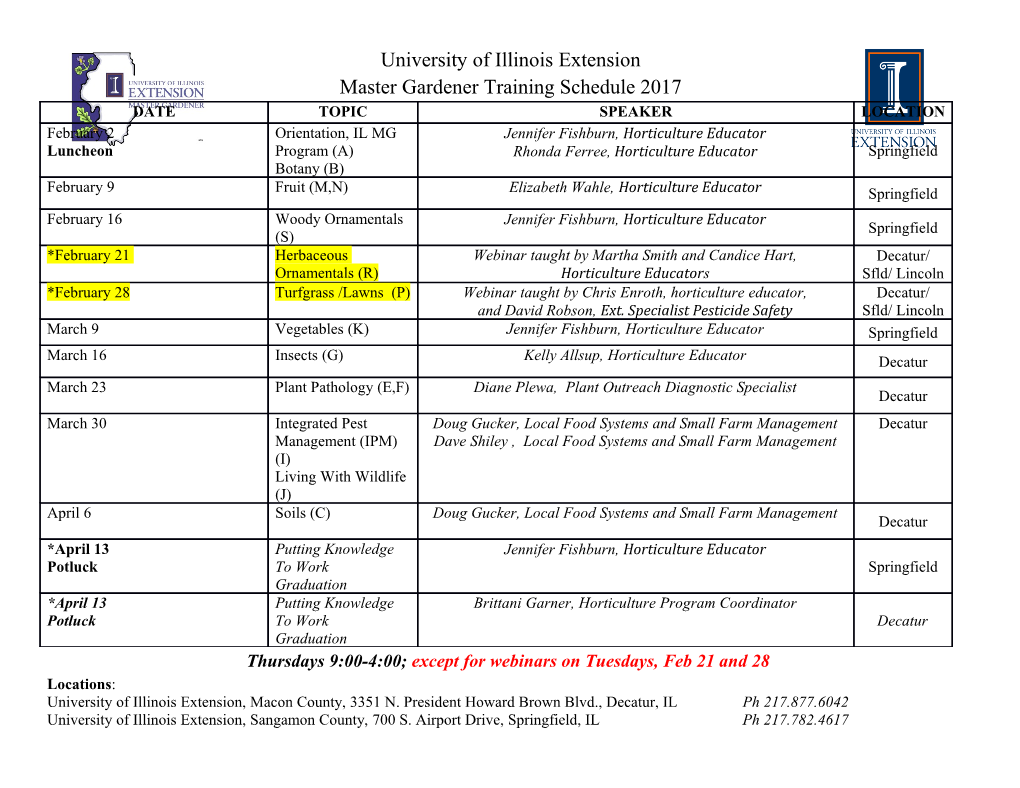
969 54. Computational Neurogenetic Modeling: Gene-Dependent Dynamics of Cortex Computationaand Idiopathic Epilepsy Lubica Benuskova, Nikola Kasabov 54.1 Overview 969 The chapter describes a novel computational ap- .............................................. 54.1.1 Neurogenesis............................. 970 proach to modeling the cortex dynamics that 54.1.2 Circadian Rhythms ..................... 970 integrates gene–protein regulatory networks with 54.1.3 Neurodegenerative Diseases ........ 970 a neural network model. Interaction of genes 54.1.4 Idiopathic Epilepsies .................. 970 and proteins in neurons affects the dynamics of 54.1.5 Computational Models the whole neural network. We have adopted an of Epilepsies .............................. 971 exploratory approach of investigating many ran- 54.1.6 Outline of the Chapter ................ 973 domly generated gene regulatory matrices out of which we kept those that generated interesting 54.2 Gene Expression Regulation .................. 974 54.2.1 Protein Synthesis ....................... 974 dynamics. This naïve brute force approach served Part K 54.2.2 Control of Gene Expression .......... 975 us to explore the potential application of compu- tational neurogenetic models in relation to gene 54.3 Computational Neurogenetic Model ....... 975 knock-out experiments. The knock out of a hy- 54.3.1 Gene–Protein 54 pothetical gene for fast inhibition in our artificial Regulatory Network .................... 975 genome has led to an interesting neural activity. 54.3.2 Proteins and Neural Parameters... 977 In spite of the fact that the artificial gene/protein 54.3.3 Thalamo-Cortical Model .............. 977 network has been altered due to one gene knock 54.4 Dynamics of the Model.......................... 979 out, the dynamics of SNN in terms of spiking activ- 54.4.1 Estimation of Parameters ............ 979 ity was most of the time very similar to the result 54.4.2 Simulation Plan ......................... 980 obtained with the complete gene/protein network. 54.5 Results of Computer Simulations............ 981 However, from time to time the neurons spon- 54.5.1 Dynamics of the Gene–Protein taneously temporarily synchronized their spiking Regulatory Network .................... 981 into coherent global oscillations. In our model, the 54.5.2 Dynamics of GPRN fluctuations in the values of neuronal parameters After Gene Knock Out.................. 982 leads to spontaneous development of seizure-like 54.5.3 Dynamics of the Cortex ............... 983 global synchronizations. These very same fluctua- 54.5.4 Dynamics of the Cortex tions also lead to termination of the seizure-like After Gene Knock Out.................. 983 neural activity and maintenance of the inter-ictal 54.6 Discussion and Future Directions............ 984 normal periods of activity. Based on our model, 54.6.1 Q and A for Neurogenetic we would like to suggest a hypothesis that param- Modeling .................................. 985 eter changes due to the gene–protein dynamics 54.6.2 Hypothesis ................................ 986 should also be included as a serious factor deter- 54.6.3 Robustness of Gene Regulatory mining transitions in neural dynamics, especially Networks .................................. 987 when the cause of disease is known to be genetic. References .................................................. 988 54.1 Overview Properties of all cell types, including neurons, are de- types and levels of proteins are determined by differ- termined by proteins they contain [54.1]. In turn, the ential transcription of genes in response to internal and 970 Part K Information Modeling for Understanding and Curing Brain Diseases external signals. Eventually, the properties of neurons 54.1.3 Neurodegenerative Diseases determine the structure and dynamics of the whole neu- ral network they are part of. In this chapter, we turn Many diseases that affect the central nervous system our attention to modeling the influence of genes and and manifest cognitive symptoms have an underlying proteins upon the dynamics of mature cortex and the dy- genetic cause – some are due to a mutation in a sin- namic effects due to mutations of genes. The chapter is gle gene, others are proving to have a more complex thus an extension and further development of a general mode of inheritance [54.9]. A large group of disor- framework for modeling brain functions and genetically ders are neurodegenerative disorders (like Alzheimer’s caused dysfunctions by means of computational neuro- disease, Rett syndrome, Huntington disease, etc.), in genetic modeling [54.2]. which the underlying gene mutations lead to particular degenerative processes in the brain that progressively 54.1.1 Neurogenesis affect neural functions and eventually have fatal conse- quences. In the case