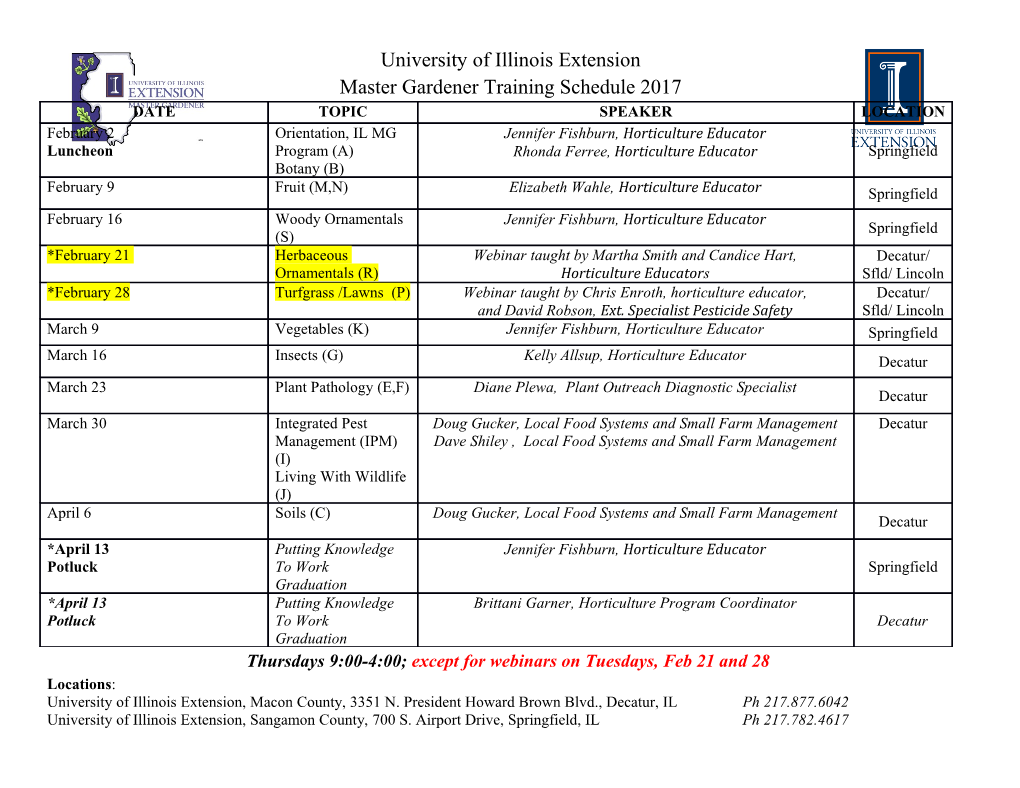
Asymmetric Tsallis distributions for modelling financial market dynamics Sandhya Devi† 509 6th Ave S, Edmonds, WA 98020, United States of America Email: [email protected] Abstract: Financial markets are highly non-linear and non-equilibrium systems. Earlier works have suggested that the behavior of market returns can be well described within the framework of non-extensive Tsallis statistics or superstatistics. For small time scales (delays), a good fit to the distributions of stock returns is obtained with q-Gaussian distributions, which can be derived either from Tsallis statistics or superstatistics. These distributions are symmetric. However, as the time lag increases, the distributions become increasingly non-symmetric. In this work, we address this problem by considering the data distribution as a linear combination of two independent normalized distributions – one for negative returns and one for positive returns. Each of these two independent distributions are half q-Gaussians with different non-extensivity parameter q and temperature parameter beta. Using this model, we investigate the behavior of stock market returns over time scales from 1 to 80 days. The data covers both the .com bubble and the 2008 crash periods. These investigations show that for all the time lags, the fits to the data distributions are better using asymmetric distributions than symmetric q-Gaussian distributions. The behaviors of the q parameter are quite different for positive and negative returns. For positive returns, q approaches a constant value of 1 after a certain lag, indicating the distributions have reached equilibrium. On the other hand, for negative returns, the q values do not reach a stationary value over the time scales studied. In the present model, the markets show a transition from normal to superdiffusive behavior (a possible phase transition) during the 2008 crash period. Such behavior is not observed with a symmetric q-Gaussian distribution model with q independent of time lag. Keywords: non-extensive systems, Tsallis statistics, superstatistics, entropy, nonlinear dynamics, asymmetry, superdiffusion, models of financial markets, econophysics 1. Introduction Many well-known financial models [1] are based on the efficient market hypothesis [2] according to which: a) investors have all the information available to them and they independently make rational decisions using this information, b) the market reacts to all the information available reaching equilibrium quickly, and c) in this equilibrium state the market essentially follows a random walk [3]. In such a system, extreme changes are very rare. In reality however, the market is a complex system that is the result of decisions by interacting agents (e.g., herding behavior), traders who speculate and/or act impulsively on little news, etc. Such collective/chaotic behavior † Shell International Exploration and Production Co. (Retired) Asymmetric Tsallis distributions can lead to wild swings in the system, driving it away from equilibrium into the realm of nonlinearity, resulting in a variety of interesting phenomena such as phase transitions, critical phenomena such as bubbles, crashes [4], superdiffusion [5] and so on. The distribution of a system following a random walk can be obtained by maximizing Shannon entropy [6][7] with constraints on the moments. In particular, this maximization with constraints on the normalization and the second moment yields a Gaussian distribution. Therefore, if the stock market follows a pattern of random walk, the corresponding returns should show a Gaussian distribution. However, it is well known [8] that stock market returns, in general, have a more complicated distribution. This is illustrated in figure 1 which compares the distributions of 1 day and 20 day log returns of S&P 500 and Nasdaq stock markets (1994-2014) with the corresponding Gaussian distributions. The data distributions show sharp peaks in the center and fat tails over many scales, neither of which is captured by the Gaussian distribution. Several studies [9][10] indicate that these issues can be addressed using statistical methods based on Tsallis entropy [11] (also known as q-entropy), which is a generalization of Shannon entropy to non-extensive systems. These formalisms were originally proposed to study classical and quantum chaos, physical systems far from equilibrium such as turbulent systems, and long range interacting Hamiltonian systems. However, in the last several years, there has been considerable interest in applying these methods to analyze financial market dynamics as well. Such applications fall into the category of econophysics [5]. As in the case of Shannon entropy, the equilibrium distribution of a non-extensive system can be obtained by maximizing Tsallis entropy with constraints on the moments of the distribution [11]. Constraints on the normalization and the second moment yield a symmetric q-Gaussian distribution. As q → 1, the system tends to an extensive system and the q-Gaussian distribution approaches a Gaussian distribution. Let us now look at the