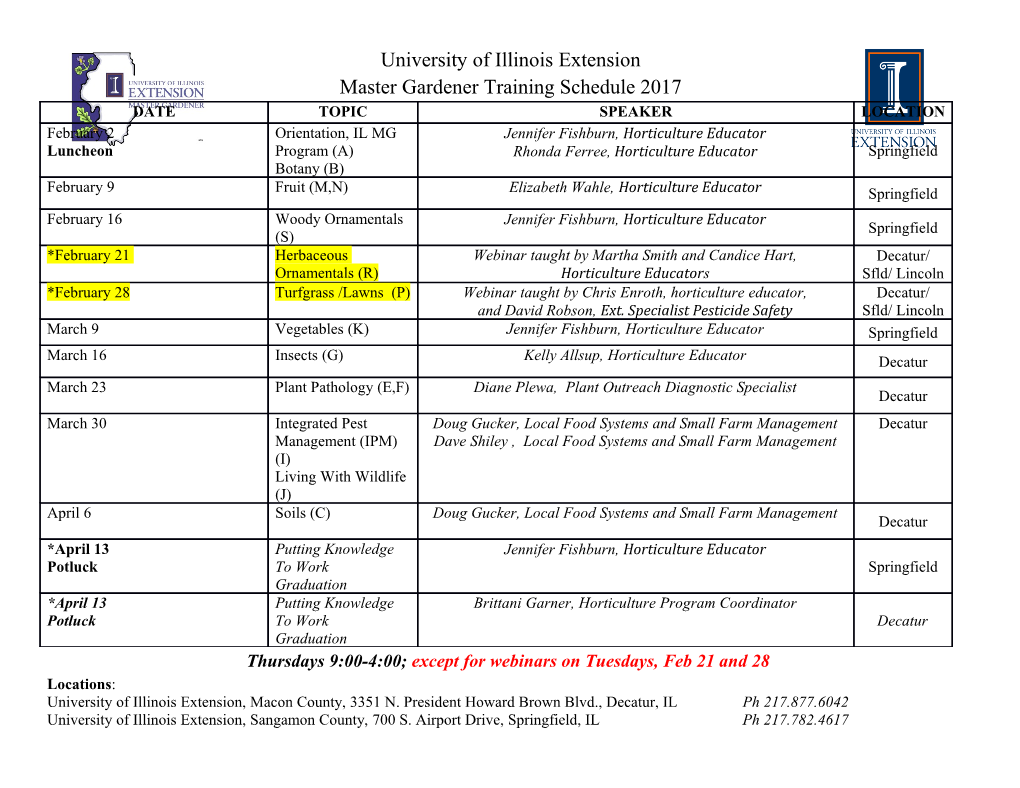
Discussion Paper | Discussion Paper | Discussion Paper | Discussion Paper | Hydrol. Earth Syst. Sci. Discuss., 7, 8809–8835, 2010 Hydrology and www.hydrol-earth-syst-sci-discuss.net/7/8809/2010/ Earth System doi:10.5194/hessd-7-8809-2010 Sciences © Author(s) 2010. CC Attribution 3.0 License. Discussions This discussion paper is/has been under review for the journal Hydrology and Earth System Sciences (HESS). Please refer to the corresponding final paper in HESS if available. Hydrological real-time modeling using remote sensing data P. Meier, A. Fromelt,¨ and W. Kinzelbach ETH Zurich,¨ Institute of Environmental Engineering (IfU), Wolfgang-Pauli Strasse 15, 8093 Zurich¨ Received: 28 October 2010 – Accepted: 2 November 2010 – Published: 10 November 2010 Correspondence to: P. Meier ([email protected]) Published by Copernicus Publications on behalf of the European Geosciences Union. 8809 Discussion Paper | Discussion Paper | Discussion Paper | Discussion Paper | Abstract Reliable real-time forecasts of the discharge can provide valuable information for the management of a river basin system. Sequential data assimilation using the Ensemble Kalman Filter provides a both efficient and robust tool for a real-time modeling frame- 5 work. One key parameter in a hydrological system is the soil moisture which recently can be characterized by satellite based measurements. A forecasting framework for the prediction of discharges is developed and applied to three different sub-basins of the Zambezi River Basin. The model is solely based on remote sensing data provid- ing soil moisture and rainfall estimates. The soil moisture product used is based on 10 the back-scattering intensity of a radar signal measured by the radar scatterometer on board the ERS satellite. These soil moisture data correlate well with the measured discharge of the corresponding watershed if the data are shifted by a time lag which is dependent on the size and the dominant runoff process in the catchment. This time lag is the basis for the applicability of the soil moisture data for hydrological forecasts. The 15 conceptual model developed is based on two storage compartments. The processes modeled include evaporation losses, infiltration and percolation. The application of this model in a real-time modeling framework yields good results in watersheds where the soil storage is an important factor. For the largest watershed a forecast over almost six weeks can be provided. However, the quality of the forecast increases significantly with 20 decreasing prediction time. In watersheds with little soil storage and a quick response to rainfall events the performance is relatively poor. 1 Introduction In hydrological forecasting, fully distributed, physically based models provide the ability to account both for the heterogeneity of a watershed and physical changes of the sys- 25 tem (e.g. induced by the construction of irrigation schemes or land use change). On the other hand simple conceptual models can provide a satisfactory performance for 8810 Discussion Paper | Discussion Paper | Discussion Paper | Discussion Paper | short term forecasts. Especially in regions with limited facilities for the measurement of relevant hydrological data this can be an advantage. Kitanidis and Bras (1980a) stated that the effective water management in a river basin system needs a reliable real-time forecast. This involves a continuous correction 5 of the forecasts based on the prediction errors of earlier forecasts. The application of a model is accompanied by several sources of errors, such as model, input and param- eter uncertainty. This leads to a deficient knowledge of the system states. Hence it is appropriate to use observed system outputs to update the states of the system (Kitani- dis and Bras, 1980a,b). 10 This so called data assimilation problem can be solved in different ways. In real- time applications a new assimilation problem is formulated at every time step. To solve this problem efficiently sequential assimilation techniques are considered su- perior (McLaughlin, 2002). Sequential assimilation algorithms, also known as filter- ing algorithms, are divided into two steps: first a propagation step, where the system 15 states are propagated through time using a model and forcing data; second an update step, where the modeled states of the system are updated based on the difference be- tween the modeled quantity and its real-world observation. To solve nonlinear filtering problems the Ensemble Kalman Filter (EnKF) has proven to be both efficient and ro- bust (Evensen, 1994). EnKF has, other than standard batch calibration, the advantage 20 of being able to incorporate a wide range of uncertainties. The uncertainties of forcing data, model parameters and modeled observations are considered separately but can be incorporated in the same mathematical scheme (Thiemann et al., 2001). As soil moisture is a key parameter in land surface hydrology, the availability of area representative measurements offers a unique opportunity to improve hydrological mod- 25 eling. The first global dataset on soil moisture has been presented by Wagner et al. (1999a). It was shown that runoff predictions were greatly enhanced when measured soil moisture both from ground measurements and from remote sensing were incorpo- rated (Aubert et al., 2003; Crow and Ryu, 2009). 