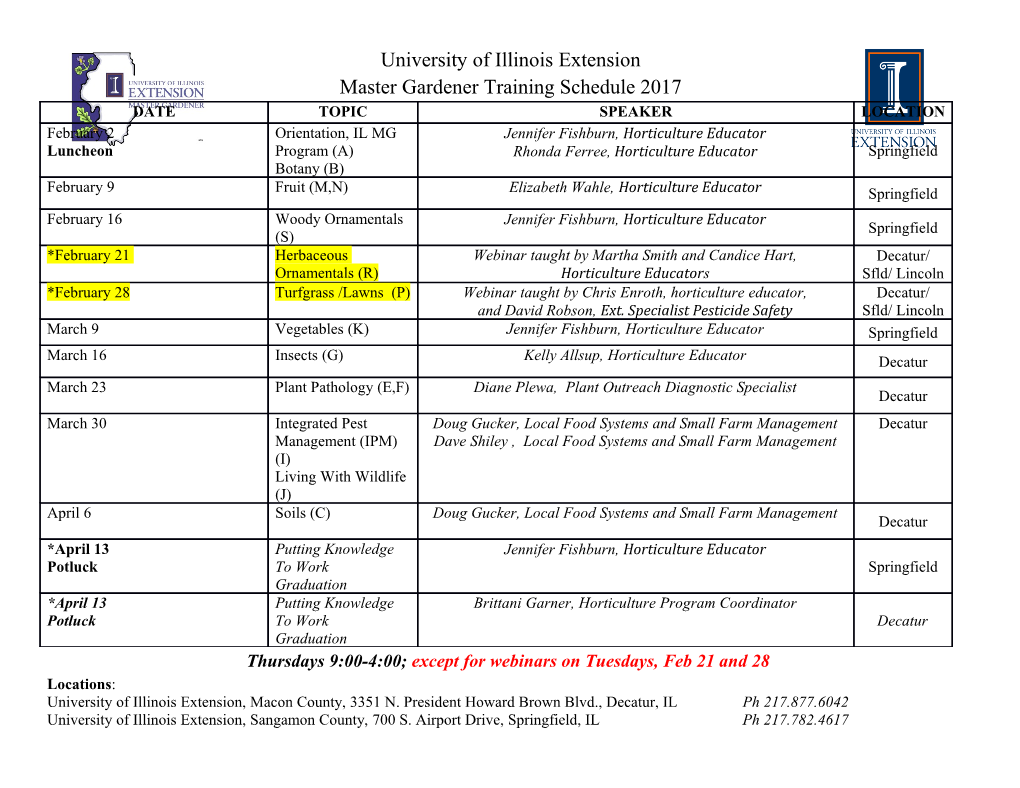
IssuesIssues && MethodsMethods forfor AnalyzingAnalyzing ZooplanktonZooplankton TimeTime SeriesSeries –– SampleSample ApplicationsApplications ofof thethe WG125WG125 ToolkitToolkit Todd O’Brien1, Todd O’Brien , 1National Marine Fisheries Service, Dave Mackas2, Silver Spring, MD 3 Mark Ohman , 2Fisheries and Oceans Canada, Institute WG125 members, of Ocean Sciences, Sidney, BC & 3 ‘data partners’ (G. Mazzocchi, 3Scripps Institution of Oceanography, La R.Harris, W. Greve) Jolla, CA TopicsTopics && practicespractices:: Value & availability of zooplankton time series Diversity of sampling designs Modes and time scales of variability Data transformation & averaging Removing seasonal cycle -> Climatology Deviations from climatology -> Anomalies Dealing with data gaps Comparing years within data sets Comparing taxa within data sets Comparing across data sets Visualization tools AdvantagesAdvantages ofof mesozooplanktonmesozooplankton forfor trackingtracking && interpretinginterpreting ‘‘OceanOcean ChangeChange’’ KeyKey intermediateintermediate stepstep inin marinemarine foodfood webswebs AbundantAbundant andand relativelyrelatively easyeasy toto samplesample LifeLife cyclecycle durationduration (>>phytoplankton,(>>phytoplankton, <<fish)<<fish) allowsallows goodgood resolution/responseresolution/response atat seasonalseasonal--toto--interannualinterannual timetime scalesscales NoNo directdirect fisheryfishery -->> reducesreduces ambiguityambiguity aboutabout causecause ofof observedobserved populationpopulation changeschanges ∗ ∗ ∗ ∗ ∗ ∗ WhereWhere areare thethe data?data? LocationsLocations withwith longlong zooplanktonzooplankton timetime seriesseries DiverseDiverse samplingsampling methodsmethods && schedulesschedules butbut mostmost shareshare thethe followingfollowing traits:traits: Densest coverage along continental margins Sampling gear fairly basic Best for mesozooplankton (~1mm-1 cm). Smaller, larger, or fragile taxa under-sampled. Sampling interval weekly-to-annual (varies with distance to home port) Longer time series often contain: - Time gaps - Changes in sampling grid or method - Changes in ‘taxonomy’ DiverseDiverse samplingsampling designs:designs: (1)(1) FrequentFrequent samplingsampling atat aa singlesingle sitesite Examples: Plymouth L4 (at right) Helgoland Roads Naples Bay Station P Hawaii Ocean Time-series (HOT) Newport NH5 Typical Advantages: Frequent & regular Year-round, good resolution of seasonal cycle Ease of visualization & analysis Typical Disadvantages: Lack within-sampling-period replication Often very nearshore DiversityDiversity ofof samplingsampling designs:designs: (2)(2) RepeatRepeat surveyssurveys ofof ‘‘standardstandard’’ lineline oror gridgrid Examples: CalCOFI (at right top) Korean coastal waters (at right bottom) Georges Bank GLOBEC Vancouver Island margin Hokkaido A-line Line P Typical Advantages: Several per year – fair-to-good resolution of seasonal cycle 43N Can compare 2D patterns (zooplankton, chl a, T, S, currents) 41 Can quantify and/or filter spatial patchiness 39 107 106 105 37 307 104 308 KOREA 103 309 102 Typical Disadvantages: 310 209 35 311 207208 JAPAN Mix of day and night samples 312 