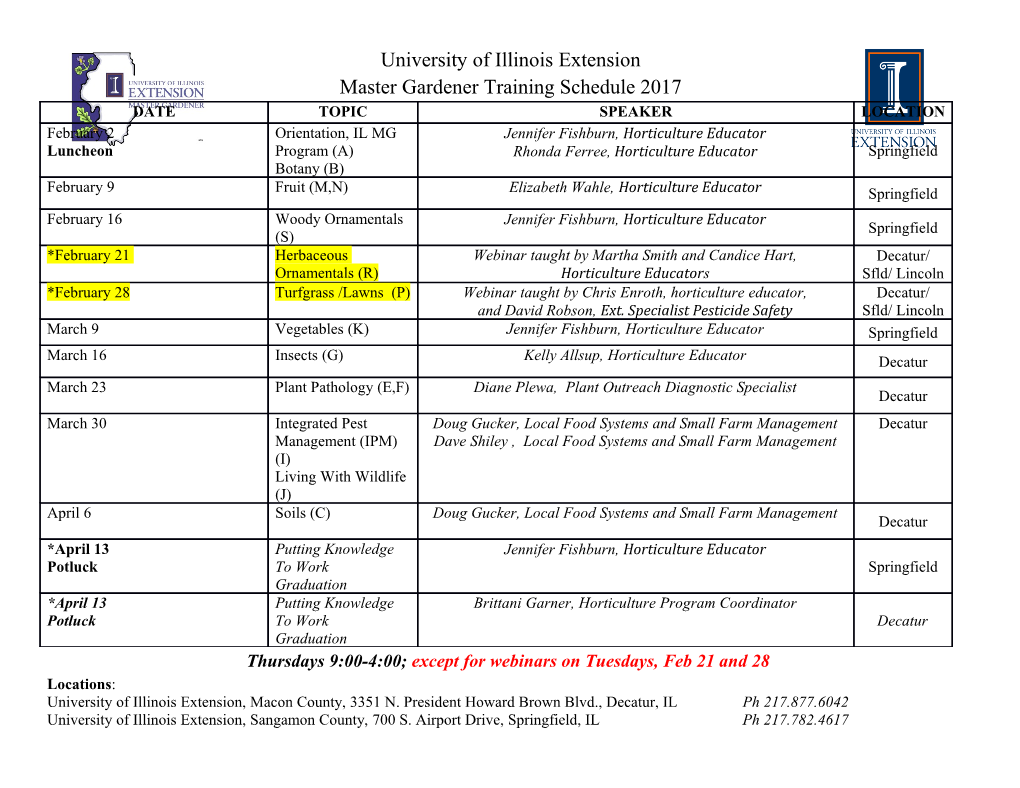
THURSDAY APRIL 16 001. Chan Zuckerberg Fellows 8:00 to 5:00 pm Hilton San Francisco Union Square: Yosemite A Chan Zuckerberg Fellows 002. Classroom Assessment Task Force 8:00 to 5:00 pm Hilton San Francisco Union Square: Yosemite B Classroom Assessment Task Force 003. Exploring, Visualizing, and Modeling Big Data with R Training Session 8:00 to 5:00 pm Hilton San Francisco Union Square: Franciscan Ballroom A Working with big data requires a particular suite of data analytics tools and advanced techniques, such as machine learning (ML). Many of these tools are readily and freely available in R. This full-day session will provide participants with a hands-on training on how to use data analytics tools and machine learning methods available in R to explore, visualize, and model big data. The first half of the session will focus on organizing (manipulating and summarizing) and visualizing (both statically and dynamically) big data in R. The second half will involve a series of short lectures on ML techniques (decision trees, random forest, and support vector machines), as well as hands-on demonstrations applying these methods in R. Examples will be drawn from various assessments (e.g., PISA and TIMSS). Participants will get opportunities to work through several, directed labs throughout the day. The target audience for this session includes graduate students, researchers interested in analyzing big data from large-scale assessments and surveys, and practitioners working with big data on a daily basis. Some familiarity with the R programming language is required. Participants should bring a laptop with R and RStudio installed to be able to complete the labs during the session. Session Organizers: Okan Bulut, University of Alberta Christopher D. Desjardins, St. Michael's College 004. Assessment of Intrapersonal/Interpersonal Skills for K-12, Higher Education, and the Workplace Training Session 8:00 to 5:00 pm Hilton San Francisco Union Square: Union Square 1 & 2 This full-day workshop provides training, discussion, and hands-on experience in developing methods for assessing, scoring, and reporting on interpersonal and intrapersonal skills, for K-12, higher education, and the workplace. Workshop focuses on (a) reviewing the most important skills based on current research and frameworks; (b) general methods for writing good items; (c) standard and innovative measurement methods, including self- and others’-ratings, forced-choice (rankings), anchoring vignettes, and situational judgment testing (SJT); (d) classical and item-response theory (IRT; e.g., 2PL, partial credit, nominal response model) scoring procedures; (e) reliability from classical test theory and IRT; and (f) reporting. Workshop sessions will be organized around item types (e.g., forced-choice, anchoring vignettes). Examples will be drawn from various assessments (e.g., CORE, PISA, NAEP, SuccessNavigator, FACETS). There will be hands-on demonstrations using R for scoring anchoring vignettes and SJTs, and group participation in a collaborative problem solving task. The workshop is designed for a broad audience of assessment developers and psychometricians, working in applied or research settings. Participants should bring laptops preferably with R and Rstudio installed (but help will be provided if needed, and it will be possible to participate as an observer in a group). Presenters: Jiyun Zu, Educational Testing Service Jiangang Hao, Educational Testing Service Session Organizer: Patrick Charles Kyllonen, Educational Testing Service THURSDAY APRIL 16 005. Modeling Writing Process Using Keystroke Logs Training Session 8:00 to 12:00 pm Hilton San Francisco Union Square: Union Square 15 & 16 In this half-day workshop, participants will have an opportunity to learn about and analyze a newer type of the educational data that is being progressively used in writing research; namely, the keystroke logs collected during the writing process. Information contained in the keystroke logs goes much beyond a holistic evaluation on the written product. From the keystroke logs, one may identify, for example, whether a writer had trouble with retrieving words, edited what was written before the submission, or spent sufficient time and effort on the task. As much as the opportunities and potential applications given by this type of timing and process data, it also poses many challenges to researchers and practitioners, which includes construct-relevant evidence identification from the logs, evidence extraction/feature engineering, and statistical treatment and modeling of such complex data. Students and professionals in the areas of writing research and educational measurement are invited. The format of this workshop will be a mix of lecture-style presentation, hands-on data analyses, and group discussion. Some background