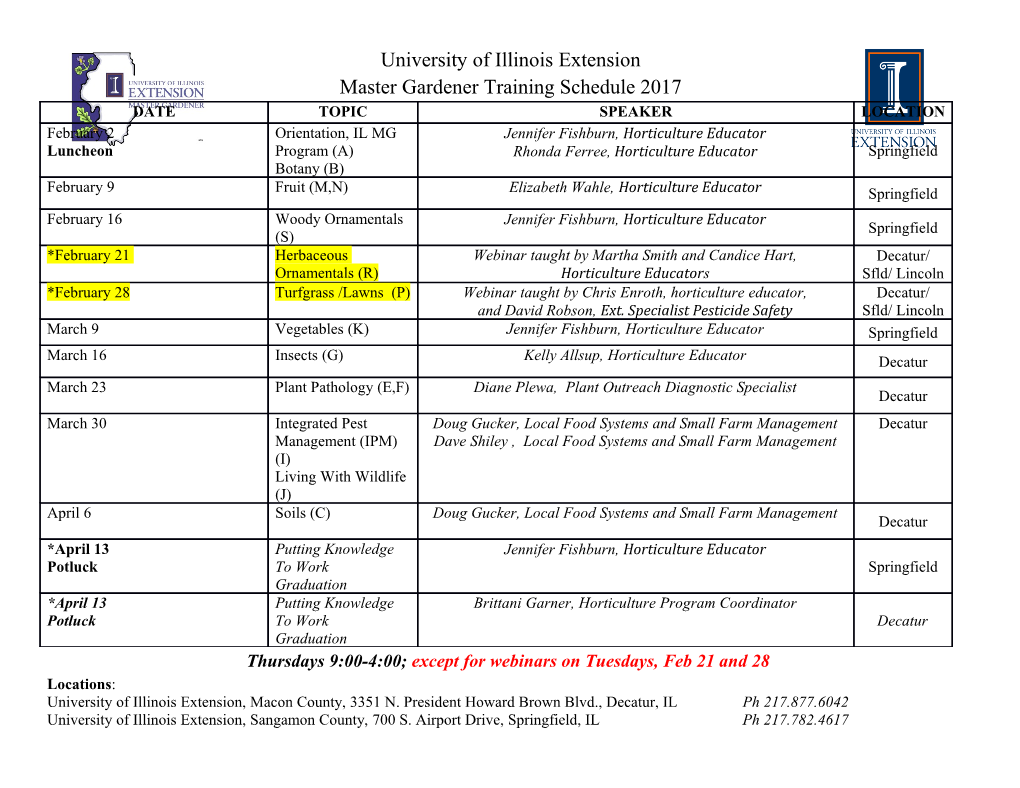
LARGEST CENTER-SPECIFIC MARGIN FOR DIMENSION REDUCTION Jian’an Zhang Yuan Yuan Feiping Nie Qi Wang School of Computer Science and Center for OPTical IMagery Analysis and Learning (OPTIMAL), Northwestern Polytechnical University, Xi’an 710072, Shaanxi, PR China ABSTRACT 6DPSOHVIURPLWKFODVV Dimensionality reduction plays an important role in solving 6DPSOHVIURPMWKFODVV the “curse of the dimensionality” and attracts a number of 5HODWLRQVKLSVEHWZHHQ FHQWHUVDQGVDPSOHV researchers in the past decades. In this paper, we proposed 5HODWLRQVKLSVEHWZHHQ a new supervised linear dimensionality reduction method FHQWHUV named largest center-specific margin (LCM) based on the in- tuition that after linear transformation, the distances between Fig. 1. Diagram for the intuition, circles and triangles should the points and their corresponding class centers should be be close to their diamond centers and the distances between small enough, and at the same time the distances between d- different diamond centers should be as large as possible. ifferent unknown class centers should be as large as possible. On the basis of this observation, we take the unknown class centers into consideration for the first time and construct an optimization function to formulate this problem. In addition, we creatively transform the optimization objective function methods do not utilize the information of class centers. There- into a matrix function and solve the problem analytically. fore, supervised method with class centers’ information can Finally, experiment results on three real datasets show the be taken into consideration and applied into dimensionality competitive performance of our algorithm. reduction. Index Terms— Dimensionality Reduction, LCM, Center- In this work, we proposed a new linear dimensionality re- specific Method duction method on the basis of the observation that after lin- ear transformation the distances between the points and their corresponding class center should be small enough, and at 1. INTRODUCTION the same time the distances between different unknown class centers should be as large as possible. It will be clearer to Dimensionality reduction plays an important role in solving understand the above idea from Fig. 1. the “curse of the dimensionality”. Directly working on high dimensional data is not only time consuming but also compu- From Fig.1, it can be found that the class centers’ infor- tationally unreliable. So a great effort has been put in the past mation is of importance to the dimensionality reduction. So in decades and many classical algorithms have been proposed. this work, for the first time, we take the unknown class cen- A good review of these algorithms can be referenced from ters that generate after linear transformation into considera- [1] [2] [3]. In addition, new ideas and methods can be further tion. And based on the relationships showed in Fig.1, we con- referenced from [4] [5] [6] [7] [8] [9] [10] [11] [12] struct an optimization objective function with the variables of Traditional dimensionality reduction algorithms can be the transformation matrix A and the unknown class centers grouped into two classes, unsupervised ones and supervised y1, y2, ··· , yn to formulate this intuition. Furthermore, we ones. A great number of these methods belong to unsuper- creatively convert the initial objection function into a matrix vised ones such as principal components analysis (PCA [13]), function which is more prone to analysing and solving the however, compared with supervised methods, unsupervised problem. Moreover, we study the objective function inten- methods cannot make full use of the samples’ potential. On sively and improve the objective function by imposing two the other hand, most of traditional dimensionality reduction regular terms making it a convex function, and at the same time the meanings of the formulation are reserved. At last, Qi Wang and Feiping Nie are the corresponding authors. This work is we get the transformation matrix by solving the optimization supported by the National Natural Science Foundation of China under Grant 61379094 and Natural Science Foundation Research Project of Shaanx- problem and the low-dimensional transformed data can be ac- i Province under Grant 2015JM6264. quired by multiplying the transformation matrix. − 1 T 2. METHODOLOGY L = I c 11 , which is the centering matrix, I stands for identity matrix with dimensionality of c, and 1 stands for the In this section, we introduce a new linear dimensionality re- c-dimensional vector with all elements being 1. Note that, k − k2 − − T duction method named largest center-specific margin(LCM). AX YC F = tr(AX YC)(AX YC) , so Eq. (2) As a definition, linear dimensionality means, given n d- can be simplified into the following form d×n dimensional data points X = [x1; x2; :::; xn] 2 R and a choice of dimensionality r < d, optimize some objective min tr(AXXT AT ) − 2tr(YT AXCT ) · 2 r×d A;Y fX( ) to produce a linear transformation A R , and (3) 2 r×n 1 call Y = AX R the low-dimensional transformed + tr(Y(CCT − I + 11T )YT ): data. Next,we introduce a new method to optimize the linear c transformation matrix A Nevertheless, the optimal problem described in Eq.