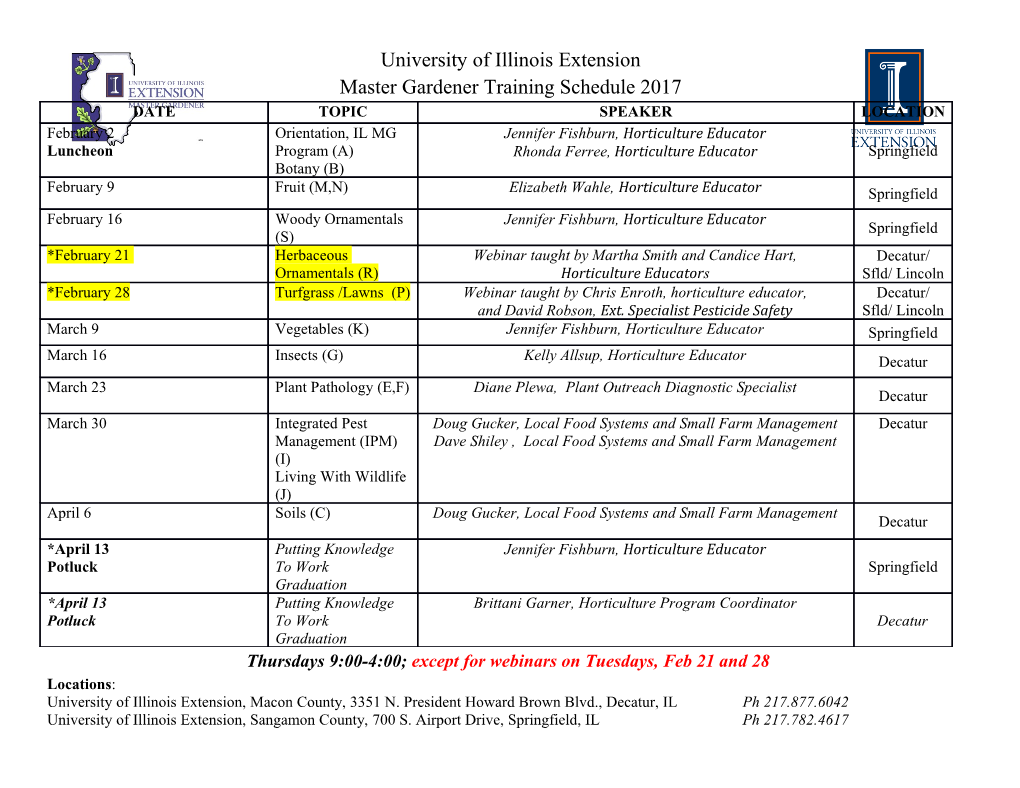
Running head: CAUSALITY IN IMPLICIT COGNITION The role of causal structure in implicit cognition Benedek Kurdi Adam Morris and Fiery A. Cushman Yale University, New Haven, Connecticut Harvard University, Cambridge, Massachusetts Author Note Benedek Kurdi, Department of Psychology, Yale University; Adam Morris and Fiery A. Cushman, Department of Psychology, Harvard University. Parts of this research have been presented at the 41st Annual Meeting of the Cognitive Science Society, in Montreal, Canada, in July 2019, and at the Annual Meeting of the Person Memory Interest Group, Severn Bridge, Canada, in October 2019. All raw data files, analysis scripts, and materials used in this article are available for download from the Open Science Framework (OSF; https://osf.io/afwyk/?view_only=7be20ac60b9c4c688de7a27cdbad0ce1). Studies 1B, 5, and 6 were formally preregistered. We thank Elad Zlotnick and Victor Yang for assistance with study coding. Declarations of interest: Benedek Kurdi is a member of the Scientific Advisory Commit- tee of Project Implicit, a 501(c)(3) non-profit organization and international collaborative of re- searchers who are interested in implicit social cognition. Correspondence concerning this article should be addressed to Benedek Kurdi, Depart- ment of Psychology, Yale University, New Haven, CT 06511, email: [email protected]. Running head: CAUSALITY IN IMPLICIT COGNITION 2 Abstract Causal relationships, unlike mere co-occurrence, allow humans to obtain rewards and avoid pun- ishments by intervening on their environment. Accordingly, explicit (controlled) cognition is known to represent causal relationships above and beyond mere co-occurrence. In this project, we conduct stringent tests of whether implicit (automatic) cognition is also sensitive to causal re- lationships and begin to probe the representational mechanisms underlying such sensitivity. Par- ticipants (N = 4836) observed causal events during which two stimuli were equally contingent with positive or negative outcomes but only one of them was causally responsible for these out- comes. Across 6 studies, varying in design and amount of verbal scaffolding provided, differ- ences in causal status consistently guided not only explicit measures of evaluation (Likert and 46 slider scales; Cohen’s d = .28, BF10 > 10 ) but also their implicit counterparts (Implicit Associa- 29 tion Tests; Cohen’s d = .22, BF10 > 10 ). However, unlike explicit evaluations, implicit evalua- tions were not sensitive to causal relationships that had to be derived by combining disparate past experiences via causal principles. Taken together, these studies suggest that implicit evaluations encode causal information, but through compressed value representations influenced by causal knowledge rather than online computations over a causal model. Keywords: associative learning; causal learning; dual-process theories; implicit cognition; im- plicit evaluation Running head: CAUSALITY IN IMPLICIT COGNITION 3 The role of causal structure in implicit cognition 1. Introduction Parents of newborns tend to keep diapers around, and they also tend to be less happy than their peers (Blanchflower, 2009). So, if you and your partner