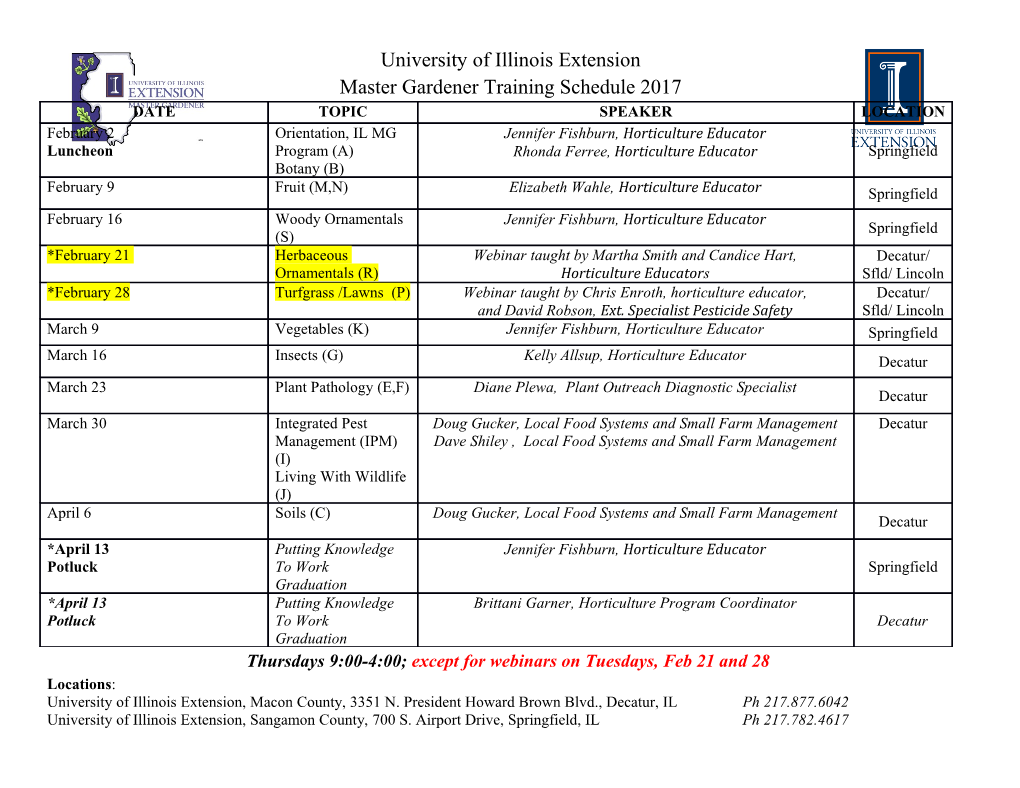
Supporting Information Maynard et al. 10.1073/pnas.1712211114 SI Materials and Methods outcomes, and see Dataset S2 for the community-level values of Calculating Intransitivity. There are numerous approaches for average competitive ability and variance in competitive ability. calculating competitive intransitivity; however, most assume a Functional and Phylogenetic Dissimilarity. Functional dissimilar- binary win/loss outcome, such that they cannot easily allow for ity and phylogenetic dissimilarity were calculated for each com- deadlocks among species. Thus, we use an approach proposed munity and included as potential confounding variables, given by ref. 47 to calculate “transitivity in probability,” where the mea- that these community characteristics can independently affect sure of intransitivity refers to the stochastic probability that one functional outcomes (51, 52). Functional dissimilarity was calcu- species displaces another. The competitive outcomes (win, loss, lated using Rao’s quadratic entropy (53) in the FD package in R, tie) for all pairings were converted into a pairwise competition using 11 functional traits: wood decomposition rate (% mass loss matrix, with a value of “1” representing a win and a value of “0” over 12 wk), enzyme activity of five hydrolytic and three oxidative representing a loss. Pairings that resulted in a draw were assigned enzymes (given in units of fluorescence and absorbance, respec- a value of 0.5, representing only stochastic fluctuations in out- tively), hyphal density (micrograms of dry mass per square cen- comes when interacting (see Dataset S1). Following ref. 47, we timeter), and growth rate (millimeter extension per day). See calculated a measure of intransitivity for each of the 150 commu- Dataset S1 for the complete data, and see ref. 15 for detailed nities used in the experiment by first permutating the rows and methods of trait measurements. Functional dissimilarity was columns of their competition matrix to find the ordering with the included as a predictor in the statistical models (Table S2), and maximum number of matrix entries > 0.5 in the upper right trian- is included in Dataset 2. gle (an approach first proposed by ref. 50). Note that this can be Phylogenetic dissimilarity was calculated using mean pair- computationally intensive, requiring nearly 500 million permuta- wise phylogenetic distance (54) using the concatenated ITS and tions to be checked for a 12-species community. After sorting, LSU sequences for each fungus (Fig. S1). Phylogenetic anal- we calculated a normalized measure of intransitivity, taken as ysis for these isolates is outlined in detail in ref. 15. Briefly, the relative proportion of entries in the upper right triangle that DNA sequences were aligned using Multiple Alignment using were less than their corresponding entry in the lower left triangle. Fast Fourier Transform (MAFFT) version 6 (55) using the Q- That is, INS-I algorithm for the alignment of ITS and the G-INS-I algo- rithm for LSU sequences. The combined LSU+ITS dataset P I (a < a ) Intransitivity = ij ji ; was constructed by concatenating both alignment files, and n(n − 1)=2 the evolutionary history was inferred using Maximum Like- lihood methods by calculating the bootstrap consensus tree where the summation is taken over all i < j , and the operator I (·) from 500 replicates. Phylogenetic pairwise distances were cal- is the indicator function, which equals 1 if aij < aji and 0 other- culated for all 18 isolates using Molecular Evolutionary Genet- wise. This value ranges between 0 (completely hierarchical) and ics Analysis (MEGA) version 5 (56) using the number of dif- 1 (completely intransitive). See Dataset S2 for the community- ferences for all nucleotide positions with greater than 95% site level intransitivity measurements. coverage. Phylogenetic dissimilarity (mean pairwise distance) was calculated for each community and included as a predic- Competitive Rankings and Average Competitive Ability. Some tor in the statistical models (Table S2), and is included in isolates were strongly defensive, neither winning nor losing Dataset 2. against strongly combative fungi; others were strongly offen- sive, rarely deadlocking but having high rates of wins and losses SI Discussion (Dataset S1). To capture this variation in combative strategies, The goal of the simulation experiment is twofold: first, to explore we used the Elo Ranking system (48) in the PlayerRatings pack- how inherent correlations between richness and the competitive age in R. As opposed to standard rankings, Elo penalizes a network constrain the realized patterns we observe across the highly ranked competitor if it loses against a weak competitor, metacommunity, and, second, to explore the relative importance and assigns a higher rank to a weak competitor if it draws