8811 Discussion Paper | Discussion Paper | Discussion Paper | Discussion Paper | The assimilation of remotely sensed soil moisture data becomes more and more feasible. Several satellite missions have been launched or will be launched in the near future equipped with instruments to retrieve soil moisture using radar. These missions include the MetOp Advanced Scatterometer (ASCAT), the Soil Moisture and 5 Ocean Salinity Mission (SMOS) and NASA’s Soil Moisture Active-Passive instrument (SMAP) (Kerr et al., 2001; Naeimi et al., 2009; Piles et al., 2009). This study presents a prediction framework for river discharge based solely on re- motely sensed data of soil moisture and rainfall, a simple conceptual model and data assimilation techniques. The availability of the input data in real-time allows the model 10 to be operated in real-time, providing a prediction for discharge each time new input data are retrieved. When observation data are available the model state is updated us- ing sequential data assimilation techniques (EnKF). The update step allows the model to be relatively simple. This modeling approach is evaluated in three different sub-basins within the Zam- 15 bezi River basin (Fig. 1). The three watersheds are the Upper Zambezi upstream of the gauging station Senanga with an area of 281 000 km2, the Kafue River where the discharge is measured at the Kafue Hook Bridge with an area of 95 300 km2 and the Luangwa River which is gauged a few kilometers upstream of the confluence with the Zambezi River (142 070 km2). These watersheds cover together more than one 20 third of the whole Zambezi watershed and contribute more than one half of the total runoff at the mouth of the Zambezi, where the Upper Zambezi catchment contributes the largest amount (850 m3 s-1 mean annual discharge), the Kafue River discharges 300 m3 s-1 and the Luangwa River 700 m3 s-1. The whole Zambezi River basin lies in the semi-arid zone of southern Africa. Rainfall 25 is strongly seasonal and occurs almost exclusively between October and March. The total amount of rainfall is on average around 1000 mm yr-1, the potential evapotranspi- ration around 2000 mm yr-1. The water resources in the Zambezi river basin are more and more developed. Fea- sibility studies for several new hydro-power plants are carried out and new irrigation 8812 Discussion Paper | Discussion Paper | Discussion Paper | Discussion Paper | schemes are developed all over the river basin. While the pressure on the resources is growing, short term forecasts of the discharge can help optimizing the operation of smaller reservoirs and water abstraction schemes for irrigation without neglecting the river dependent ecosystems as a third water user. 5 2 Soil moisture The vadose zone is one of the most important components of the global water cycle. Through the coupling of water and energy fluxes soil moisture determines the local and global climate. However, the variability of soil moisture is very high in both space and time. Traditional measurement methods, such as TDR, are reasonably accurate 10 but they provide information on a very local scale. Monitoring large areas is nearly infeasible. The typical correlation length for such measurements lies between a few tens of meters and a few hundred meters (Western et al., 2004). On larger scales the temporal variation seems to be very stable since it is mainly influenced by climatic conditions (Brocca et al., 2010). Therefore remotely sensed soil moisture data provides 15 a valuable dataset for hydrological monitoring on a larger scale. There is a wide variety of techniques measuring the soil water content through re- mote sensing. However, the data can only be retrieved by indirect measurements. Both active and passive methods rely on the measurement of radiation intensities in a certain range of wavelengths. For passive systems operating in the thermal infrared 20 band the measurement target is the soil surface temperature (Verstraeten et al., 2006). The radiation intensity measured by systems operating in the microwave band is con- trolled by the dielectric constant of the top soil layer. Especially soil moisture prod- ucts based on radar satellite imagery provide an attractive
Details
-
File Typepdf
-
Upload Time-
-
Content LanguagesEnglish
-
Upload UserAnonymous/Not logged-in
-
File Pages14 Page
-
File Size-