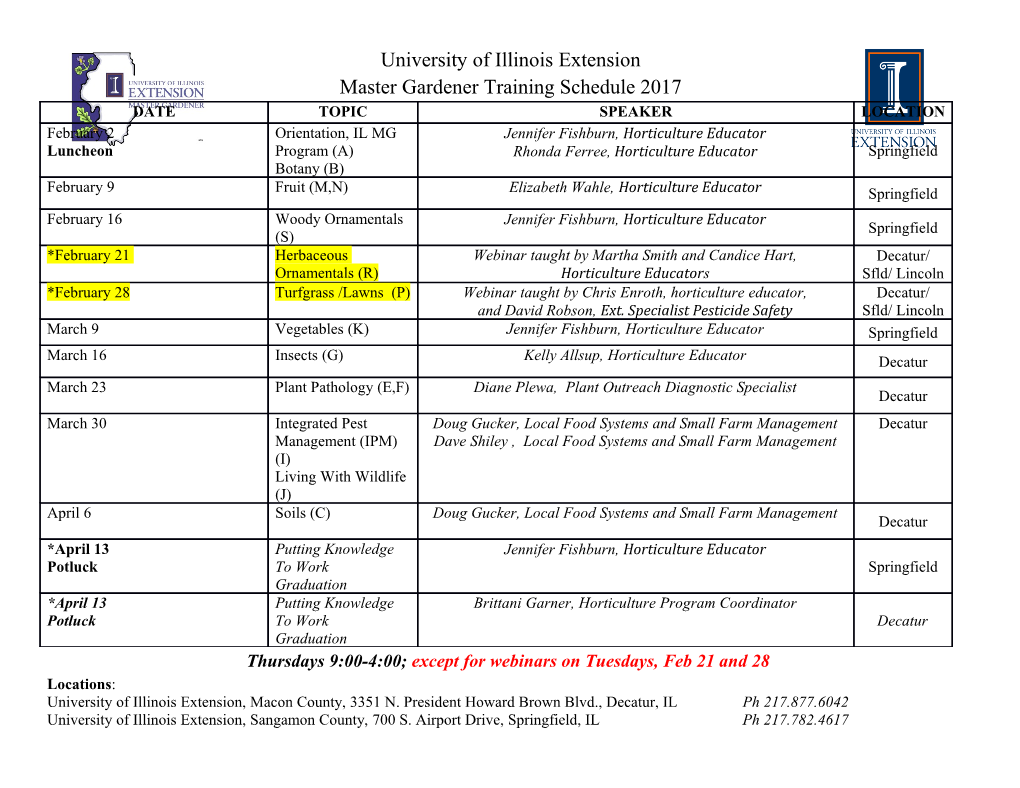
ISSN (Print) : 0974-6846 Indian Journal of Science and Technology, Vol 10(20), DOI: 10.17485/ijst/2017/v10i20/115033, May 2017 ISSN (Online) : 0974-5645 Dialect Identification of Assamese Language using Spectral Features Tanvira Ismail* and L. Joyprakash Singh Department of Electronics and Communication, School of Technology, North-Eastern Hill University, NEHU Campus, Shillong – 793022, Meghalaya, India; [email protected] Abstract Objective: telemedicine Accurate which isdialect especially identification important technique for older helps and homebound in improving people. the speech Methods: recognition In this systemspaper we that have exist developed in most of the present day electronic devices and is also expected to help in providing new services in the field of e-health and Indian language) and two of its dialects, viz., Kamrupi and Goalparia. Findings: the speech corpora needed for the dialect identification purpose and described a method to identify Assamese language (an Research work done on dialect identification tois relativelyextract the much spectral less thanfeatures that ofon thelanguage collected identification speech data. for Two which modeling one of the techniques, reasons being namely, dearth Gaussian of sufficient Mixture database Model andon dialects. Gaussian As Mixture mentioned, Model we with have Universal developed Background the database Model and havethen Mel-Frequencybeen used as the Cepstral modeling Coefficient techniques has tobeen identify used the dialects and language. Novelty: So far, standard speech database for Assamese dialects that can be used for speech processing research has not been made. In this paper, we not only describe a method to identify dialects of the Assamese language, but have also developed the speech corpora needed for the dialect identification purpose. Keywords: Assamese, Gaussian Mixture Model, Gaussian Mixture Model with Universal Background Model, Goalparia, Kamrupi, Mel-Frequency Cepstral Coefficient 1. Introduction • Improving certain applications and services such as Speech Recognition Systems which exist in Dialect is defined as a variety of a language spoken in a most of the today’s electronic devices, particular geographical area that is distinguished from • Enhancing the human - computer interaction other varieties of the same language by features of pro- applications and nunciation, grammar and vocabulary. It is also defined as • Securing the remote access communication.2 a variety of speech that differs from the standard language normally designated as the official language. Moreover, accurate dialect detection technique is Humans are born with the ability to discriminate expected to help in providing new services in the field of between spoken languages as part of human intelligence e-health and telemedicine which is especially important and the quest to automate such ability has never stopped.1 for older and homebound people.2 Just like any other artificial intelligence technologies, auto- Although much research work has been done in matic dialect identification aims to replicate such human language identification, the problem of dialect identifi- ability through computational means.1 Dialect identifica- cation, which is very similar to the problem of language tion is the task of recognizing a speaker’s regional dialect identification, has not received the same level of research within a predetermined language. interest.3 Research in the field of dialect is still limited Developing a good method to detect dialect accu- due to the dearth of databases and the time consuming rately helps in analysis process.4 Initial work using Gaussian Mixture *Author for correspondence Dialect Identification of Assamese Language using Spectral Features Models (GMM) was performed for the identification Split and Merge Expectation Maximization Algorithm of twelve languages and dialect identification was also was tested on four Indian languages, viz, Hindi, Telugu, performed for three out of the twelve languages, viz., Gujarati and English.10 Four Indian languages, viz, Hindi, English, Mandarin and Spanish.3 Identification capabil- Bengali, Oriya and Telugu were identified by consider- ity was improved by using Gaussian Mixture Model with ing the special CV (Consonant-Vowel) behavior of the Universal Background Model (GMM-UBM) and it was language in their syllables and were also analyzed using showed that a GMM-UBM based model provides good the SVM classifier.11 Work was also done on language results for recognition of American vs. Indian English, identification that was speaker dependent and was tested four Chinese dialects and three Arabic dialects.5 on three Indian languages, viz., Assamese, Hindi and In the field of Indian languages, Mel-Frequency Indian English, based on clustering and supervised