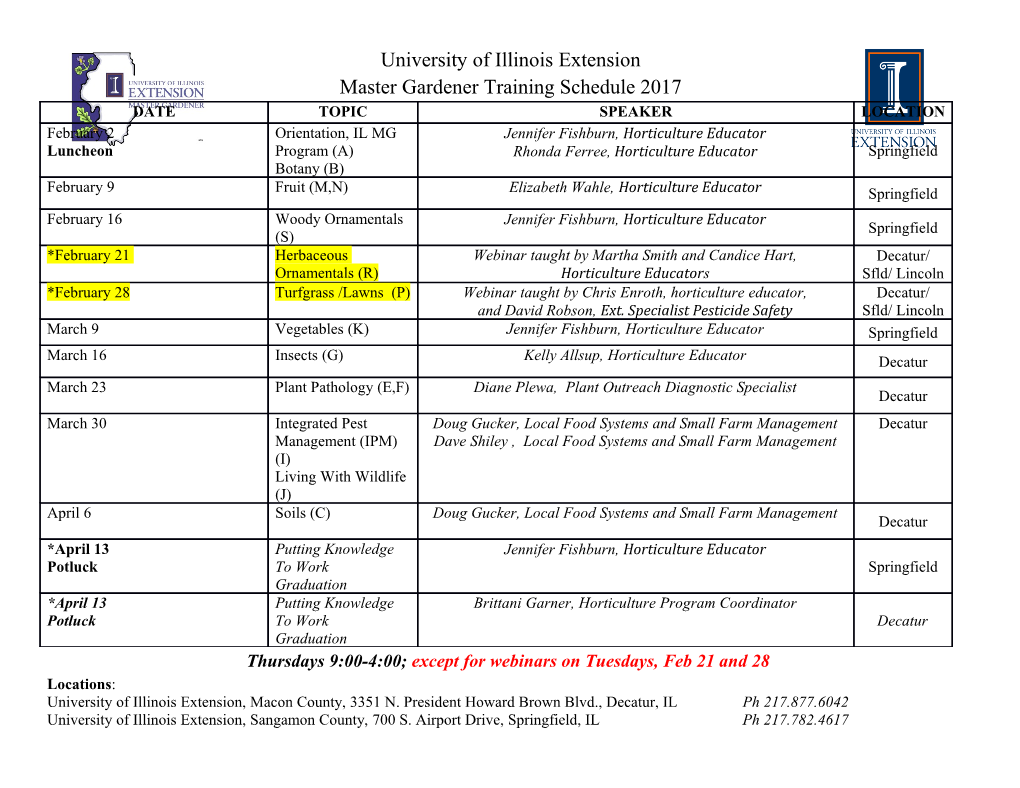
Non-Parametric Policy Gradients: A Unified Treatment of Propositional and Relational Domains Kristian Kersting [email protected] Dept. of Knowledge Discovery, Fraunhofer IAIS, Schloss Birlinghoven, 53754 Sankt Augustin, Germany Kurt Driessens [email protected] Dept. of Computer Science, Katholieke Universiteit Leuven, Celestijnenlaan 200A, 3001 Heverlee, Belgium Abstract ceives to estimate a value-function indicating the ex- pected value of being in a state or of taking an action Policy gradient approaches are a powerful in- in a state. The policy is represented only implicitly, strument for learning how to interact with for instance as the policy that selects in each state the the environment. Existing approaches have action with highest estimated value. As Sutton et al. focused on propositional and continuous do- (2000) point out, the value function approach, how- mains only. Without extensive feature engi- ever, has several limitations. First, it seeks to find de- neering, it is difficult – if not impossible – terministic policies, whereas in real world applications to apply them within structured domains, in the optimal policy is often stochastic, selecting differ- which e.g. there is a varying number of ob- ent actions with specific probabilities. Second, a small jects and relations among them. In this pa- change in the value-function parameter can push the per, we describe a non-parametric policy gra- value of one action over that of another, causing a dis- dient approach – called NPPG – that over- continuous change in the policy, the states visited, and comes this limitation. The key idea is to overall performance. Such discontinuous changes have apply Friedmann’s gradient boosting: poli- been identified as a key obstacle to establishing con- cies are represented as a weighted sum of re- vergence assurances for algorithms following the value- gression models grown in an stage-wise opti- function approach (Bertsekas & Tsitsiklis, 1996). Fi- mization. Employing off-the-shelf regression nally, value-functions can often be much more complex learners, NPPG can deal with propositional, to represent than the corresponding policy as they en- continuous, and relational domains in a uni- code information about both the size and distance to fied way. Our experimental results show that the appropriate rewards. Therefore, it is not surprising it can even improve on established results. that so called policy gradient methods have been de- veloped that attempt to avoid learning a value function explicitly (Williams, 1992; Baxter et al., 2001; Konda 1. Introduction & Tsitsiklis, 2003). Given a space of parameterized Acting optimally under uncertainty is a central prob- policies, they compute the gradient of the expected lem of artificial intelligence. If an agents learns to act reward with respect to the policy’s parameters, move solely on the basis of the rewards associated with ac- the parameters into the direction of the gradient, and tions taken, this is called reinforcement learning (Sut- repeat this until they reach a local optimum. This ton & Barto, 1998). More precisely, the agent’s learn- direct approximation of the policy overcomes the lim- ing task is to find a policy for action selection that itations of the value function approach stated above. maximizes its reward over the long run. For instance, Sutton et al. (2000) show convergence even when using function approximation. The dominant reinforcement learning (RL) approach for the last decade has been the value-function ap- Current policy gradient methods have focused on proach. An agent uses the reward it occasionally re- propositional and continuous domains assuming the environment of the learning agent to be representable Appearing in Proceedings of the 25 th International Confer- as a vector-space. Nowadays, the role of structure ence on Machine Learning, Helsinki, Finland, 2008. Copy- and relations in the data, however, becomes more and right 2008 by the author(s)/owner(s). Non-Parametric Policy Gradients: A Unified Treatment of Propositional and Relational Domains more important (Getoor & Taskar, 2007): information Consider the standard RL framework (Sutton & Barto, about one object can help the agent to reach conclu- 1998), where an agent interacts with a Markov Deci- sions about other objects. Such domains are hard to sion Process (MDP). The MDP is defined by a number represent meaningfully using a fixed set of features. of states s ∈ S, a number of actions a ∈ A, state- Therefore, relational RL approaches have been devel- transition probabilities δ(s, a, s0): S × A × S → [0, 1] oped (Dˇzeroski et al., 2001), which seek to avoid ex- that represent the probability that taking action a in plicit state and action enumeration as – in principle state s will result in a transition to state s0 and a re- – traditionally done in RL through a