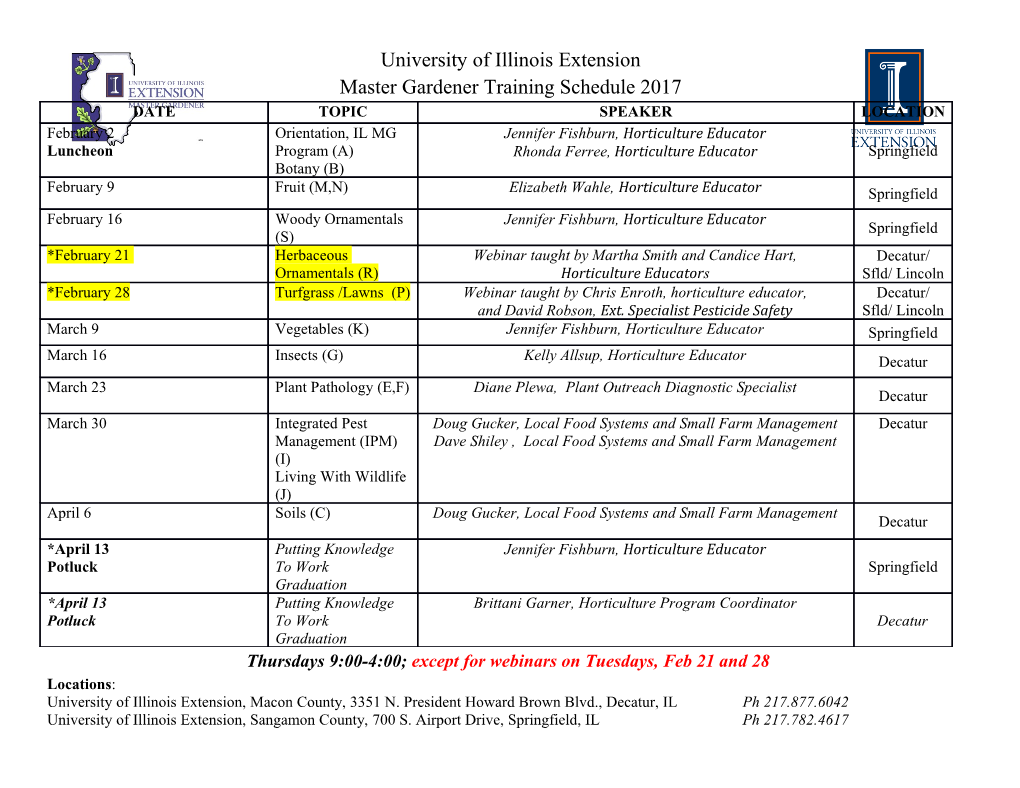
Creating Fair Models of Atherosclerotic Cardiovascular Disease Risk Stephen Pfohl1, Ben Marafino1,∗, Adrien Coulet1,2,∗ Fatima Rodriguez3, Latha Palaniappan4, Nigam H. Shah1 1 Stanford Center for Biomedical Informatics Research, Stanford University, Stanford, California 2 Universite´ de Lorraine, CNRS, Inria, Loria, Nancy, France 3 Cardiovascular Medicine and Cardiovascular Institute, Stanford University, Stanford, California 4 Primary Care and Population Health, Stanford University, Stanford, California Abstract cular events or side effects from unnecessary therapy, re- spectively. Guidelines for the management of atherosclerotic cardiovas- The inability of the PCEs to generalize to diverse cohorts cular disease (ASCVD) recommend the use of risk strat- likely owes to both under-representation of minority popu- ification models to identify patients most likely to benefit lations in the cohorts used to develop the PCEs and shifts in from cholesterol-lowering and other therapies. These models have differential performance across race and gender groups medical practice and lifestyle patterns in the decades since with inconsistent behavior across studies, potentially result- data collection for those cohorts. In attempting to correct for ing in an inequitable distribution of beneficial therapy. In this these patterns, one recent study (Yadlowsky et al. 2018) up- work, we leverage adversarial learning and a large observa- dated the PCEs using data from contemporary cohorts and tional cohort extracted from electronic health records (EHRs) demonstrated that doing so reduced the number of minor- to develop a ”fair” ASCVD risk prediction model with re- ity patients incorrectly misclassified as being high or low duced variability in error rates across groups. We empiri- risk. Similar results were observed in the same study with cally demonstrate that our approach is capable of aligning the an approach using an elastic net classifier, rather than a distribution of risk predictions conditioned on the outcome proportional hazards model. However, neither approach is across several groups simultaneously for models built from able to explicitly guarantee an equitable distribution of mis- high-dimensional EHR data. We also discuss the relevance of these results in the context of the empirical trade-off between estimation across relevant subgroups, particularly for race- fairness and model performance. and gender-based subgroups. To account for under-represented minorities and to take advantage of the wider variety of variables made available in Introduction electronic health records (EHRs), we derive a large and di- verse modern cohort from EHRs to learn a prediction model Atherosclerotic cardiovascular disease (ASCVD), which in- for ASCVD risk. Furthermore, we investigate the extent to cludes heart attack, stroke, and fatal coronary heart dis- which we can encode algorithmic notions of fairness, specif- ease, is a major cause of mortality and morbidity world- ically equality of odds, (Hardt, Price, and Srebro 2016) into wide, as well as in the U.S., where it contributes to 1 in the model to encourage an equitable distribution of perfor- 3 of all deaths–many of which are preventable (Benjamin mance across populations. To the best of our knowledge, our et al. 2018). In deciding whether to prescribe cholesterol- effort is the first to explore the extent to which this formal