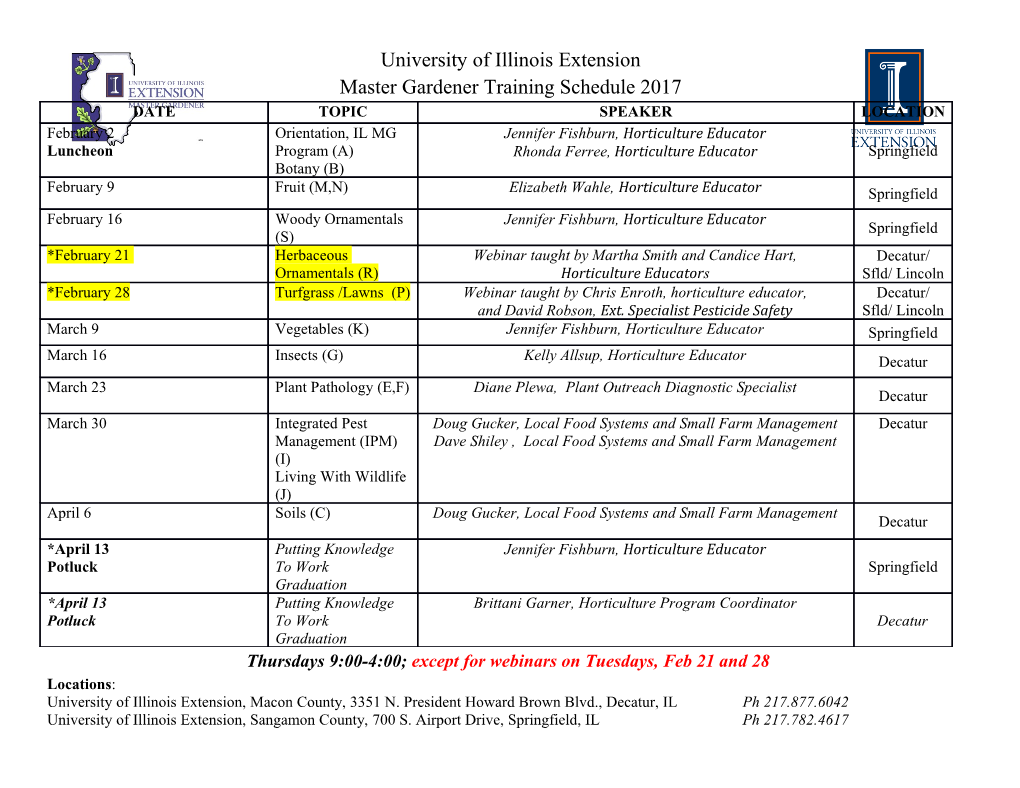
ISSN: 2469-5831 Gu et al. Int J Clin Biostat Biom 2018, 4:017 DOI: 10.23937/2469-5831/1510017 Volume 4 | Issue 1 International Journal of Open Access Clinical Biostatistics and Biometrics RESEARCH ARTICLE Partial Variable Selection and its Applications in Biostatistics Jingwen Gu1, Ao Yuan1,2*, Chunxiao Zhou2, Leighton Chan2 and Ming T Tan1* 1Department of Biostatistics, Bioinformatics and Biomathematics, Georgetown University, USA Check for updates 2Epidemiology and Biostatistics Section, Rehabilitation Medicine Department, Clinical Center, National Institutes of Health, USA *Corresponding author: Ao Yuan, Department of Biostatistics, Bioinformatics and Biomathematics, Georgetown University, Washington DC 20057, USA; Epidemiology and Biostatistics Section, Rehabilitation Medicine Department, Clinical Center, National Institutes of Health, Bethesda MD 20892, USA, E-mail: [email protected]; Ming T Tan, Department of Biostatistics, Bioinformatics and Biomathematics, Georgetown University, Washington DC 20057, USA, E-mail: [email protected] addressed variable selection in artificial neural network Abstract models, Mehmood, et al. [8] gave a review for variable We propose and study a method for partial covariates selec- selection with partial least squares model. Wang, et al. tion, which only select the covariates with values fall in their effective ranges. The coefficients estimates based on the re- [9] addressed variable selection in generalized additive sulting data is more interpretable based on the effective covari- partial linear models. Liu, et al. [10] addressed variable ates. This is in contrast to the existing method of variable se- selection in semiparametric additive partial linear mod- lection, in which some variables are selected/deleted in whole. els. The Lasso [6,11] and its variation [12,13] are used to To test the validity of the partial variable selection, we extend- ed the Wilks theorem to handle this case. Simulation studies select some few significant variables in the presence of are conducted to evaluate the performance of the proposed a large number of covariates. method, and it is applied to a real data analysis as illustration. However, existing methods only select the whole Keywords variable(s) to enter the model, which may not the most Covariate, Effective range, Partial variable selection, Linear desirable in some bio-medical practice. For example, in model, Likelihood ratio test two heart disease studies [14,15] there are more than ten risk factors identified by medical researchers in their Introduction long time investigations, with the existing variable se- lection methods, some of the risk factors will be deleted Variables selection is a common practice in biosta- wholly from the investigation, this is not desirable, since tistics and there is vast literature on this topic. Com- risk factors will be really risky only when they fall into monly used methods include the likelihood ratio test some risk ranges. Thus deleting the whole variable(s) in [1], Akaike information criterion, AIC [2] Bayesian in- this case seems not reasonable, while a more reason- formation criterion, BIC [3], the minimum description able way is to find the risk ranges of these variables, length [4,5] stepwise regression and Lasso [6], etc. The and delete the variable values in the un-risky ranges. In principal components model linear combinations of the some other studies, some of the covariates values may original covariates, reduces large number of covariates just random errors which do not contribute to the influ- to a handful of major principal components, but the ence of the responses, and remove these covariates val- result is not easy to interpret in terms of the original ues will make the model interpretation more accurate. covariates. The stepwise regression starts from the full In this sense we select the variables when their value model and deletes the covariate one by one according falls within some range. To our knowledge, method for to some statistical significance measure. May, et al. [7] this kind of partial variable selection hasn’t been seen in Citation: Gu J, Yuan A, Zhou C, Chan L, Tan MT (2018) Partial Variable Selection and its Applications in Biostatistics. Int J Clin Biostat Biom 4:017. doi.org/10.23937/2469-5831/1510017 Received: January 08, 2018: Accepted: April 12, 2018: Published: April 14, 2018 Copyright: © 2018 Gu J, et al. This is an open-access article distributed under the terms of the Creative Commons Attribution License, which permits unrestricted use, distribution, and reproduction in any medium, provided the original author and source are credited. Gu et al. Int J Clin Biostat Biom 2018, 4:017 • Page 1 of 10 • DOI: 10.23937/2469-5831/1510017 ISSN: 2469-5831 the literature, which is the goal of our study here. Note ter(s). For simple of discussion