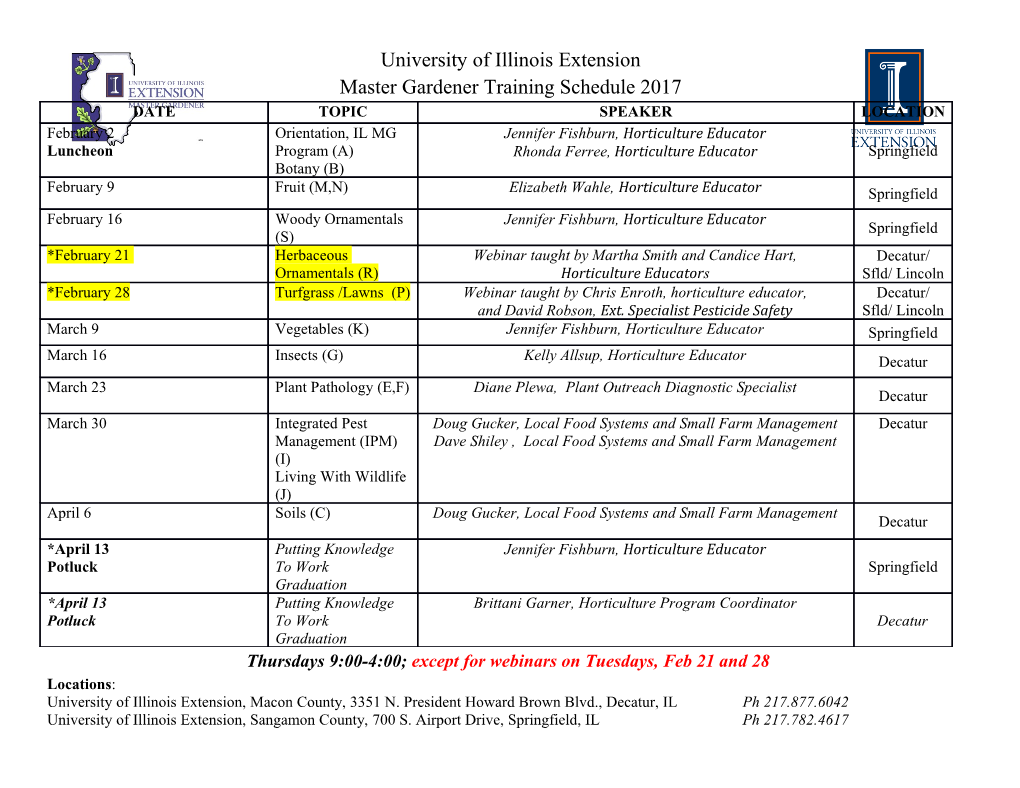
Capacity Factor of Wind Power Realized Values vs. Estimates Nicolas Boccard¤ October 2008 Abstract For two decades, the capacity factor of wind power measuring the mean energy delivered by wind turbines has been assumed at 35% of the name plate capacity. Yet, the mean realized value for Europe over the last five years is closer to 21% thus making levelized cost 66% higher than pre- viously thought. We document this discrepancy and offer rationalizations, emphasizing the long term variations of wind speeds. We conclude with the consequences of the capacity factor miscal- culation and some policy recommendations. Keywords: Electricity, Renewables, Planning JEL codes : L51, H42, D61 1 Capacity Factors Puzzle Wind Powered Generation (WPG) is the top player in the renewables field and can only benefit from more information regarding some of its characteristics. Very little has been published regarding capac- ity factors at the global level of an entire country. Contrary to the implicit popular opinion, the wedge with local values happens to be large because particular findings cannot be generalized. Our aim in this section is to contrast capacity factor estimates from the academic literature with the actual level computed from recorded installation and production in Europe. 1.1 Theory and Local observations The capacity factor (CF) of wind power is the ratio of average delivered power to theoretical maximum power. It can be computed for a single turbine, a wind farm consisting of dozens of turbines or an entire country consisting of hundreds of farms. Although geographical location determines in great part the capacity factor of a wind farm, it is also a matter of turbine design. Indeed, a large rotor combined with a small generator will take advantage of just about any wind and achieve an artificially high CF, obviously at the cost of a low yearly energy output. Most of the literature on capacity factors is in fact composed of studies trying to identify optimal turbine designs for particular places; the reported CF is then the one corresponding to maximum yearly energy output. ¤Departament d’Economia, Universitat de Girona, 17071 Girona, Spain.Financial support from Instituto de Estudios Fis- cales, Generalitat de Catalunya (contract 2005SGR213 and Xarxa de referència d’R+D+I en Economia i Polítiques Públiques) and Ministerio de Educación y Ciencia (project SEJ2007-60671) are gratefully acknowledged. 1 Electronic copy available at: http://ssrn.com/abstract=1285435 Table1 displays a number of capacity factor estimates, mostly obtained with computational mod- els using wind speed data (and sometimes of wind power output at specific farms).1 Although some low measures are recorded, the general picture is a rather high capacity factor; for instance table entries average at 37%. It is interesting to note that studies geared at computing global wind power resources are based on more realistic values: Grubb and Meyer(1993), World Energy Council(1994) and Hoog- wijk et al.