lowering therapies to prevent ASCVD, physicians are often fairness metric is achievable for risk prediction models built guided by risk estimates yielded by the Pooled Cohort Equa- using high-dimensional data from the EHR. We show that tions (PCEs). PCEs provide a proportional hazards model while it is feasible to develop models that achieve equality (Goff et al. 2013) that leverages nine clinical measurements of odds, we emphasize that this process involves trade-offs to predict the 10-year risk of a first ASCVD event. How- that must be assessed in a broader social and medical context ever this model has been found to overestimate risk for fe- (Verghese, Shah, and Harrington 2018). male patients (Mora et al. 2018), Chinese patients (De Fil- ippis et al. 2017) or globally (Yadlowsky et al. 2018), as well as also underestimate risk for other groups such as Ko- Background and Related Work rean women (Jung et al. 2015). Such mis-estimation results ASCVD Risk Prediction and EHRs in an inequitable distribution of the benefits and harms of ASCVD risk scoring, because incorrect risk estimates can The PCEs are based on age, gender, cholesterol levels, blood expose patients to substantial harm through both under- or pressure, and smoking and diabetes status and were devel- over-treatment; potentially leading to preventable cardiovas- oped by pooling data from five large U.S. cohorts (Goff et al. 2013) composed of white and black patients, with white ∗These authors contributed equally patients constituting a majority. Recently, attempts (Yad- Copyright c 2019, Association for the Advancement of Artificial lowsky et al. 2018) were made to update the PCEs to im- Intelligence (www.aaai.org). All rights reserved. prove model performance for race- and gender-based sub- groups using elastic net regression and data from modern sensitive attribute and the outcome exists (Hardt, Price, and prospective cohorts. However, this effort focused on de- Srebro 2016). mographic groups and variables already used to develop Furthermore, this definition can be extended to the case of the PCEs and did not consider other populations or clin- a continuous risk score by requiring that ical measurements. The increasing adoption of EHRs of- fers opportunities to deploy and refine ASCVD risk mod- p(f(X)jZ = Zi;Y = Yk) = p(f(X)jZ = Zj;Y = Yk) els. Efforts have recently been undertaken to apply and re- 8 Zi;Zj 2 Z; Yk 2 Y: (3) fine existing models, including the PCEs and the Framing- ham score, to large EHR-derived cohorts and characterize In this case, the distribution of the predicted probability of their performance in certain subgroups (Pike et al. 2016; the outcome conditioned on whether the event occurred or Rana et al. 2016). Beyond ASCVD risk prediction, there ex- not should be matched across groups of a sensitive variable. ist many recent works that develop prediction models with Formulation 3 is stronger than 2 since it implies that equality EHRs, which are reviewed in (Goldstein et al. 2017). of odds is achieved for all possible thresholds, thus requiring that the same ROC curve be attained for all groups. This is Fair Risk Prediction desirable since it provides the end-user the ability to freely We consider the case where supervised learning is used to adjust the decision threshold of the model without violating estimate a function f(X) that approximates the conditional equality of odds. N Finally, we also note that satisfying