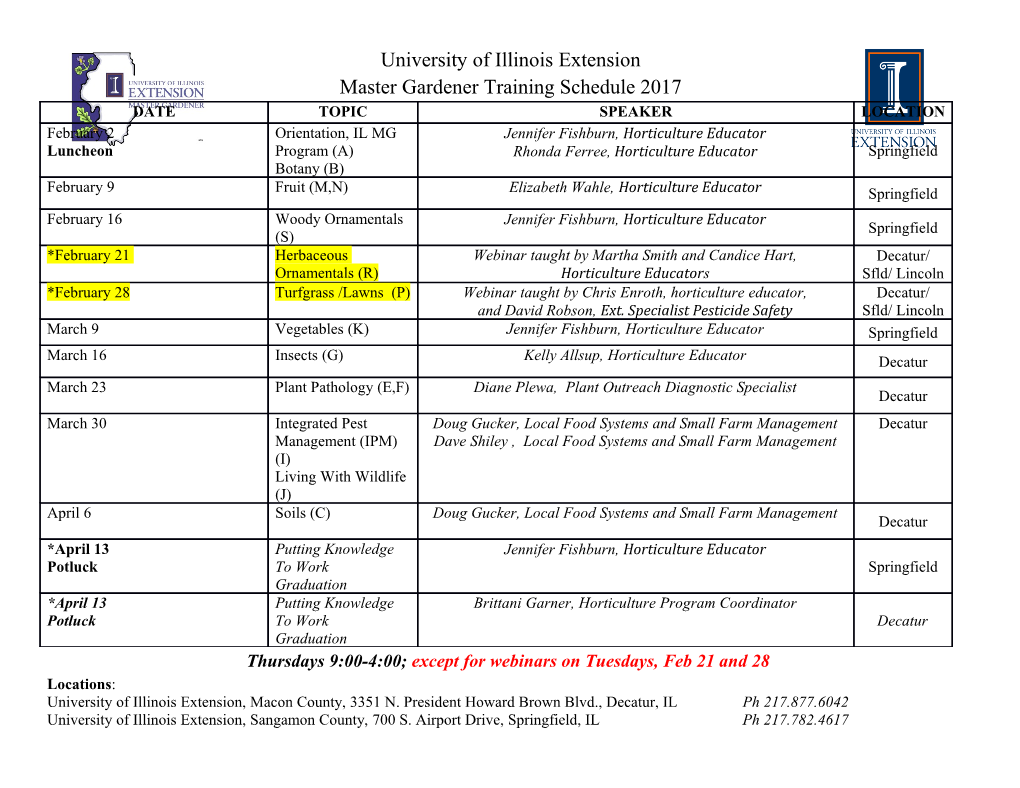
STATISTICAL INFERENCES HYPOTHESIS TESTS Maªgorzata Murat BASIC IDEAS Suppose we have collected a representative sample that gives some information concerning a mean or other statistical quantity. There are two main questions for which statistical inference may provide answers. 1. Are the sample quantity and a corresponding population quantity close enough together so that it is reasonable to say that the sample might have come from the population? Or are they far enough apart so that they likely represent dierent populations? 2. For what interval of values can we have a specic level of condence that the interval contains the true value of the parameter of interest? STATISTICAL INFERENCES FOR THE MEAN We can divide statistical inferences for the mean into two main categories. In one category, we already know the variance or standard deviation of the population, usually from previous measurements, and the normal distribution can be used for calculations. In the other category, we nd an estimate of the variance or standard deviation of the population from the sample itself. The normal distribution is assumed to apply to the underlying population, but another distribution related to it will usually be required for calculations. TEST OF HYPOTHESIS We are testing the hypothesis that a sample is similar enough to a particular population so that it might have come from that population. Hence we make the null hypothesis that the sample came from a population having the stated value of the population characteristic (the mean or the variation). Then we do calculations to see how reasonable such a hypothesis is. We have to keep in mind the alternative if the null hypothesis is not true, as the alternative will aect the calculations. LEVEL OF SIGNIFICANCE The observed level of signicance or p-value is the probability of obtaining a result as far away from the expected value as the observation is, or farther, purely by chance, when the null hypothesis is true. Notice that a smaller observed level of signicance indicates that the null hypothesis is less likely. If this observed level of signicance is small enough, we conclude that the null hypothesis is not plausible. LEVEL OF SIGNIFICANCE In many instances we choose a critical level of signicance before observations are made. The most common choices for the critical level of signicance are 10%, 5%, and 1%. If the observed level of signicance is smaller than a particular critical level of signicance, we say that the result is statistically signicant at that level of signicance. If the observed level of signicance is not smaller than the critical level of signicance, we say that the result is not statistically signicant at that level of signicance. p-VALUE APPROACH p-value: the smallest signicance level at which the null hypothesis can be rejected. How to compute p-value? First, compute the test statistic. Then the p-value is computed as the probability of a result as extreme and more extreme as the observed tests statistics in the direction of the alternative hypothesis. THE PROCEDURE FOR HYPOTHESIS TESTS 1. State H0 in terms of a population parameter, such as µ or σ2. 2. State Ha in terms of the same population parameter. 3. State the test statistic, substituting quantities given by the null hypothesis but not the observed values. State what statistical distribution is being used. 4. Show calculations assuming that the null hypothesis is true. 5. Report the observed level of signicance p-value. 6. State a conclusion, that might be either to accept the null hypothesis, or else to reject the null hypothesis in favour of the alternative hypothesis. ILLUSTRATION Say the percentage metal in the tailings stream from a otation mill in the metallurgical industry has been found to follow a normal distribution. When the mill is operating normally, the mean percentage metal in the stream is 0.370 and the standard deviation is 0.015. These are assumed to be population values, µ and σ. Now a plant operator takes a single specimen as a sample and nds a percentage metal of 0.410. Does this indicate that something in the process has changed, or is it still reasonable to say that the mill is operating normally? Tabel-Cumulative normal probability TYPES OF POSSIBLE ERROR We may decide to take some action on the basis of the test of signicance, such as adjusting the process if a result is statistically signicant. But we can never be completely certain we are taking the right action. There are two types of possible error which we must consider. TYPES OF POSSIBLE ERROR H0 is true H0 is false accept H0 correct decision type II error reject H0 type I error correct decision SPECIFICATIONS OF A DECISION SYSTEM The type I error specication is the probability of making errors when the null hypothesis is true. This specication is commonly represented with the symbol α. For example if we say that a test has α ¬ 0.05 we guarantee that if the null hypothesis is true the test will not make more than 1 mistakes. 