(1) is We build on the simple intuition that after linear transfor- not guaranteed to be convex. For the convenience of tracking, mation the distances between the points of the same label and the original problem can be reformulated as follow, i.e. add their corresponding class center should be small enough, and two regular terms to the objective function at the same time the distances among unknown centers of dif- X X X X ferent classes should be as large as possible. As shown in Fig. 2 2 min kAxi − yjk − kyi − yjk (1), we can take the class centers’ information into consider- A;y ;y ;:::;y 1 2 n 6 ation and establish the relationships between points and their i j j cj=ci k k2 k k2 unknown centers as well as the relationships among the corre- + γ A F + η Y F : sponding centers. From the basic intuition, we can formulate (4) the idea as below Note that, after modifying, the new optimal Eq. (4) is jointly X X X X convex with regard to A and Y, hence this optimal problem 2 2 min kAxi−yjk − kyi−yjk ; (1) has globally optimal solution. Moreover, even if adding two A;y1;y2;:::;yc i j j cj=6 ci regular terms to the original optimal Eq. (1), the significance of the problem is not changed because the above regular terms where xi; i = 1; 2; :::; n are the feature representations of in- are equivalent to imposing constraint to A and Y so that the stances of different classes and yj; j = 1; 2; :::; c are the un- norms of A and Y are not too large. known class centers. In Eq. (1), the first term implies that af- In the same manner, we can convert the Eq.(4) into the ter linear transformation A, the distances between the points following matrix form based on the Eq.(3) and the property and their corresponding unknown center of the same class, of trace operator. and the second term represents the distance between two un- known centers of different class. In intuition, in order to ac- min tr(ANAT ) − 2tr(YT AXCT ) + tr(YKYT ); (5) quire an effective transformation matrix, the first term should A;Y be as small as possible and in contrast the second term should where be as larger as possible. So we transform the second term to T the minus term making it a unified optimal problem. N = XX + γI; (6) Eq. (1) can be transformed into the following matrix form 1 after permutation and combination of the terms K = CCT + (η − 1)I + 11T ; (7) c k − k2 − T min AX YC F tr(YLY ); (2) and γ > 0, η > 1. A;Y From now on, the optimization objective function has been established. So the next task is to solve the optimal Eq. where k · kF is Frobenius norm, tr(·) stands for the trace op- erator. m is the dimensionality of feature vector and n rep- (5). It is noted that Eq. (6) is continuous with regard to A resents the number of all training samples. A 2 Rm×m is a and Y, hence it can be solved by taking the derivation of linear transform matrix and X 2 Rd×n stands for the sam- one of the variables when fixed the other one and letting the ple matrix which consist of all the training samples and each derivation be 0. By fixing Y , we get the derivation of Eq.(5) − column stands for a feature vector of an instance of one class. w.r.t A, this is AN YCX = 0, and hence we can get Y = [y ; y ; :::; y ] 2 Rm×c 1 2 c is the matrix synthesized by T −1 the centers of c classes. C 2 Rc×n is a matrix with the fol- A = YCX N : (8) lowing form By fixing A, we get the derivation of Eq.(5) w.r.t Y, this is 0 1 T 1 ··· 1 0 ············ 0 YK − AXC = 0, and hence we can get B ··· ··· ··· C B 0 0 1 1 0 0 C − C = @ ··························· A Y = AXCT K 1: (9) 0 ············ 0 1 ··· 1 Algorithm 1 LCM Algorithm for Dimensionality Reduction show the results. We can see that our LCM algorithm always Input: projects the high dimensional data points into a linear mani- The n training samples with corresponding labels fold and at the same time maintains the property of clustering, n (xi; yi)i=1 which is a powerful tool for analysing the high dimensional Output: data points. Compared with LCM, traditional dimensionality The transformation matrix A reduction methods such as PCA and LLE do not maintain the 1: Initialize parameters γ, η, error bound ", class numbers c, special shapes in low-dimensional space.
Details
-
File Typepdf
-
Upload Time-
-
Content LanguagesEnglish
-
Upload UserAnonymous/Not logged-in
-
File Pages5 Page
-
File Size-