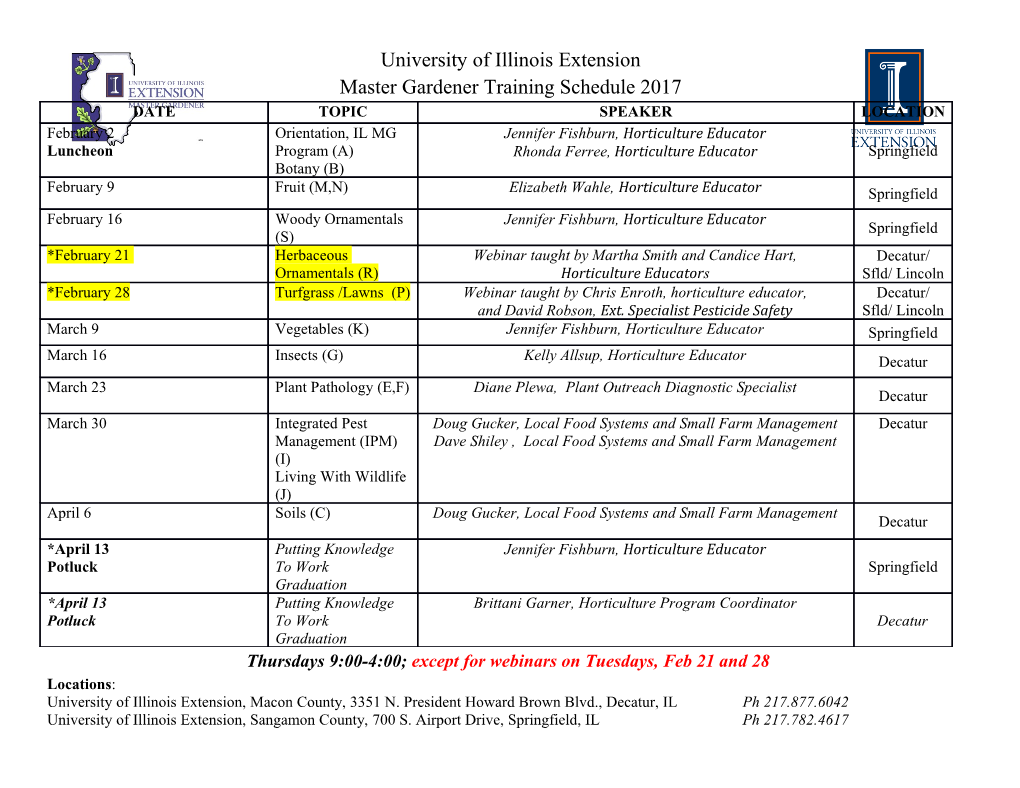
Towards End-to-End In-Image Neural Machine Translation Elman Mansimov1;∗ Mitchell Stern2;∗ Mia Chen3 Orhan Firat3 Jakob Uszkoreit3 Puneet Jain3 1New York University, 2UC Berkeley,3Google Research, ∗Equal Contribution, [email protected], [email protected] Abstract pelling test-bed for both research and engineering In this paper, we offer a preliminary investiga- communities for a variety of reasons. Although tion into the task of in-image machine trans- there are existing commercial products that ad- lation: transforming an image containing text dress this problem such as image translation fea- in one language into an image containing the ture of Google Translate1 the underlying techni- same text in another language. We propose an cal solutions are unknown. By leveraging large end-to-end neural model for this task inspired amounts of data and compute, end-to-end neu- by recent approaches to neural machine trans- ral system could potentially improve overall qual- lation, and demonstrate promising initial re- ity of pipelined approaches for image translation. sults based purely on pixel-level supervision. We then offer a quantitative and qualitative Second, and arguably more importantly, working evaluation of our system outputs and discuss directly with pixels has the potential to sidestep some common failure modes. Finally, we con- issues related to vocabularies, segmentation, and clude with directions for future work. tokenization, allowing for the possibility of more 1 Introduction universal approaches to neural machine transla- tion, by unifying input and output spaces via pix- End-to-end neural models have emerged in recent els. years as the dominant approach to a wide variety Text preprocessing and vocabulary construction of sequence generation tasks in natural language has been an active research area leading to work on processing, including speech recognition, machine investigating neural machine translation systems translation, and dialog generation, among many operating on subword units (Sennrich et al., 2016), others. While highly accurate, these models typi- characters (Lee et al., 2017) and even bytes (Wang cally operate by outputting tokens from a prede- et al., 2019) and has been highlighted to be one termined symbolic vocabulary, and require inte- of the major challenges when dealing with many gration into larger pipelines for use in user-facing languages simultaneously in multilingual machine applications such as voice assistants where neither translation (Arivazhagan et al., 2019), and cross- the input nor output modality is text. lingual natural language understanding (Conneau In the speech domain, neural methods have et al., 2019). Pixels serve as a straightforward way recently been successfully applied to end-to-end to share vocabulary among all languages at the ex- speech translation (Jia et al., 2019; Liu et al., 2019; pense of being a significantly harder learning task Inaguma et al., 2019), in which the goal is to for the underlying models. translate directly from speech in one language to speech in another language. We propose to study In this work, we propose an end-to-end neu- the analogous problem of in-image machine trans- ral approach to in-image machine translation that lation. Specifically, an image containing text in combines elements from recent neural approaches one language is to be transformed into an im- to the relevant sub-tasks in an end-to-end differ- age containing the same text in another language, entiable manner. We provide the initial problem removing the dependency of any predetermined definition and demonstrate promising first qualita- symbolic vocabulary or processing. tive results using only pixel-level supervision on the target side. We then analyze some of the errors Why In-Image Neural Machine Translation ? In-image neural machine translation is a com- 1blog.google/translate/instant-camera-translation 70 Proceedings of the First International Workshop on Natural Language Processing Beyond Text, pages 70–74 Online, November 20, 2020. c 2020 Association for Computational Linguistics http://www.aclweb.org/anthology/W23-20%2d made by our models, and in the process of doing Then, the compressed representation is used as so uncover a common deficiency that suggests a the input to a convolutional decoder that aims path forward for future work. to predict all target pixels in parallel. Decoder outputs the probabilities of each pixel p(Y ) = 2 Data Generation QH QW i=1 j=1 softmax(dec(henc)). The convolu- To our knowledge, there are no publicly avail- tional encoder consists of four residual blocks with able datasets for the task of in-image machine the dimensions shown in Table1, and the con- translation task. Since collecting aligned natural volutional decoder uses the same network struc- data for in-image translation would be a difficult ture in reverse order, composing a simple encoder- and costly process, a more