symbolic repre- ward function r(s, a): S × A 7→ IR. When the reward sentation of states and actions. Existing relational RL function is nondeterministic, we will use the expected approaches, however, have focused on value functions rewards R(s, a) = Es,a [r(s, a)], where Es,a denotes the and, hence, suffer from the same problems as their expectation over all states s and actions a. The state, propositional counterparts as listed above. action, and reward at time t are denoted as st ∈ S, a ∈ A and r (or R ) ∈ IR. In this paper, we present the first model-free pol- t t t icy gradient approach that deals with relational and The agent selects which action to execute in a state propositional domains. Specifically, we present a following a policy function π(s, a): S × A → [0, 1]. non-parametric approach to policy gradients, called Current policy gradient approaches assume that the NPPG. Triggered by the observation that finding policy function π is parameterized by a (weight) vector many rough rules of thumb of how to change the way θ ∈ IRn and that this policy function is differentiable to act can be a lot easier than finding a single, highly ∂π(s,a,θ) with respect to its parameters, i.e., that ∂θ ex- accurate policy, we apply Friedmann’s (2001) gradient ists. A common choice is a Gibbs distribution based boosting. That is, we represent policies as weighted on a linear combination of features: sums of regression models grown in a stage-wise op- X π(s, a) = eΨ(s,a)/ eΨ(s,b) , (1) timization. Such a functional gradient approach has b recently been used to efficiently train conditional ran- T dom fields for labeling (relational) sequences using where the potential function Ψ(s, a) = θ φsa with φsa boosting (Dietterich et al., 2004; Gutmann & Ker- the feature vector describing state s and action a. This sting, 2006) and for policy search in continuous do- representation guarantees that the policy specifies a mains (Bagnell & Schneider, 2003). In contrast to probability distribution independent of the exact form the supervised learning setting of the sequence labeling of the function Ψ. Choosing a parameterization, cre- n task, feedback on the performance is received only at ates an explicit policy space IR with n equal to size the end of an action sequence in the policy search set- of the parameter vector θ. This space can be traversed ting. The benefits of a boosting approach to functional by an appropriate search algorithm. policy gradients are twofold. First, interactions among Policy gradients are computed w.r.t. a func- states and actions are introduced only as needed, so tion ρ that expresses the value of a policy in that the potentially infinite search space is not explic- P π P an environment, ρ(π) = s∈S d (s) a∈A π(s, a) · itly considered. Second, existing off-the-shelf regres- Qπ(s, a) , where Qπ is the usual (possibly dis- sion learners can be used to deal with propositional counted) state-action value function, e.g., Qπ(s, a) = and relational domains in a unified way. To the best of P∞ i π Eπ i=0 γ Rt+i|st = s, at = a , and d (s) is the (pos- the authors’ knowledge, this is the first time that such sibly discounted) stationary distribution of states un- a unified treatment is established. As our experimen- der policy π. We assume a fully ergodic environment tal results show, NPPG can even significantly improve so that dπ(s) exists and is independent of any starting upon established results in relational domains. state s0 for all policies. We proceed as follows. We will start off by reviewing A property of ρ for both average reward reinforcement policy gradients and their mathematical background. learning and episodic tasks with a fixed starting state Afterwards, we will develop in Section 3. In NPPG s0 is that (Sutton et al., 2000, Theorem 1) Section 4, we will present our experimental results. ∂ρ X X ∂π(s, a) Before concluding, we will touch upon related work. = dπ(s) · Qπ(s, a) . (2) ∂θ s a ∂θ 2. Policy Gradients Important to note is that this gradient does not in- ∂dπ (s) clude the term ∂θ . Eq. (2) allows the computation Policy gradient algorithms find a locally optimal policy of an approximate policy gradient through exploration. starting from an arbitrary initial policy using a gra- By sampling states s through exploration of the envi- dient ascent search through an explicit policy space. ronment following policy π, the distribution dπ(s) is Non-Parametric Policy Gradients: A Unified Treatment of Propositional and Relational Domains automatically represented in the generated sample of tions. Specifically, one starts with some initial func- P ∂π(s,a) π tion Ψ , e.g. based on the zero potential, and itera- encountered states. The sum a ∂θ Q (s, a) then 0 ∂ρ tively adds corrections Ψm = Ψ0 + ∆1 + ..
Details
-
File Typepdf
-
Upload Time-
-
Content LanguagesEnglish
-
Upload UserAnonymous/Not logged-in
-
File Pages8 Page
-
File Size-