of neurodegenerative diseases, the Sophisticated mathematical and computer models of the dynamics of neural networks degeneration is slow, usu- general control of gene expression during early embry- ally lasting for years. The gene mutation is always there onic development of neural system in vertebrates have and particular degenerative changes in the brain tissue been developed [54.3]. Computational models based on accumulate over time. It is a challenge for future com- the analogy of gene regulatory networks with artificial putational neurogenetic-genetic models to attempt to neural networks have been applied to model the steps model the onset and progression of these diseases using Part K in Drosophila early neurogenesis [54.4, 5]. The latter the integration of gene regulatory networks and artifi- models attempted to elucidate how genes orchestrate cial neural networks. Already for Alzheimer’s disease, the detailed pattern of early neural development. During computational models of neural networks’ dysfunction 54.1 early development, dynamics of changes in architecture caused by experimentally identified biochemical fac- and morphology of neural network parallels changes in tors that result from genetic abnormalities have been gene expression in time. developed to gain insights into the neural symptoms of the disease [54.10–12]. All these neurodegenera- 54.1.2 Circadian Rhythms tive diseases (like Alzheimer’s disease, Rett syndrome, Huntington disease, etc.) are characterized by the fact One particular instance where the gene expression de- that once the gene mutation manifests itself, the disease termines the neural dynamics is the circadian rhythm. progresses and its symptoms of cognitive impairment A circadian rhythm is a roughly-24 h cycle in the physi- are always there. ological processes of plants and animals. The circadian rhythm partly depends on external cues such as sun- 54.1.4 Idiopathic Epilepsies light and temperature, but otherwise it is determined by periodic expression patterns of the so-called clock However, there are genetic diseases of the brain like, for genes [54.6, 7]. Smolen et al. [54.8] have developed instance, some genetically caused epilepsies, in which a computational model to represent the regulation of the main symptom – seizure – occurs only from time core clock component genes in Drosophila (per, vri, to time and between these episodes, in fact most of Pdp-1,andClk). To model the dynamics of gene ex- the time, the brain activity appears normal. In general, pression, differential equations and first-order kinetics epilepsy is a disorder characterized by the occurrence equations were employed for modeling the control of of at least two unprovoked seizures [54.13]. Seizures genes and their products. The model illustrates the are the manifestation of abnormal hypersynchronous ways in which negative and positive feedback loops discharges of neurons in the cerebral cortex. The clin- within the gene regulatory network (GRN) cooperate ical signs or symptoms of seizures depend on the to generate oscillations of gene expression. The relative location and extent of the propagation of the discharg- amplitudes and phases of simulated oscillations of gene ing cortical neurons. The prevalence of active epilepsy expressions resemble empirical data in most simulated is about 1% [54.13], which, however, means about situations. The model of Smolen et al. [54.8]showsthat 70 million people worldwide are affected. Seizures are it is possible to develop detailed models of gene control often a common manifestation of neurologic injury and of neural behavior provided that enough experimental disease, which should not be surprising because the data is available to adjust the model. main function of neurons is the transmission of elec- Computational Neurogenetic Modeling: Gene-Dependent Dynamics 54.1 Overview 971 trical impulses. Thus, most epilepsies are not caused high-frequency stimulation, a mechanism implied in genetically. A particular case of nongenetic epilepsy the generation of epileptic activity [54.28]. Through is temporal lobe epilepsy (TLE). The most common an increase in inhibition, the absence of PV facili- pathological finding in TLE is hippocampal (temporal tates hypersynchrony through the depolarizing action of lobe) sclerosis that involves cell loss [54.14]. High- GABA [54.26]. Thus there is a permanent change in resolution MRI shows hippocampal atrophy in 87% the spectrum of the local field potential (LFP)ofthe of patients with TLE. Causes of the cell loss involve: PV gene KO mice. We developed a hierarchical spik- past infections, trauma, and vascular and brain tissue ing neural network model of LFP, in which each neuron malformations including
Details
-
File Typepdf
-
Upload Time-
-
Content LanguagesEnglish
-
Upload UserAnonymous/Not logged-in
-
File Pages23 Page
-
File Size-