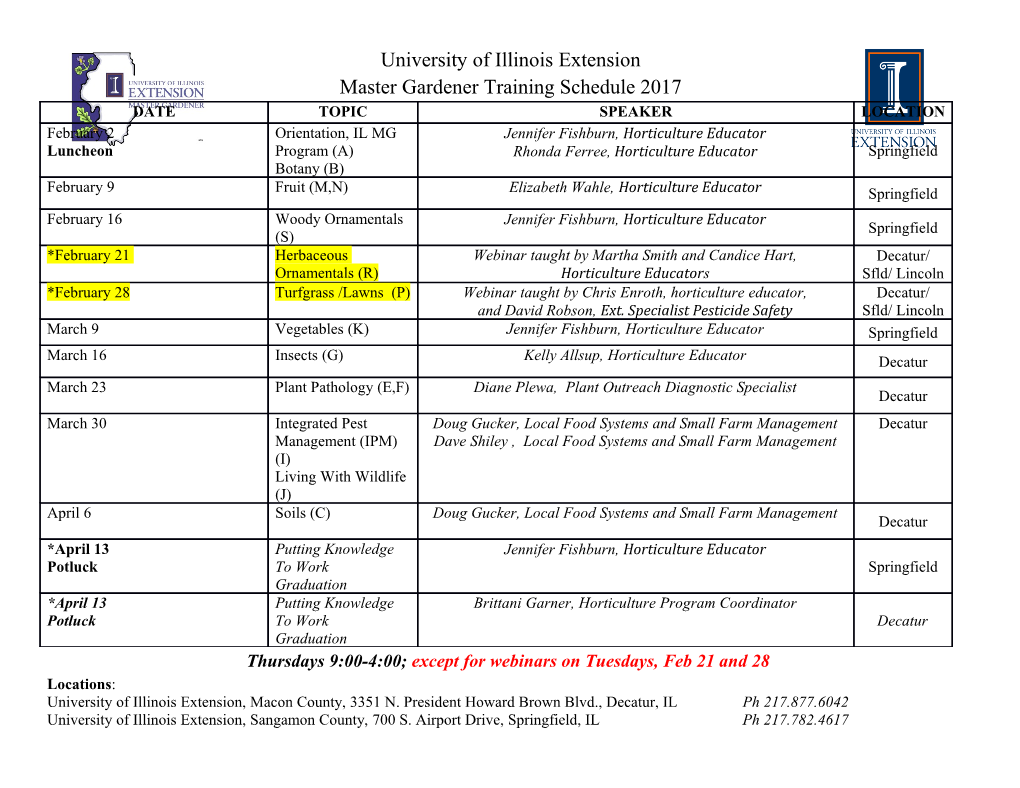
http://www.diva-portal.org Postprint This is the accepted version of a paper published in IEEE Robotics and Automation Letters. This paper has been peer-reviewed but does not include the final publisher proof-corrections or journal pagination. Citation for the original published paper (version of record): Kucner, T P., Magnusson, M., Schaffernicht, E., Hernandez Bennetts, V M., Lilienthal, A J. (2017) Enabling Flow Awareness for Mobile Robots in Partially Observable Environments IEEE Robotics and Automation Letters, 2(2): 1093-1100 https://doi.org/10.1109/LRA.2017.2660060 Access to the published version may require subscription. N.B. When citing this work, cite the original published paper. Permanent link to this version: http://urn.kb.se/resolve?urn=urn:nbn:se:oru:diva-55174 Enabling Flow Awareness for Mobile Robots in Partially Observable Environments Tomasz Piotr Kucner1, Martin Magnusson1, Erik Schaffernicht1, Victor Hernandez Bennetts1, and Achim J. Lilienthal1 Abstract— Understanding the environment is a key require- local airflow. However, the task of wind flow modelling in ment for any autonomous robot operation. There is extensive small scales is rarely addressed [20]. Moreover, for tasks research on mapping geometric structure and perceiving ob- considering long term surveillance with aerial vehicles it jects. However, the environment is also defined by the movement patterns in it. Information about human motion patterns can, is important to plan energy efficient paths considering air e.g., lead to safer and socially more acceptable robot trajecto- flow. Probabilistic modelling of wind flow can aid trajectory ries. Airflow pattern information allow to plan energy efficient planning of a robot during exploration. paths for flying robots and improve gas distribution mapping. B. Problem statement However, modelling the motion of objects (e.g., people) and We address the problem of statistically modelling flows in flow of continuous media (e.g., air) is a challenging task. We present a probabilistic approach for general flow mapping, non-trivial environments using sparse and noisy data. which can readily handle both of these examples. Moreover, we It is rarely possible to have access to data completely present and compare two data imputation methods allowing to describing the motion in an environment, e.g., building a build dense maps from sparsely distributed measurements. The very dense sensor network to monitor wind flow is usually methods are evaluated using two different data sets: one with unfeasible or too expensive. Another problem is randomness pedestrian data and one with wind measurements. Our results show that it is possible to accurately represent multimodal, in motion observations, which can be a result of noisy turbulent flow using a set of Gaussian Mixture Models, and measurements, or an inherent feature of the motions. Both also to reconstruct a dense representation based on sparsely cases require a proper statistical analysis in order to identify distributed locations. and model the motion patterns. Our proposed Circular–Linear Flow Field map (CLiFF- I. INTRODUCTION map), introduced in Sec III-A, represents motion patterns A. Motivation using multimodal statistics to represent speed and orientation Many robotics applications can benefit from maps going jointly. The approaches presented in Sec III-D allow to beyond mere occupancy by explicitly modelling the motion reconstruct a dense map from spatially and temporally sparse data. in an environment. To allow robots to operate efficiently and C. Contribution in an acceptable way among people, we have to provide The contributions of the paper are as follows. methods to both map and exploit information about motion First, we describe CLiFF-map, a probabilistic framework patterns. Motion pattern information can be useful for many for mapping velocity observations independently from their tasks; e.g., task and motion planning, object tracking and underlying physical processes. In this way we gain generality intention recognition. These tasks are relevant for areas as that allows to build a comprehensive and coherent model. diverse as Human Robot Interaction (HRI) and Mobile Robot The model consists of a set of Gaussian mixture models Olfaction (MRO). (GMMs) representing local observations, and variables de- In HRI, information about how people usually move scribing the confidence in each part of the model, as well as should affect the robot’s motion planning. A motion planner the likelihood of the motion. In contrast to other methods, can use this knowledge to build socially more acceptable and CLiFF-map represents speed and orientation in a joint space. predictable paths [12]. Motion patterns implicit embed in- This allows to properly address multimodality in the data. formation about traffic rules and also mark frequented paths. Second, we investigate two data imputation methods for Such information will help to improve motion planning in generating maps