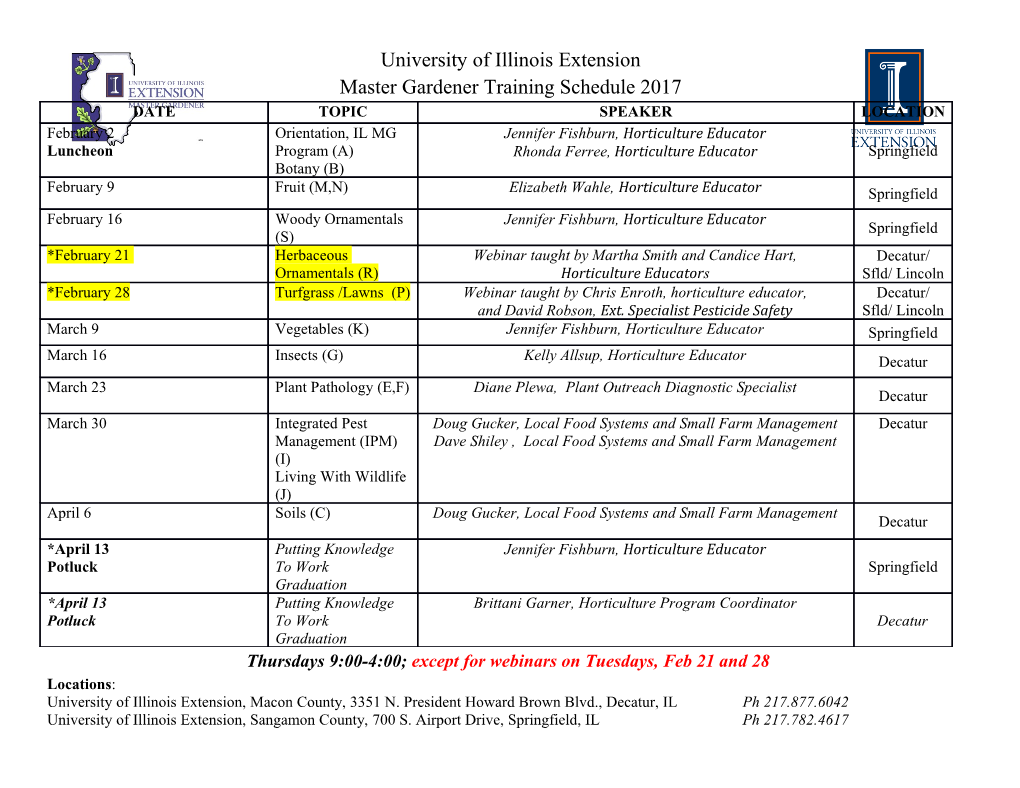
Real-time Population of Knowledge Bases: Opportunities and Challenges Ndapandula Nakashole, Gerhard Weikum Max Planck Institute for Informatics Saarbrucken,¨ Germany {nnakasho,weikum}@mpi-inf.mpg.de Abstract 2011; Nakashole 2011) which is now three years old. The NELL system (Carlson 2010) follows a Dynamic content is a frequently accessed part “never-ending” extraction model with the extraction of the Web. However, most information ex- process going on 24 hours a day. However NELL’s traction approaches are batch-oriented, thus focus is on language learning by iterating mostly not effective for gathering rapidly changing data. This paper proposes a model for fact on the same ClueWeb09 corpus. Our focus is on extraction in real-time. Our model addresses capturing the latest information enriching it into the the difficult challenges that timely fact extrac- form of relational facts. Web-based news aggrega- tion on frequently updated data entails. We tors such as Google News and Yahoo! News present point out a naive solution to the main research up-to-date information from various news sources. question and justify the choices we make in However, news aggregators present headlines and the model we propose. short text snippets. Our focus is on presenting this information as relational facts that can facilitate re- 1 Introduction lational queries spanning new and historical data. Challenges. Timely knowledge extraction from fre- Motivation. Dynamic content is an important part quently updated sources entails a number of chal- of the Web, it accounts for a substantial amount of lenges: Web traffic. For example, much time is spent read- ing news, blogs and user comments in social media. 1. Relation Discovery: We need to discover and To extract meaningful relational facts from text, sev- maintain a dynamically evolving open set of re- eral approaches have emerged (Dalvi 2009; Etzioni lations. This needs to go beyond common re- 2008; Weikum 2009). These approaches aim to con- lations such as “bornIn” or “headquateredIn”. struct large knowledge bases of facts. However, For example, major knowledge bases lack po- most knowledge bases are built in a batch-oriented tentially interesting relations like “firedFrom” manner. Facts are extracted from a snapshot of a cor- or “hasGoddaughter”. For completeness, we pus and some weeks or months later, another round need to automatically discover such relations. of facts are extracted from a new snapshot. This pro- Furthermore, we may occasionally pick up cess is repeated at irregular and long intervals result- completely new relations, such as the new no- ing in incomplete and partly stale knowledge bases. tion of a person ”unfriending” another per- If knowledge bases were updated in-sync with Web son in an online community. The TextRun- changes, time spent examining long Web articles for ner/Reverb project has addressed this challenge facts would be reduced significantly. to some extent(Banko 2007; Fader 2011) , but Current Web information extraction systems rely the output has the form of verbal phrases, rather on snapshots such as the ClueWeb09 crawl1 (Fader than typed relations and it is computed in a 1lemurproject.org/clueweb09.php/ batch-oriented manner. 41 Proc. of the Joint Workshop on Automatic Knowledge Base Construction & Web-scale Knowledge Extraction (AKBC-WEKEX), pages 41–45, NAACL-HLT, Montreal,´ Canada, June 7-8, 2012. c 2012 Association for Computational Linguistics 2. Dynamic Entity Recognition: We need to map noun phrases in text to entities in a dictionary of entities provided by knowledge bases. For example, when we encounter the noun phrase “Jeff Dean”, we need to map it to the correct entity, which can either be the Google engineer or the rock musician. How- ever, knowledge bases are incomplete in the en- tities they contain, due to newly emerging en- tities and entities in the long tail. For exam- ple, Jeff Dean the Google engineer, does not have a Wikipedia page, and thus is missing in Wikipedia-derived knowledge bases. We need to recognize and handle out-of-knowledg-base entities as they emerge. 3. Extraction under Time Constraints: Due to the need for timely extraction, our extraction methods need to produce results under time constraints. We discuss ideas for optimizing execution time. Figure 1: Noisy triples obtained from naive approach Our goal is to design a model for fact extraction that adequately addresses these three main challenges. semantics would indicate what the pattern actually Overview. Section 2 presents a naive baseline and means. For example, synonymy semantics could in- points out its shortcomings. Section 3 gives an dicate that ”return to” is the same as ”traveled to, overview of our approach. Sections 4 and 5 de- trip to, ...”, and typing semantics could reveal that it scribe our solutions for generating open sets of rela- is a pattern that applies to a person and a location. tions and entities. Section 6 