(2004) use respectively 22.5%, 25,1% and 26,5%. 2 Nfaoui et al.(1991): 33%, L, MO van Wijk et al.(1992): 22%, L, NL ² ² Wood(1994): 55%, L, NZ Cavallo(1995): 60%, L, Ka, US ² ² Salameh and Safari(1995): 35%, L, JO Cataldo and Nunes(1996): 40%, L, UY ² ² Abed(1997): 40%, T Iniyan et al.(1998): 19%, L, IN ² ² Iniyan and Jagadeesan(1998): 25%, L, IN Jangamshetti and Rau(1999): 29%, L, IN ² ² Pryor and Barthelmie(2001): 25–51%, L, DK Lu et al.(2002): 39%, L, HK ² ² Chang et al.(2003): 45%, L, TW Teetz et al.(2003): 49%, L, AQ ² ² Doherty et al.(2004): 31%, L, MD, US Jaramillo et al.(2004): 51%, L, MX ² ² Rehman(2004): 38%, L, SA Abderrazzaq(2004): 24%, L, DE ² ² Bird et al.(2005): 38%, L, CA, US Denholm et al.(2005): 46%, L, ND, US ² ² Ilkan et al.(2005): 35%, L, CY White(2006): 20–29%, L, MN, US ² ² Ahmed Shata and Hanitsch(2006): 53%, L, EG Inoue et al.(2006): 17–45%, L, JP ² ² Caralis et al.(2008): 27–30%, G, GR Sahin(2008): 30%, G, TR ² ² Letter codes are G for global, L for local, T for theory and ISO 3166 country codes Table 1: Capacity Factors Estimates 1.2 Global realizations Table2 contrasts the theory with actual records of WPG in European countries ordered by currently installed capacity. Our main reference is the wind energy barometer of think-tank EurObserv’ER with some corrections from more reliable sources whenever available.3 We also use the mid-year average installed capacity to mitigate for the continuous development of WPG; it thus yield greater capacity factors than the mere ratio of output to capacity for a given year. Although the capacity (GW) and output (TWh) figures reported here have been published every year over the last decade by national public agencies, they have seldom been used to produce a capacity factor ratio, let alone a country comparison. The average European CF over the last five years is less than 21%, significantly lower than the 24% claim made by the European wind power lobby for a “normal wind year” at the current level of de- velopment (cf. EWEA(2008) p29). Realized capacity factors oscillate across time and across regions 1We searched the Elsevier, Wiley and Springer databases for “capacity factor” AND “wind power”. 2We convert their full-load hours assumption into CFs. 3While there are only minor revisions regarding installed capacity from year to year in all sources, generation data show important discrepancies, both between year to year reports of the same source and between different sources. We have favored the most recent reports and those of Transmission System Operators over European think tanks. For the UK, we use BERR(2008); for Spain, reports from the TSO REE; for France, the report by France Energie Eolienne. 