20 The type II error specication is the probability of making errors when the null hypothesis is false. This specication is commonly represented with the symbol β. For example if we say that for a test β is unknown we say that we cannot guarantee how it will behave when the null hypothesis is actually false. The power specication is the probability of correctly rejecting the null hypothesis when it is false. Thus the power specication is 1 − β. REJECTION REGION APPROACH Critical values: The values of the test statistic that separate the rejection and non-rejection regions. They are the boundary values obtained corresponding to the preset α level. Rejection region: The set of values for the test statistic that leads to rejection of H0. Non-rejection region: the set of values not in the rejection region that leads to non-rejection of H0. THE PROCEDURE FOR HYPOTHESIS TESTS 1. State H0 in terms of a population parameter, such as µ or σ2. 2. State Ha in terms of the same population parameter. 3. State the test statistic, substituting quantities given by the null hypothesis but not the observed values. State what statistical distribution is being used. 4. Show calculations assuming that the null hypothesis is true. 5. Report critical values and rejection region. 6. State a conclusion, that might be either to accept the null hypothesis, or else to reject the null hypothesis in favour of the alternative hypothesis. Comparing the P-Value Approach to the Rejection Region Approach Both approaches will ensure the same conclusion and either one will work. However, using the p-value approach has the following advantages: Using the rejection region approach, you need to check the table for the critical value every time people give you a dierent value. In addition to just using it to reject or not reject Ho by comparing p-value to value, p-value also gives us some idea of the strength of the evidence against H0. INFERENCES FOR THE MEAN WHEN VARIANCE IS ESTIMATED In most cases the variance or standard deviation must be estimated from a sample. If variance is estimated from a sample of moderate size, that estimate is also subject to random error related to the size of the sample. The larger the sample, the more reliable the estimate of variance becomes. The quantitative relation is expressed in terms of the degrees of freedom of the sample. The degrees of freedom refer to the number of pieces of independent information used to estimate the variance. The sample mean, x , was calculated from n independent quantities, xi. But the deviations from the mean, (xi − x) are not all independent. The number of independent pieces of information on which the variance or standard deviation is based is (n − 1). INFERENCES FOR THE MEAN WHEN VARIANCE IS ESTIMATED A sample of size n gives an estimate of variance n 2 1 X 2 s = (xi − x) . n − 1 i=1 The independent variable of the normal distribution x−µ σ applied to sample means is z = , where σx = √ . σx n If we don't know σ, we estimate it from a sample by the estimated standard deviation, s. Then instead of z x − µ we have the variable t = √ . s/ n If the variance is estimated from a sample, statistical inferences should be made using the t-distribution rather than the normal distribution. If the number of degrees of freedom is large enough, the normal distribution can be used as an approximation to the t-distribution. EXAMPLE The electrical resistances of components are measured as they are produced. A sample of six items gives a sample mean of 2.62 ohms and a sample standard deviation of 0.121 ohms. Is there evidence at the 2% that a mean of the electrical resistances of components is 2.80 ohms? Table- t-distribution.
Details
-
File Typepdf
-
Upload Time-
-
Content LanguagesEnglish
-
Upload UserAnonymous/Not logged-in
-
File Pages10 Page
-
File Size-