206 313 203 205 NA 204 I 314 33 H Aliasing of phenologic variability C May consume lots of dedicated ship time 31 120E 122 124 126 128 130 132 134 136 DiversityDiversity ofof samplingsampling designs:designs: (3)(3) VariableVariable locationslocations withinwithin ‘‘StatisticalStatistical AreasAreas’’ Examples: Continuous Plankton Recorder (at left) IMARPE (Peru) Earlier parts of the ODATE data set (Japan) Benguela Current Typical Advantages: Typical Disadvantages: Good resolution of seasonal Mix of day and night samples cycle Variable sampling-location Economical (multi-tasked aliasing? of spatial structure ships) Can classify/stratify samples based on water properties (ODATE) DiversityDiversity ofof samplingsampling designs:designs: (4)(4) AnnualAnnual (or(or lessless frequent)frequent) expeditionsexpeditions Examples: Hokkaido University training-ship cruises Icelandic zooplankton monitoring program Most Arctic and Southern Ocean surveys Typical Advantages: ‘Distant waters’ & extensive spatial coverage Can map changes in large scale zoogeography Typical Disadvantages: Poor or no resolution of seasonal cycle Significant confounding of variability of sampling date, seasonal cycle, and phenology AllAll fourfour designsdesigns faceface similarsimilar challenges!!challenges!! Separating multi-year change from other large ‘real’ sources of variability (seasonal, spatial, diel, ….) [filtering methods, ‘anomalies’ from region climatology] Detecting & correcting data biases [intercalibration & standardization of gear & taxonomy] Statistical consequences of temporal autocorrelation [‘effective degrees of freedom’ < n, ensemble averaging] Sensitivity & consistency of analysis & interpretation [‘common currency’, intercalibration/standardization of data processing methods] OtherOther bigbig componentscomponents (2) persistent ‘regional’ patchiness ofof zooplanktonzooplankton variability:variability: 175 (3) transient ‘small- 150 (1) seasonal 125 scale’ patchiness 100 cycle 75 50 g wet weight/m2 25 0 1-Jun-56 1-Jun-57 1-Jun-58 1-Jun-59 1-Jun-60 1-Jun-61 1-Jun-62 1-Jun-63 1-Jun-64 1-Jun-65 1-Jun-66 1-Jun-67 1-Jun-68 1-Jun-69 1-Jun-70 1-Jun-71 1-Jun-72 1-Jun-73 1-Jun-74 1-Jun-75 1-Jun-76 1-Jun-77 1-Jun-78 1-Jun-79 1-Jun-80 Date (4) Changes in vertical distribution & DVM cartoon from website of catchability Marianne Moore, Wellesley Univ. SelectiveSelective averagingaveraging andand differencingdifferencing areare thethe mainmain strategiesstrategies forfor partitioningpartitioning zooplanktonzooplankton variabilityvariability Source/scale of variability How dealt with Unresolved small-scale patchiness Minimized by averaging of plus sampling error ‘replicates’ at all levels Persistent mesoscale spatial Stratification of samples into structure spatial averaging units Annual seasonal cycle ‘Climatology’: averaging within seasons across years Interannual & decadal variability ‘Anomalies’: space-time averaged deviations from climatology ‘Global warming’ trends As for decadal TheThe artart ofof averagingaveraging zooplanktonzooplankton data:data: NonNon--normalnormal underlyingunderlying statisticalstatistical distributions!distributions! PDFs for single samples are discrete and almost