are hoping for a successful concep- tion, here are some tips: Buy some diapers, and try not to have too much fun. We all know better, and for a good reason: Humans represent not just statistical relation- ships between variables in the world, but also the causal relations among them (Buckner, An- drews-Hanna, & Schacter, 2008; Doll, Duncan, Simon, Shohamy, & Daw, 2015; Doll, Simon, & Daw, 2012; Lagnado, Waldmann, Hagmayer, & Sloman, 2007; as do nonhuman animals, Tol- man, 1949). The logic of cause and effect enables us to explain the past, predict the future, plan our actions, and assign responsibility and blame. It tells us that having babies causes parents to buy diapers, but buying diapers doesn’t cause parents to have babies. In the present project we set out examine whether automatic cognition in on the joke. More precisely, we ask: Is automatic cognition (Kahneman, 2011; Sloman, 1996), that is, thoughts and behaviors accessed by implicit measures (e.g., Fazio, Sanbonmatsu, Powell, & Kardes, 1986; Greenwald & Banaji, 1995; Meyer & Schvaneveldt, 1971) and emerging under cognitive load or time pressure (e.g., Phillips & Cushman, 2017; Rand, 2016), sensitive to peo- ple’s representations of causal structure? Current theories in psychology (De Houwer, 2014; 2018; Kurdi & Dunham, 2020; Lieberman, Gaunt, Gilbert, & Trope, 2002; Sloman, 1996; 2014; Smith & DeCoster, 2000; Strack & Deutsch, 2004) and philosophy (Gendler, 2008; Madva, 2016; Mandelbaum, 2016) diverge on this fundamental question. Moreover, existing empirical evidence is mostly indirect and ambiguous (De Houwer & Vandorpe, 2010; Hughes, Ye, Van Dessel, & De Houwer, 2018; Moran & Bar-Anan, 2013; Moran, Bar-Anan, & Nosek, 2015). Running head: CAUSALITY IN IMPLICIT COGNITION 4 Despite mixed existing evidence and opposing theoretical positions, the first five experi- ments reported below showed that participants’ causal representations can influence their auto- matic judgments. Thus, in the final study we asked: How? Some current theories propose that au- tomatic processes perform computations organized around causal principles and do so over ex- plicit representations of causal relations (De Houwer, 2018; Mandelbaum, 2016; Sloman, 2014). An alternative theory is that such computations are not themselves a part of automatic cognition, but instead generate precompiled representations that can be quickly retrieved during automatic cognition (Kurdi & Dunham, 2020). In support of the second possibility, we find that automatic cognition does not spontaneously integrate two elements of causal knowledge (A ® B, B ® C) presented at separate points time to derive a novel causal relation (A ® C). Controlled cognition, however, does. 1.1. Against the Role of Causal Structure in Automatic Cognition Human cognition involves many different kinds of process. Some are relatively fast, au- tomatic, and unconscious, whereas others are relatively slow, effortful, and conscious (Green- wald & Banaji, 1995; Lieberman et al., 2002; Sloman, 1996; Smith & DeCoster, 2000; Strack & Deutsch, 2004). The first type of process is routinely indexed via implicit measures involving time pressure, cognitive load, or misattribution, whereas the second type of process is