non-equilibrium situation. In the random walk model, the dynamics of the probabilities are described by a Fokker Plank (FP) equation with linear drift and diffusion terms. The solution to this FP equation is a Gaussian distribution. In this case, the autocorrelation of the standard deviation 휎 falls as √휏 where 휏 is the time delay. A generalization of Fokker-Plank equation to non-extensive systems, using statistical methods based on Tsallis entropy, has been given by [12][13]. This results in a Fokker-Plank equation with a linear drift term and a non-linear diffusion term. The solution to this equation, under some assumptions, is a Tsallis q-Gaussian distribution, with the parameter q independent of time. For q < 5/3, the generalized inverse mean square deviation of this distribution follows a power law, with the magnitude of the exponent > 1. This points to a superdiffusive process. This model has been applied to study both high frequency [14][15] and long-term low frequency stock market returns [16]. However, in a previous publication [17] we have shown that for low frequency returns (time delay >= 1 day) with q independent of time delay, the inverse mean square deviation shows a power law behavior with the exponent very close to 1 pointing to a normal diffusive behavior. Hence, for returns longer than one day, the predicted superdiffusive behavior from the non-linear FP equations discussed above is not supported by the empirical results. 2 Asymmetric Tsallis distributions The non-extensive statistics is relevant for two classes of systems: a) Systems which have interacting sub-systems: Tsallis formulation of non-extensivity based on entropy principle is used for this class. In this case, the entropies of the sub-systems are not additive. b) Superstatistics: Beck [18] showed that non-extensivity can also arise in systems following ordinary statistics if the temperature or energy dissipation rate is locally fluctuating. In this case, the distribution can be considered as the integral of the probability density 푓(훽) of the inverse temperature β with the Boltzmann-Gibbs (BG) distribution function 푒−훽퐸. This is a superposition of two statistics – hence the name ‘superstatistics’. When 훽 has a 휒2 distribution (which is widely applicable to many common systems [19]), the superstatistics yields a q-exponential or q-Gaussian distribution, depending on the definition of E [20][21]. One thing to note in the case of superstatistics is that even though the system follows a BG distribution, because of the temperature variation, the entropy is quasi-additive [18]. This means, if we consider two independent systems 퐴 and 퐵 having some value 푞, the entropy of the composite system 퐴 + 퐵 is the sum of the entropies of 퐴 and 퐵 but with a different 푞. For dynamical systems, if 퐴 and 퐵 belong to smaller time scales, the system with a larger time scale will have a different 푞. Hence in superstatistics, the non-extensivity parameter changes with time scale. We will discuss the dynamics of 푞 in more detail in section 2 since this is an important aspect of the present analysis. Neither of the two non-extensivity models discussed above supports asymmetry. A perturbative approach to treating asymmetry has been discussed in [22]. However, a look at figure 2, which displays the variation of skew with time lag for S&P 500 and Nasdaq returns for the .com bubble and the 2008 crash periods, clearly shows that the skew rises very rapidly with time lag. This is particularly pronounced during the crash period. Hence, except for very small time scales, the perturbative approach for treating asymmetry will not be adequate. Budini [23] has derived a family of extended 푞-Gaussian distributions starting from two gamma distributions that have different shape parameters but the same temperature parameters. The resulting distribution is asymmetric only in the shape parameter and not in the spatial scale parameter. In this paper we address the asymmetry in the distribution of financial market returns by requiring that both 푞 and the ‘temperature’ parameter 훽 depend on the sign of the market returns. Since asymmetry increases with time delay, both these parameters change with time scale. The rest of paper is organized as follows. A brief review of derivations of q-Gaussian distributions from Tsallis entropic formulation and superstatistics will be given in section 2. Our formulation of asymmetric distributions will be discussed in section 3. Results of application of this formulation to financial market dynamics will be given in section 4 followed by summary and conclusions in section 5. 3 Asymmetric Tsallis distributions 2. q-Gaussian distributions 2.1 Tsallis statistics A detailed review of the derivation of q-Gaussian distribution from Tsallis entropy and the estimation of the parameters of the model is given in an earlier publication [17]. As already discussed earlier, in Tsallis statistics the non-extensivity of a system arises due to the long range interactions of the sub-systems.
Details
-
File Typepdf
-
Upload Time-
-
Content LanguagesEnglish
-
Upload UserAnonymous/Not logged-in
-
File Pages28 Page
-
File Size-