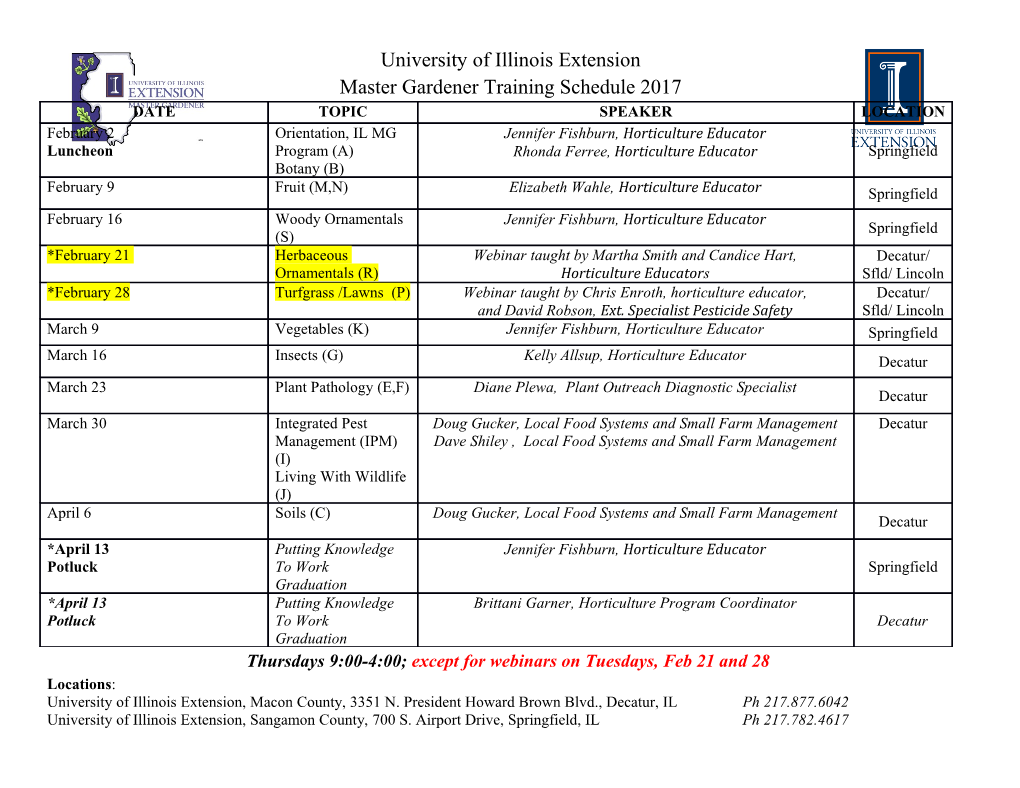
Investigating the Performance of an Adiabatic Quantum Optimization Processor Kamran Karimi, Neil G. Dickson, Firas Hamze, M.H.S. Amin, Marshall Drew-Brook, Fabian A. Chudak, Paul I. Bunyk, William G. Macready, and Geordie Rose D-Wave Systems Inc., 100-4401 Still Creek Drive, Burnaby, B.C., Canada, V5C 6G9∗ Adiabatic quantum optimization offers a new method for solving hard optimization prob- lems. In this paper we calculate median adiabatic times (in seconds) determined by the minimum gap during the adiabatic quantum optimization for an NP-hard Ising spin glass instance class with up to 128 binary variables. Using parameters obtained from a realis- tic superconducting adiabatic quantum processor, we extract the minimum gap and matrix elements using high performance Quantum Monte Carlo simulations on a large-scale Internet- based computing platform. We compare the median adiabatic times with the median running times of two classical solvers and find that, for the considered problem sizes, the adiabatic times for the simulated processor architecture are about 4 and 6 orders of magnitude shorter than the two classical solvers' times. This shows that if the adiabatic time scale were to determine the computation time, adiabatic quantum optimization would be significantly su- perior to those classical solvers for median spin glass problems of at least up to 128 qubits. We also discuss important additional constraints that affect the performance of a realistic system. I. INTRODUCTION NP-hard optimization problems arise frequently in scientific and commercial applications [1, 2]. Their formal intractability, combined with the significant value that can be generated by finding arXiv:1006.4147v4 [quant-ph] 27 Jan 2011 better techniques for solving them, has led to a thriving research community searching for more effective and useful algorithms. While much progress has been made, for many applications the best current algorithms are not nearly good enough. An example of a very difficult optimization problem is the Eternity II puzzle [3]. Adiabatic Quantum Optimization (AQO) algorithms [4{8] may be effective tools to tackle hard optimization problems, making it important to study their performance potential. AQO algorithms are physics-inspired approaches that can be used as components of both exhaustive and heuristic ∗Electronic address: kkarimi, ndickson, fhamze, mhsamin, marshall, fchudak, pbunyk, wgm, [email protected] 2 solvers. In these algorithms, a Hamiltonian changes over time in such a way that after the compu- tation is complete, the system has a non-zero probability of being in its lowest energy state, also known as its ground state. The ground state encodes the global optimum of the problem being solved. The excited states correspond to solutions that are not globally optimal. The M th excited state corresponds to the (M + 1)th best solution. AQO algorithms are not well understood. In particular it is very difficult to predict how their running time scales with problem size for any particular instance class of interest. Most of the work that has been done on this question focuses on the issue of whether, for asymptotically large problems, these algorithms require running times that scale polynomially or exponentially with problem size for some specific choice of solver parameters and instance class. Here we are not going to directly address these difficult issues, and approach the analysis of an AQO algorithm from a much simpler perspective. Previous studies of the performance of adiabatic algorithms [5, 9, 10] were merely concerned with the scaling and not the actual value of the time in e.g., seconds for a realistic system. Moreover, in all those studies it is assumed, for example, that the temperature of a real system can always be kept much lower than the minimum energy gap between the ground state and first excited state, and that the adiabatic time is the only relevant timescale. Here, we present results suggesting that under the same assumptions, a superconducting hardware implementation [11] of an AQO algorithm could offer a speedup of several orders of magnitude over state of the art conventional approaches. However, we show that for a real processor with reasonable energy scales, the minimum energy gap is much smaller than feasible temperatures, even for fairly small problems, and thus, the simulations cannot accurately predict runtime scaling of such a processor. We also find that the adiabatic time is currently negligible compared to other necessary processes, such as readout of the results and thermalization (allowing the processor to cool down after a readout has been performed). The rest of this paper is organized as follows. Section 2 presents relevant details of the simu- lated processor. Section 3 introduces the problems we solve and provides information about the techniques needed to perform our large-scale simulations. Section 4 presents the classical solvers used in this study and compares their performance with that of our simulated AQO processor. Section 5 concludes the paper. 