routinely indexed via self-report. There is considerable interest in the organizational principles underlying this division. A recurrent theme, spanning decades of theorizing, is that automatic cognition in- volves merely associative representations, while controlled cognition has the ability to draw on causal representations. In his seminal review, Sloman (1996) proposes that “[r]ather than trying to reason on the basis of an underlying causal or mechanical structure, [implicit cognition] constructs estimates Running head: CAUSALITY IN IMPLICIT COGNITION 5 based on underlying statistical structure” (p. 4). Similarly, according to Lieberman et al. (2002), when implicit cognition is confronted with a causal inference problem, it “[…] simply estimates the similarity or associative strength between the antecedent and the consequent” (p. 210) with- out taking into account the direction of the causal relationship or the presence of confounding variables (see also Smith & DeCoster, 2000; Strack & Deutsch, 2004). Complementary argu- ments have also been made in philosophy, perhaps most prominently by Gendler (2008) who posits a distinction between purely associative and arational aliefs (implicit cognition) and prop- ositional and rational beliefs (explicit cognition). Similarly, Madva (2016) contends that implicit cognition is uniquely sensitive to “spatiotemporal relations in thought and perception” (p. 2659) but does not reflect how stimuli are related to each other, including their participation in causal relationships. The claim that automatic cognition encodes mere associations, but not causal relation- ships, has some direct experimental support (Moran et al., 2015; Moran & Bar-Anan, 2013). In these studies, one novel creature was repeatedly shown to cause a pleasant auditory stimulus to start, while a second creature was repeatedly shown to cause that stimulus to stop; a third and a fourth creature started or stopped unpleasant stimuli. If a cognitive process is sensitive only to statistical information and not to causal relationships, both creatures co-occurring with the pleas- ant stimulus should be evaluated positively and both creatures co-occurring with the unpleasant stimulus should be evaluated negatively. By contrast, if a cognitive process also encodes causal- ity, then the creatures ending, rather than starting, stimuli should be evaluated opposite of the va- lence of the stimulus with which they had been paired. Moran and Bar-Anan (2013) obtained the former (associative) pattern of results on im- plicit (indirect) measures, including the Implicit Association Test (IAT; Greenwald, McGhee, & Running head: CAUSALITY IN IMPLICIT COGNITION 6 Schwartz, 1998) and the Sorting Paired Features Task (SPF; Bar-Anan, Nosek, & Vianello, 2009) and the latter (causal) pattern of results on explicit (self-report) measures. However, in a subsequent experiment conducted by the same authors, implicit evaluations did show sensitivity to causal relations; this occurred when participants were instructed to form impressions of the four creatures rather than to memorize statistical
Details
-
File Typepdf
-
Upload Time-
-
Content LanguagesEnglish
-
Upload UserAnonymous/Not logged-in
-
File Pages63 Page
-
File Size-