describes our proposal Such lexical semantics would be useful for both a for dealing with time constraints. Finally, Section 7 user looking at the data, and also for an application concludes. using the data. TextRunner/Reverb has developed a method for 2 Naive Approach reducing noise by aggregating and cleaning the re- sulting triples, in a linguistic and statistical manner. One straightforward approach that embodies the However, they do not address the issue of seman- concepts of open sets of relations and entities, is tics, for example, there are no synonyms or type to greedily extract all pairs of noun phrases co- constraints provided. We aim for an approch which occurring within a sentence. Each such extracted provides semantics. co-occurrence would then be considered to be a rela- tional facts. However, this results meaningless facts. 3 Overview of our Approach More importantly, even for the facts that are sup- posed to be meaningful, they do not exhibit real se- Our main idea is that semantic types are crucial for mantics. Figure 1 is a screenshot of a prototype discovering an open set of relations of high qual- where we applied this approach to the ClueWeb cor- ity and for recognizing out-of-knowledge-base enti- pus. From Figure 1, we can spot meaningless pat- ties. Semantic types or classes are available in many terns which should not be extracted (see marked knowledge bases. For example, Wikipedia assigns lines). We can also spot patterns that can benefit entities to categories (e.g., Jeff Dean is in the cate- from having semantics associated with them. Such gories “living people, rock musicians, . ”), YAGO 42 ties. For further semantics of a our relations, we ar- range them into groups of synonymous patterns and 0 1 2 New New Real-time Static Entities into a hierarchy of subsumptions where general pat- Relations Entities + knowledge knowledge + types + types types base base terns subsume more specific ones. For example, the relation hpersoni met hpersoni subsumes the rela- New facts tion hpersoni married hpersoni. A full description of the relation mining algorithm is beyond the scope of this paper. Figure 2: Architectural overview of our approach 5 Open Set of Entities (Suchanek 2007) assigns entities to Wordnet classes, Having mined a large collection of semantically- Freebase (Bollacker 2008) assigns entities to its own typed patterns, we now discuss the open set of en- set of categories. tities. Patterns require that entities have types which Starting with a static knowledge base consisting satisfy the type signatures. However, if an entity is of entities and their semantic types, we can generate new, its types are not known at the time of extraction. relations in the form of phrases associated with type To prevent missing out on the facts pertaining to new signatures. For example, we could generate a rela- entities, we need to deduce types for new entities. tion hactori ’s character in hmoviei. The types indi- We propose to align new entities along the type sig- cate the kinds of entities that can stand in the rela- natures of patterns by inferring entity types from the tion expressed by the phrase. Having typed phrases type signatures. One approach would be based on helps to remove many noisy facts by automatically the following hypothesis: For a given pattern such disqualifying entities whose types do not agree with as hactori’s character in hmoviei, we can conclude phrase types. Additionally, for out-of-knowledge- that an entity pair (X, Y ), occurring with the pattern base entities we can leverage the phrases they co- in text, implies that X and Y are of the types ac- occur with to infer types for new entities. In the sim- tor and movie, respectively. However, directly infer- plest case, we can assume that for all pairs of entities ring the types from the semantically-typed patterns X and Y occurring with the phrase “’s character in”, would lead to many false positives due to the follow- X is an actor and Y is a movie. We elaborate later ing problems: on the limitations of this assumption. Figure 2 illus- trates the overall approach. • Polysemy of Syntax. The same lexico- syntactic pattern can have different type sig- 4 Open Set of Relations natures. For example, the following are three different patterns: hsingeri released halbumi, In order to generate a large comprehensive set hmusic bandi released halbumi, hcountryi re- of relations, we developed methods for automati- leased hprisoneri. For an entity pair (X, Y ) cally mining relations from text corpora. We de- occurring with this pattern, X can be one of fine a relation as a pattern which frequently oc- three different types (singer, music band, coun- curs with entities of the same type, this results in try) and Y can be one of two different types (al- semantically-typed patterns, example relations are: bum, prisoner).
Details
-
File Typepdf
-
Upload Time-
-
Content LanguagesEnglish
-
Upload UserAnonymous/Not logged-in
-
File Pages5 Page
-
File Size-