equality of odds for distribution p(Y jX), given N samples fxi; yi; zigi=1 drawn from the distribution p(X; Y; Z). We take X 2 X = Rm to a continuous risk score may be reduced to the problem of correspond to a vector representation of the medical history minimizing a divergence over each pair (Zi;Zj) of distribu- extracted from the EHR prior to a patient-specific index time tions referenced in equation (3). Adversarial learning proce- ti; Y 2 Y = f0; 1g to be a binary label, which for patient i, dures (Goodfellow et al. 2014) are well-suited to this prob- indicates the presence of the outcome observed in the EHR lem in that they provide a flexible framework for minimizing in the time frame [ti; ti + wi], where wi is a parameter spec- the divergence over distributions parameterized by neural ifying the amount of time following the index time used to networks. As such, several related works (Zhang, Lemoine, derive the outcome; and Z 2 Z = f0; : : : ; k − 1g indi- and Mitchell 2018; Beutel et al. 2017; Edwards and Storkey cates a sensitive attribute, such as race, gender, or age, with 2015; Madras et al. 2018) have demonstrated the benefit of k groups. The output of the learned function f(X) 2 [0; 1] augmenting a classifier with an adversarial discriminator in is then thresholded with respect to a value T to yield a pre- order to align the distribution of predictions for satisfying diction Y^ 2 f0; 1g. fairness constraints. One standard metric for assessing the fairness of a classi- fier with respect to a sensitive attribute Z is demographic Approaches for Achieving Fairness parity (Dwork et al. 2012), which evaluates the indepen- Despite considerable interest in the ethical implications of dence between Z and the prediction Y^ . Formally, the de- implementing machine learning in healthcare (Char, Shah, mographic parity criterion may be expressed as and Magnus 2018; Cohen et al. 2014), relatively little work exists characterizing the extent to which risk prediction ^ ^ p(Y jZ = Zi) = p(Y jZ = Zj) 8 Zi;Zj 2 Z: (1) models developed with EHR data satisfy formal fairness However, optimizing for demographic parity is of limited constraints. use for clinical risk prediction, because doing so may pre- Adversarial approaches for satisfying fairness constraints clude the model from considering relevant clinical features (in the form of demographic parity) have been explored in associated with the sensitive attribute and the outcome, thus several recent works in non-healthcare domains. One ap- decreasing the performance of the model for all groups proach, (Edwards and Storkey 2015), in the context of image (Kleinberg, Mullainathan, and Raghavan 2016). anonymization, demonstrated that representations satisfying Another related metric is equality of odds (Hardt, Price, demographic parity could be learned by augmenting a pre- dictive model with both an autoencoder and an adversarial and Srebro 2016), which stipulates that the prediction Y^ be component. The adversarial approach to fairness was further conditionally independent of Z, given the true label Y . For- investigated by (Beutel et al. 2017) with a gradient reversal mally, satisfying equality of odds implies that objective for data that is imbalanced in the distribution of both the outcome and in the sensitive attribute.
Details
-
File Typepdf
-
Upload Time-
-
Content LanguagesEnglish
-
Upload UserAnonymous/Not logged-in
-
File Pages11 Page
-
File Size-