learn- Cepstral Coefficient (MFCC) and Speech Signal based ing.12 First the feature vectors using LP coefficients were Frequency Cepstral Coefficient (SFCC) feature extrac- obtained and clusters of vectors using the K-means algo- tion techniques along with GMM and Support Vector rithm were formed. Supervised learning was then used Machine (SVM) modeling techniques was used to for recognizing the probable cluster to which the test identify the two main language families of India, viz., speech sample belongs.12 Indo-Aryan and Dravidian, which in total consisted of 22 As far as Indian dialects are concerned, both spec- languages.6 The Indo-Aryan family consisted of 18 lan- tral features and prosodic features were used to identify guages, viz., Assamese, Bengali, Bhojpuri, Chhattisgarhi, five Hindi dialects, viz., Chattisgharhi, Bengali, Marathi, Dogri, English, Gujarati, Hindi, Kashmiri, Konkani, General and Telugu using Autoassociative Neural Manipuri, Marathi, Nagamese, Odia, Punjabi, Sanskrit, Network (AANN) models.13 For each dialect, their data- Sindhi and Urdu, while Dravidian family consisted of 4 base consisted of data from 10 speakers speaking in languages, viz., Kannada, Malayalam, Tamil and Telugu.6 spontaneous speech for about 5-10 minutes resulting A language identification system with two levels that used in a total of 1-1.5 hours.13 On the other hand, speaker acoustic features, was modeled using Hidden Markov identification of specific dialect, viz., Assamese was done Model (HMM), GMM and Artificial Neural Network using features obtained from various speaker dependent (ANN) and was tested on nine Indian languages, viz., parameters of voiced speech and then ANN based classi- Tamil, Telugu, Kannada, Malayalam, Hindi, Bengali, fiers were used for identification purpose.14 Marathi, Gujarati and Punjabi.7 In this two level language Thus, with regard to Indian languages vis-à-vis Indian identification system, the family of the spoken language dialects also, the dialects have not been explored as is identified in the first level and after feeding this input much as Indian languages. Therefore, the present focus to the second level the identification of a particular lan- of our research is on Indian dialects identification and guage is made in the corresponding family.7 On the other in this paper we have chosen for our study the dialects hand, both spectral features and prosodic features were of Assamese language. Assamese has mainly three dia- used for analyzing the specific information with regards lects, viz., the standard dialect, Kamrupi and Goalparia to each language present in speech and then GMM was dialects.15 The standard dialect is the standard Assamese applied for identification purposes on the Indian language language which is an Indo-Aryan language designated as speech database (IITKGP-MLILSC) which consists of as the official language of Assam state located in the north- many as 27 Indian languages.8 Concentrating more on eastern part of India. In Assam, Assamese is the formal South Indian languages (languages of Dravidian family), written language used for education, media and other a Language Identification (LID) system was presented official purposes, while Kamrupi and Goalparia are the that worked for the four South Indian languages, viz., informal spoken dialects spoken in the Kamrup and Kannada, Malayalam, Tamil and Telugu and one North Goalparia districts of Assam, respectively. The impor- Indian language, viz., Hindi in which each language was tance of Kamrupi dialect can be understood from the modeled using an approach based on Vector Quantization, early literature, wherein Kamrupi was documented as the whereas the speech was segmented into dierrant sounds first ancient Aryan literary language spoken both in the and the performance of the system on each of the seg- Brahmaputra valley of Assam and North Bengal.16-18 On ments was studied.9 An LID system using GMM for the the other hand, the importance of Goalparia, which is also features that were extracted was further modeled using an Indo-Aryan dialect, is evident from the fact that it has 2 Vol 10 (20) | May 2017 | www.indjst.org Indian Journal of Science and Technology Tanvira Ismail and L. Joyprakash Singh a rich culture of folk music known as Goalparia Lokgeet. 2.1 Development of Speech Corpora According to the 2011 Census of India, there are about 20, While building a speech corpus, the important criteria to 6 and 1 million speakers of Assamese language, Kamrupi be kept in mind are: dialect and Goalparia dialect, respectively. • Enough speech must be recorded from enough So far, standard speech database for Assamese dia- speakers in order to validate an experiment lects that
Details
-
File Typepdf
-
Upload Time-
-
Content LanguagesEnglish
-
Upload UserAnonymous/Not logged-in
-
File Pages7 Page
-
File Size-