from sparsely distributed measurements. complex environments, as demonstrated in [16, 17], and Third, we present a validation of the representation and allow robots to move in a safer way. the quality of map reconstruction using real world data with Similarly, in MRO and environmental monitoring, statis- the k-NN divergence estimator from [25]. tics about airflow can facilitate better understanding of gas II. RELATED WORK distributions [20]. Gas plumes are heavily influenced by the Our work is connected to the field of mapping of dynamics, This work was funded in part by the EU FP7 project SPENCER (ICT- which is different from dynamic mapping (creating a geomet- 2011-600877) and H2020-ICT projects SmokeBot (645101) and ILIAD ric map in a changing environment). Recently, a number of (732737), the Swedish Knowledge Foundation projects AIR (20140220) and approaches for mapping of dynamics have been developed. RAISE (20130196). 1The authors are with the MRO lab of the AASS research centre at One general approach is to store past observations in Orebro¨ University, Sweden. [email protected] a compressed way (e.g., work of Arbuckle et al. [1] and Mitsou et al. [14]). Such methods provide tools to analyse the plications. Instead, CLiFF-map uses the idea of a Semi- history of the environment, but they are not able to predict Wrapped Gaussian Mixture Model presented in a later work how it will evolve in the future. On the other hand, work of Roy et al. [22]. A similar idea on how to jointly analyse on generative models, which try to predict future states of speed and orientation was suggested in work of Calderara et the environment, can be found in work of Wang et al. [26], al. [2], which points out that using a normal distribution on Kucner et al. [10] and Saarinen et al. [23]. All of these a line and a wrapped normal distribution allows to compute methods treat dynamics as a change of occupancy in grid a covariance matrix explaining the correlation between the map cells and most of them assume that state changes of speed and orientation. neighbouring cells are independent. In contrast, CLiFF-map In this paper we also address the data imputation prob- models the flow field of the environment and how it affects lem [11]. Data imputation is a set of methods that are used the motion of objects. to fill in missing records in a data set. We use non-parametric The related problem of analysing trajectories of moving random kernel imputation [18, 19]. In this approach missing objects has been addressed, e.g., in work of Calderara et data are drawn, according to weights computed using a kernel al. [3] and Nawaz et al. [15]. The authors try to detect function, from already computed models and then imputed similarities between trajectories and cluster them. An exten- into unobserved locations. sion of this approach, presented by Ellis et al. [7], clusters III. ALGORITHMS trajectories based on their entry point and uses a Gaussian A. Representation Process to model the observed motion patterns. The shared limitation of the trajectory analysing methods listed above is Velocity V can be described as a heterogeneous quantity that they cannot work with inputs providing only velocity combining orientation (θ) and speed (ρ), (see Eq. 1). In con- estimates or incomplete data. Moreover, they rely on the trast to a representation using a 2D Euclidean vector (Vx;Vy), assumption of complete observability, which is difficult to in this representation each component has a physical meaning ensure for large environments (e.g., airports). Furthermore, and can be analysed separately, and the covariance matrix has the work of Ellis et al. [7] requires to build a separate model a clear physical interpretation. T + for each entry point, which can lead to a complex multilevel V = (θ; ρ) ; ρ R θ [0; 2π) (1) 2 ^ 2 representation. They also do not address the problem of To build a probabilistic model of velocities we use a semi- multimodality, assuming that intersections will only happen wrapped normal distribution (SWND), which is a distribu- between trajectories with different entry points. tion on a cylinder. One of the dimensions is wrapped around Joseph et al. [9] describe trajectories as flow fields where the circumference of it and the other one is along its height. each position has a corresponding path derivative. The motion in the environment is modelled as a mixture of SW X θ k (V ) = µµ,Σ + 2π (2) Gaussian Processes. The major difference between CLiFF- Nµµ,Σ N Σ ρ 0 map and the work of Joseph et al. is that CLiFF-map does not k2Z depend on the knowledge of trajectories. CLiFF-map aims In Eq. 2 we see that density function of a semi-wrapped to address a wide spectrum of problems focusing on cases normal distribution is a periodic sum of period 2π of normal where trajectory information is not available (air flow) or distributions on a surface defined by (µµ,ΣΣ).
Details
-
File Typepdf
-
Upload Time-
-
Content LanguagesEnglish
-
Upload UserAnonymous/Not logged-in
-
File Pages9 Page
-
File Size-