2 Electronic copy available at: http://ssrn.com/abstract=1285435 Area EU15 DE ES DK IT UK FR PT Capacity (GW) 56.3 22.2 14.1 3.1 2.7 2.5 2.4 2.2 Energy (TWh) 97.7 33.7 28.8 6.1 4.2 5.3 4.2 3.8 Capacity Factor (%) 20.8 17.5 24.8 22.8 19.1 26.1 22.3 22.7 Area NL AT GR IR SE BE PO CA US Capacity (GW) 1.7 1.0 0.9 0.8 0.7 0.3 0.3 2.4 16.6 Energy (TWh) 3.5 2.0 1.9 1.9 1.2 0.5 0.5 4.4 32.1 Capacity Factor (%) 21.5 20.1 29.3 29.3 21.7 20.0 25.9 22.3 25.5 Table 2: Average Capacity Factors over 2003-07 in the 20–30% range.4 The higher end is found in Greece, Ireland and UK which benefit from numer- ous windy costal areas with low density of population that enable effective sitting in those preferable zones. For reference, Table2 includes the US 5 and California (CA), the state with the longest experi- ence in WPG; there is a stark contrasts with AWEA(2005)’s claim that a 35% capacity factor is typical for the US. The strongest discrepancy between theory and reality regards the large scale development of WPG with offshore participation. Wild claims are made regarding future possibilities at the 2020-2030 hori- zons that up to now, in our opinion, are all but warranted by facts. Academic reports regarding the UK by SDC(2005), Gross et al.(2006) or Sinden(2007) borrow at face value the 35% capacity factor at the 2020 horizon adopted by Dale et al.(2004). The later authors justify their choice on the grounds that the UK wind resource is excellent and that half the capacity will be offshore. The first statement is true but only in relative terms. Indeed, Table2 indicates that UK WPG outperforms the EU mean by 25% but since the later is appallingly low, the feat is not so impressive. Secondly, given the 27.5% long-term CF average for current on-shore capacity in the UK, future off-shore wind power would need to reach a 43% CF which is quite off the mark at the moment since the 2003-07 average is a meager 26.5%, as one can check from table 7.4 in BERR(2008). The more recent opinion by BWEA(2006) (cf. Table 1 p16) shows more restraint in anticipating capacity factors of 30% for onshore and 35% for offshore. At the European level, EWEA(2008) assumes that by 2020 capacity factors will reach 29.6% for onshore and 44.6% for offshore.6 For onshore, this means that repowering, better sitting and improved design of wind turbines would succeed to increase overall efficiency by 29.6 1 42%. Summing-up, 20.8 ¡ ' capacity factors above 30% for onshore and above 40% for offshore appears to be mere leaps of faith lacking the support of either hard data or a proper model of the learning curve able to deliver the promises enhancements w.r.t. currently observed levels. 1.3 Correction for Wind Indexes The long term distribution of wind speeds is known to depend on meteorological phenomena whose duration is of the order of the decade. Capacity factors based on yearly output are thus likely to evolve and do not reflect the long term potential of a region. For that reason, a low observed capacity factor may be due to unusually low winds, below their long term potential. Several research institutes from 4We only report on areas where wind power is not anecdotal (i.e., makes more than one percent of system capacity). 5 The Energy Information Administration (EIA) uses sampled (40%) and estimated (60%) data.
Details
-
File Typepdf
-
Upload Time-
-
Content LanguagesEnglish
-
Upload UserAnonymous/Not logged-in
-
File Pages10 Page
-
File Size-