we assume there are no that in existing method of variable selection, some vari- unknown parameters. Then the log-likelihood is n ables are selected/deleted, while in our method, some = − ' β n ( β) ∑log f (yi xi ). variable(s) are partially selected/deleted, i.e., only some i=1 proportions of some variable observations are select- Let βˆ be the Maximum Likelihood Estimate (MLE) ed/deleted. The latter is very different from the exist- of β (when f (⋅) is the standard normal density, βˆ is ing methods. In summary, traditional variable selection just the least squares estimate). If we delete kd(≤ ) col- methods, such as stepwise or Lasso, some covariate(s) umns of Xn and the corresponding components of β, will be removed either wholly or none from the anal- denote the remaining covariate matrix as X− and the n ysis. This is not very reasonable, since some of the re- resulting β as β -, and the corresponding MLE as βˆ -. moved covariates may be partially effective, removing Then under the hypothesis H0 : the deleted columns of all their values may yield miss-leading results, or at least X has no effects, or equivalently the deleted compo- n cost information loss; while for the variables remaining nents of β are all zeros, then asymptotically [1]. in the model, not all their values are necessarily effec- D 2 βˆ − βˆ - → χ 2 , tive for the analysis. With the proposed method, only n ( ) n ( ) k the non-effective values of the covariates are removed, where χ 2 is the chi-squared distribution with k k and the effective values of the covariates are kept in -degrees of freedom. For a given nominal level α , let the analysis. This is more reasonable than the existing 2 χ 2 χαd (1− ) be the (1−α ) -th upper quantile of the k methods of removing all or nothing. 2 βˆ − βˆ − ≥ χ 2 (1−α ) distribution, if n ( ) n ( ) d , then H0 In the existing method of deleting whole variable(s), the validity of such selection can be justified using the is rejected at significance level α , and its not good to X Wilks result, under the null hypothesis of no effect of delete these columns of n ; otherwise we accept H0 X the deleted variable(s), the resulting two times log-like- and delete these columns of n . lihood ratio will be asymptotically chi-squared distrib- There are some other methods to select columns of uted. We extended the Wilks theorem to the case for Xn , such as AIC, BIC and their variants, as in the model the proposed partial variable deletion, and use it to jus- selection field. In these methods, the optimal deletion tify the partial deletion procedure. Simulation studies of columns of Xn corresponds to the best model selec- are conducted to evaluate the performance of the pro- tion, which maximize the AIC or BIC. These methods are posed method, and it is applied to analyze a real data not as solid as the above one, as may sometimes de- set as illustration. pending on eye inspection to choose the model which The Proposed Method maximize the AIC or BIC. = All the above methods require the models under The observed data is ( yiii,x )( 1,..., n) , where yi is d consideration be nested within each other, i.e., one is the response and xi ∈ R is the covariates, of the i -th ' a sub-model of the other. Another more general model subject. Denote y =( yy,, … ) 'and Xn =(x1n , … ,x ') '. nn1 selection criterion is the Minimum Description Length Consider the linear model (MDL) criterion, a measure of complexity, developed by yn = Xn β + εn , (1) Kolmogorov [4], Wallace and Boulton (1968) [16], etc. where ββ=( ,, … β) 'is the vector of regression pa- The Kolmogorov complexity has close relationship with 1 d the entropy, it is the output of a Markov information rameter, εn = (ε1,…,ε n )' is the vector of random errors, or residual departure from the linear model assumption. source, normalized by the length of the output. It con- verges almost surely (as the length of the output goes Without loss of generality we consider the case the εi’s are to infinity) to the entropy of the source. Let =g ( ⋅⋅, ) independently identically distributed (iid), i.e. with vari- { } ance matrix Var (ε ) = σ 2 I , where I is the n -dimensional be a finite set of candidate models under consideration, n n = = … identity matrix. When theε ’s are not iid, often it is assumed and Θ {θ j : j 1, ,h} be the set of parameters of in- i terest. θ may or may not be nested within some other Var (ε ) = Ω for some known positive-definite Ω, then make i −1/2 −1/2 −1/2 = = θj, or θi and both in Θ may have the same dimen- the transformationy n Ω yn, Xn = Ω Xn and ε Ω ε θ j sion but with different parametrization. Next consid- , then we get the model y n = Xn β + ε, and the ε ’s are iid i er a fixed density , with parameter running with Var (ε) = I .
Details
-
File Typepdf
-
Upload Time-
-
Content LanguagesEnglish
-
Upload UserAnonymous/Not logged-in
-
File Pages10 Page
-
File Size-