always ‘contagious’ or ‘patchy’ Observed variance ≈ μ + Kμ2 (Cassie 1963) (Poisson) (~log normal) Asymmetric with strong positive skew (not as big a problem as in the past, but still a no-no for many parametric tests & comparisons) Three common alternatives for averaging (not yet a clear ‘best choice’ for all cases): Arithmetric mean Geometric mean ‘Trim means’ 1/n ΣBt/(n-1) (ПBt) (discard % from tails of (but usually derived as distribution before arithmetic mean of logB ) arithmetic or arithmetic mean of logBt) geometric averaging) Advantages: Advantages: Advantages: Advantages: Unbiased Narrow & symmetric error bars Often excludes ‘bad’ Easy to calculate error bars data Uses all data Easy to calculate Now available in Uses all data many stat packages Sampling biases additive & easy to filter (more later) Disadvantages: Disadvantages: Disadvantages: Disadvantages: Noisy Usually biased low Biased low relative to Asymmetric error arithmetic mean Discards good data bars, unless n very (a problem if n is large Input data must be >0. small) May requires an additive small) Sampling biases data offset (not Subjective, behavior multiplicative necessarily +1) hard to document WG125WG125 isis developingdeveloping averagingaveraging toolstools andand exploringexploring howhow methodmethod choicechoice affectsaffects estimatesestimates (e.g.(e.g. ofof seasonalseasonal cycle)cycle) The densely-sampled coastal time series (Naples, L4, Helgoland) are especially useful for this. If n is large: Both arithmetic and geometric means have very similar patterns (compare red & blue lines) Bias of the geometric mean is present but small compared to the overall scatter (black symbols) WhatWhat ifif thethe samplingsampling isis lessless densedense ((nn smallsmall oror timetime coveragecoverage gappygappy)?)? Climatologies calculated from randomized 50% subsample (grey lines) track the ‘100%’ patterns (blue or red lines) Peak timing more erratic than level Downward bias of geomean is proportional to within-time- period variance WhatWhat amongamong--yearyear comparisonscomparisons mightmight wewe want?want? Data courtesy W. Greve • In principle, can estimate all of the above from low-order harmonic fits to each year’s data (e.g. Dowd et al. 2004) Bt = a + ΣCj*sin (jt+Φj) • In practice, data gaps & lack of within-time-period replication limit usefulness of this approach AlternativeAlternative forfor amongamong yearyear comparison:comparison: DeriveDerive anomaliesanomalies AAt (differences(differences ofof datadata BBt fromfrom climatologyclimatology BB )) forfor eacheach observationobservation periodperiod Anomalies (mostly + here) can be averaged to give an annual anomaly Ay Three commonly used equations: At = log (Bt/B) At = (Bt-B)/B At = Bt - B The first two are unit-free ratios measuring % change (more later on why this is useful). The last has the same units as the raw data. With sufficient data, all three At estimates yield similar multi-year time series. The log scale index is usually less noisy Data courtesy G. Mazzocchi Beware of using overall annual mean
Details
-
File Typepdf
-
Upload Time-
-
Content LanguagesEnglish
-
Upload UserAnonymous/Not logged-in
-
File Pages34 Page
-
File Size-