practical approach is decoder architecture with a representational bot- to bootstrap by generating pairs of rendered im- tleneck. We threshold the grayscale value of each ages containing sentences from the WMT 2014 pixel in the groundtruth output image at 0.5 to ob- German-English parallel corpus. The dataset con- tain a binary black-and-white target, and use a bi- sists of 4.5M German-English parallel sentence nary cross-entropy loss on the pixels of the model pairs. We use newstest-2013 as a development output as our loss function for training. set. For each sentence pair, we create a minimal web page for the source and target, then render Dimensions In Out Kernel Stride 2 each using Headless Chrome to obtain a pair of (1024, 32) 3 64 3 1 images. The text is displayed in a black 16-pixel (1024, 32) 64 128 3 2 sans-serif font on a white background inside of a fixed-size 1024x32-pixel frame. For simplicity, all (512, 16) 128 128 3 1 sentences are vertically centered and left-aligned (512, 16) 128 256 3 2 without any line-wrapping. The consistent posi- (256, 8) 256 256 3 1 tion and styling of the text in our synthetic dataset (256, 8) 256 512 3 2 represents an ideal scenario for in-image transla- (128, 4) 512 512 3 1 tion, serving as a good test-bed for initial attempts. (128, 4) 512 512 3 2 Later, one could generalize to more realistic set- tings by varying the location, size, typeface, and Table 1: The parameters of our convolutional encoder perspective of the text and by using non-uniform network. Each block contains a residual connection backgrounds. from the input to the output. The decoder network uses the same structure in reverse. Dimensions correspond 3 Model to the size of image. In, out, kernel and stride corre- Our goal is to build a neural model for the in- spond to conv layer hyperparameters. image translation task that can be trained end-to- end on example image pairs (X∗;Y ∗) of height In order to solve the proposed task, this baseline and width H and W using only pixel-level su- must address the combined challenges of recog- pervision. We evaluate two approaches for this nizing and rendering text at a pixel level, captur- task: convolutional encoder-decoder model and ing the meaning of a sentence in a single vector as full model that combines soft versions of the tra- in early sequence-to-sequence models (Sutskever ditional pipeline in order to arrive at a modular yet et al., 2014), and performing non-autoregressive fully differentiable solution. translation (Gu et al., 2018). Although the model can sometimes produce the first few words of the 3.1 Convolutional Baseline output, it is unable to learn much beyond that; see Inspired by the success of convolutional encoder- Figure1 for a representative example. decoder architectures for medical image segmen- 3.2 Full Model tation (Ronneberger et al., 2015), we begin with To better take advantage of the problem struc- a U-net style convolutional baseline. In this ver- ture, we next propose a modular neural model that sion of the model, the source image X∗ is first breaks the problem down into more manageable compressed into a single continuous vector h enc sub-tasks while still being trainable end-to-end. using a convolutional encoder h = enc(X∗). enc Intuitively, one would expect a model that can suc- 2developers.google.com/headless-chrome cessfully carry out the in-image machine trans- 71 Output Target Image The president gave a speech Convolutional Decoder Self-Attention Encoder Figure 1: Example predictions made by the baseline Convolutional Encoder Convolutional Encoder convolutional model from Section 3.1. We show two pairs of groundtruth target images followed by gener- ated target images. Although it successfully predicts Der Präsident hielt heute eine Rede. The president gave a one or two words, it quickly devolves into noise there- Source Image Input Target Image after. Figure 2: One decoding step for our full model on an example German-English in-image translation pair. lation task to first recognize the text represented The model can be viewed as a fully differentiable ana- in the input image, next perform some computa- log of the more traditional OCR ! translate ! render tion over its internal representation to obtain a soft pipeline. translation, and finally generate the output image through a learned rendering process. Moreover, tence into sentence pieces, and decompose each just as modern neural machine translation systems example into one sub-example per sentence piece. predict the output over the span of multiple time The nth sub-example has a target-side input im- steps in a auto-regressive way rather than all at age consisting of the first n − 1 sentence pieces, once, it stands to reason that such a decomposition and is trained to predict an output image consist- would be of use here as well.
Details
-
File Typepdf
-
Upload Time-
-
Content LanguagesEnglish
-
Upload UserAnonymous/Not logged-in
-
File Pages5 Page
-
File Size-