on statistical analyses will be preferred. Sample R codes for applying Markov or semi-Markov process and other graphical models will be provided. Participants should bring personal laptops with the statistical software R installed. Presenters: Mo Zhang, ETS Hongwen Guo, Educational Testing Service Xiang Liu, Educational Testing Service Session Organizer: Mo Zhang, ETS 006. An Intuitive Introduction to Maximum Likelihood and Bayesian Estimation for Applied Researchers. Training Session 8:00 to 12:00 pm Hilton San Francisco Union Square: Union Square 17 & 18 The standard two-course statistical methods sequence typically covers through linear regression, ANOVA, and closely related models. However, consulting a text on other common methods (e.g., logistic regression, loglinear models, factor analysis, and item response theory) goes beyond that as they are typically analyzed using maximum likelihood. Even worse for many practitioners, the use of maximum likelihood in these texts often seems to assume the reader has had two semesters of calculus based statistical theory. Similar roadblocks occur when Bayesian methods are introduced. This course, which requires no previous calculus knowledge, introduces maximum likelihood and Bayesian methods for applied researchers. Using the normal distribution, logistic regression, and ability estimation in item response theory (no experience needed!) as examples, the concepts of maximum likelihood and Bayesian estimation are explored graphically and by using participants’ laptops with easy to run interactive programs and simulations. Examples from the literature and the methods' limitations are discussed throughout the course. Session Organizer: Brian Habing, University of South Carolina 007. Optimal Test Design Approach to Fixed and Adaptive Test Construction using R Training Session 8:00 to 12:00 pm Hilton San Francisco Union Square: Union Square 19 & 20 In recent years, fixed test forms and computerized adaptive testing (CAT) forms coexist in many testing programs and are often used interchangeably on the premise that both formats meet the same test specifications. In conventional CAT, however, items are selected through computer algorithms to meet mostly statistical criteria along with other content-related and practical requirements, whereas fixed forms are often created by test development staff using iterative review processes and more holistic criteria. The optimal test design framework can provide an integrated solution for creating test forms in various configurations and formats, conforming to the same specifications and requirements. This workshop will present some foundational principles of the optimal test design approach and their applications in fixed and adaptive test construction. Practical examples will be provided along with an R package for creating and evaluating various fixed and adaptive test formats. Session Organizer: Seung Choi, The University of Texas at Austin THURSDAY APRIL 16 008. Python, Machine Learning, and Applications - A Gentle Introduction Training Session 8:00 to 12:00 pm Hilton San Francisco Union Square: Union Square 22 Machine learning is getting popular in recent years. Its applications span a vast range: from agriculture to astronomy, from business to biology, from communication to chemistry, from data mining to dentistry, from education to economy; the list goes on. The interest in machine learning continues growing as indicated by related presentations and publications. The goal of this lecture-style training is to provide a gentle introduction on this topic. Although other languages are available for machine learning, Python will be introduced as a starter in this training. The main course has two dishes, supervised machine learning and unsupervised machine learning. Dessert samples of using machine learning in educational measurement research conclude the training. Participants do not need to have any experience in machine learning or Python. Upon completion, participants are expected to have a general idea of machine learning and know how to use Python on a simple machine learning project. Participants do not need to bring their laptops or install software; the training will be as gentle as possible so that it is tasty to a broad audience. Having said this, following an example with a laptop near the end of the training would make the dessert taste sweeter. Session Organizer: Zhongmin Cui, ACT, Inc. 009. Using the R package PLmixed to Estimate Complex Measurement and Growth Models Training Session 8:00 to 12:00 pm Hilton San Francisco Union Square: Union Square 23 & 24 Latent variable modeling, including factor analysis, item response theory (IRT), and multilevel modeling, has proved
Details
-
File Typepdf
-
Upload Time-
-
Content LanguagesEnglish
-
Upload UserAnonymous/Not logged-in
-
File Pages132 Page
-
File Size-