3 II. THE SIMULATED PROCESSOR In order to calculate adiabatic times for a quantum computation, in units of seconds, it is necessary to target a specific AQO processor architecture. In more theory-oriented publications, no limitation is placed on the connectivity of the architecture, but arbitrary connectivity becomes impractical to fabricate as the number of qubits increases. Here, we model a processor architecture with finite connectivity that has been fabricated. All of the simulation parameters come from measurements done on the processor in [11], so there were no free parameters. This processor is a programmable superconducting integrated circuit [12] designed to perform AQO with up to 128 pairwise-coupled [13] superconducting flux qubits [11]. To our knowledge, there are no other AQO processor architectures of comparable size under development. For analyzing an AQO process of a closed system at zero temperature, the key details are captured in the hardware's Hamiltonian. As with the aforementioned studies, in order to perform the analysis, we make the assumption that the temperature of the processor is much smaller than the minimum energy gap, effectively zero, and that there is no disturbance from any outside environment. This approximation is clearly not exact, but is necessary, because of the infeasibility of simulating a non-trivial open system. The Hamiltonian we consider here is of the form H(s) = A(s) HI + B(s) HP (1) HI and HP are dimensionless, and s = t=tf is a normalized time 0 ≤ s ≤ 1. The initial dimension- less Hamiltonian as implemented by the hardware [11] is N X x HI = − σi (2) i=1 x where σi is the Pauli X matrix for qubit i. The final Hamiltonian, HP , encodes the problem to be solved, and must be diagonal in the basis in which the qubits are read out. Because of this, the final state of the system will be classical, and therefore, qubits can be read out individually without destroying the state. The specific form we use is N X z X z z HP = hiσi + Jijσi σj (3) i=1 i;j2E z where σi is the Pauli Z matrix for qubit i. The real-valued vector h and matrix J together define a problem instance, and are normalized such that −1 ≤ fhi;Jijg ≤ +1. E is the allowed edge set, dictating which Jij can be non-zero (all other Jij are zero). In other words, E is the set of pairwise 4 FIG. 1: (Colour online) Top: Two representations of the 8 vertex/variable/qubit unit cell graph (the complete bipartite graph on 4 vertices K4;4). On the left, the variables are shown as black lines, with the allowed edge set E denoted by the set of blue points (intra-cell couplers) where the lines intersect. Note that this is not a conventional graph representation. On the right, showing the conventional graph representation, the variables are the black dots, and the non-zero edges are shown as the blue connecting lines. Bottom: 4 tiled K4;4 unit cells, giving a total of 32 variables. Here the red circles represent edges between unit cells (inter-cell couplers). Larger edge sets are constructed by further tiling. connections between qubits in our particular processor [11]. We restrict E to correspond to tiled bipartite K4;4 graphs, as shown in Fig. 1. The functions A(s) and B(s) in Eq. (1) have units of energy. Here we use the functional forms shown in Fig. 2. These functions are determined from the devices in the real processor we have simulated [11, 13{15], i.e. the processor uses a similar annealing schedule. The energy scales of A(s) and B(s) are limited by practical considerations for the actual superconducting devices used in the modeled processor, such as junction sizes, critical currents, etc. At the beginning of the 5 FIG. 2: (Colour online) Envelope functions A(s) and B(s) of the simulated processor. computation (the leftmost side of Fig. 2 at s = 0), the transverse term (2) in the Hamiltonian (1) is dominant. As the computation proceeds, the strength of the diagonal term (3) grows while the transverse term shrinks. Once the transverse term has been turned off completely (the rightmost side of Fig. 2 at s = 1) the qubits are read out in the Z basis. Note that while these are not the envelope functions A(s) ∼ (1 − s) and B(s) ∼ s used in other studies of AQO [5, 9], they do satisfy the underlying requirement of the AQO algorithms in that A(0) B(0) and A(1) B(1). We do not expect the results to change significantly if the linear envelope functions were used. Changing s from 0 to 1 within time tf at zero temperature results in finding the global optimum with measurable probability as long as tf & ta, where [16, 17] dH 4 < 1j ds j0 > s=s∗ ta = 2 ; (4) πgm which here we refer to as the adiabatic time scale.
Details
-
File Typepdf
-
Upload Time-
-
Content LanguagesEnglish
-
Upload UserAnonymous/Not logged-in
-
File Pages14 Page
-
File Size-