or of the competitive network structure and richness in relation to wins against stronger competitors. Whereas a simple hierarchy additional confounding factors (e.g., phylogenetic or functional based on win percentage would assign a low rank to a species diversity, or species-specific effects). Out of 20,000 communities, with a high number of draws but no wins, the Elo system ranks ∼4% were classified as highly intransitive, strong competitors these species closer to the middle of the hierarchy. This approach (Fig. 4A), 3% were classified as hierarchical, weak competitors better captured variation among “defensive” competitors and (Fig. 4B), 15% were classified as hierarchical, strong compet- “weak” competitors in our study system (15). itors (Fig. 4C), and 21% were classified as intransitive, weak com- Given that the Elo rating system was originally designed for petitors (Fig. 4D). The remaining 55% of communities (Fig. S4B) ranking chess players, it does not return readily interpretable fell within the 33rd and 66th percentile for either intransitivity or values (the raw rankings ranged between 2,000 and 2,500 for average competitive ability, such that they were not classified into this system). Thus, these values were subsequently standardized any of the four cases. between 0 and 1 for use here. Note that the full ranking was If we completely ignore the role of the competitive network, obtained using a more diverse community comprising 37 isolates we see essentially no relationship between diversity and func- (15), and so the lowest rated species used here has a value of 0.05 tion (Fig. S4A), mirroring the experimental outcomes (i.e., Fig. (Laetiporus huroniensis) rather than exactly 0. 1). However, when we break down the communities into those For each experimental community, an estimate of average that have high vs. low intransitivity and high vs. low competi- competitive ability and variance in competitive ability was cal- tive ability, we see clear patterns emerge (Fig. 4). Moreover, the culated by taking the simple average and the empirical variance two dominant groups that emerge—hierarchical, high competi- of these ranks across all species in the community. See Dataset tive ability and intransitive, low competitive ability—have essen- S1 for the individual rankings and all of the pairwise competitive tially offsetting trends. Thus, despite the fact that the competitive Maynard et al. www.pnas.org/cgi/content/short/1712211114 1 of 5 network plays an important role in determining community-level network that constrain the realized set of network structures function, these contrasting relationships effectively offset each (e.g., Fig. S2). Thus, our results suggest that there should be three other, such that, when taken on aggregate, there appears to be clear groups (and three different corresponding BEF relation- no relationship. ships) that we predominantly observe in natural settings: hierar- Once richness is greater than approximately eight species, chical communities with high competitive ability (negative BEF), it becomes exceedingly rare that a community is hierarchical intransitive communities with low competitive ability (positive with low competitive ability, or that it is intransitive with high BEF), and communities with moderate intransitivity and mod- competitive ability, reflecting inherent correlations within the erate competitive ability (null BEF). Schizophyllum commune Porodisculus pendulus Phlebia rufa Merulius tremellosus Phlebiopsis flavidoalba Hyphoderma setigerum Laetiporus caribensis Laetiporus gilbertsonii Laetiporus conifericola Laetiporus huroniensis Tyromyces chioneus Pycnoporus sanguineus Fomes fomentarius Lentinus crinitus Phellinus gilvus Phellinus hartigii Phellinus robiniae Xylobolus subpileatus Fig. S1. The phylogenetic tree for the 18 fungi. DNA sequences were aligned using MAFFT version 6 (55) using the Q-INS-I algorithm for the alignment of ITS and the G-INS-I algorithm for LSU sequences. The combined LSU+ITS dataset was constructed by concatenating both alignment files, and the evolutionary history was inferred by using Maximum Likelihood methods by calculating the bootstrap consensus tree from 500 replicates. The tree is drawn to scale, with branch lengths measured in the number of substitutions per site. Phylogenetic pairwise distances were calculated for all 18 isolates using MEGA version 5 (56) using the number of differences for all nucleotide positions with greater than 95% site coverage. Phylogenetic dissimilarity (mean pairwise distance) was calculated for each community and included as a predictor in the statistical models (Table S2). Maynard et al. www.pnas.org/cgi/content/short/1712211114 2 of 5 Fig. S2. The community-level
Details
-
File Typepdf
-
Upload Time-
-
Content LanguagesEnglish
-
Upload UserAnonymous/Not logged-in
-
File Pages5 Page
-
File Size-