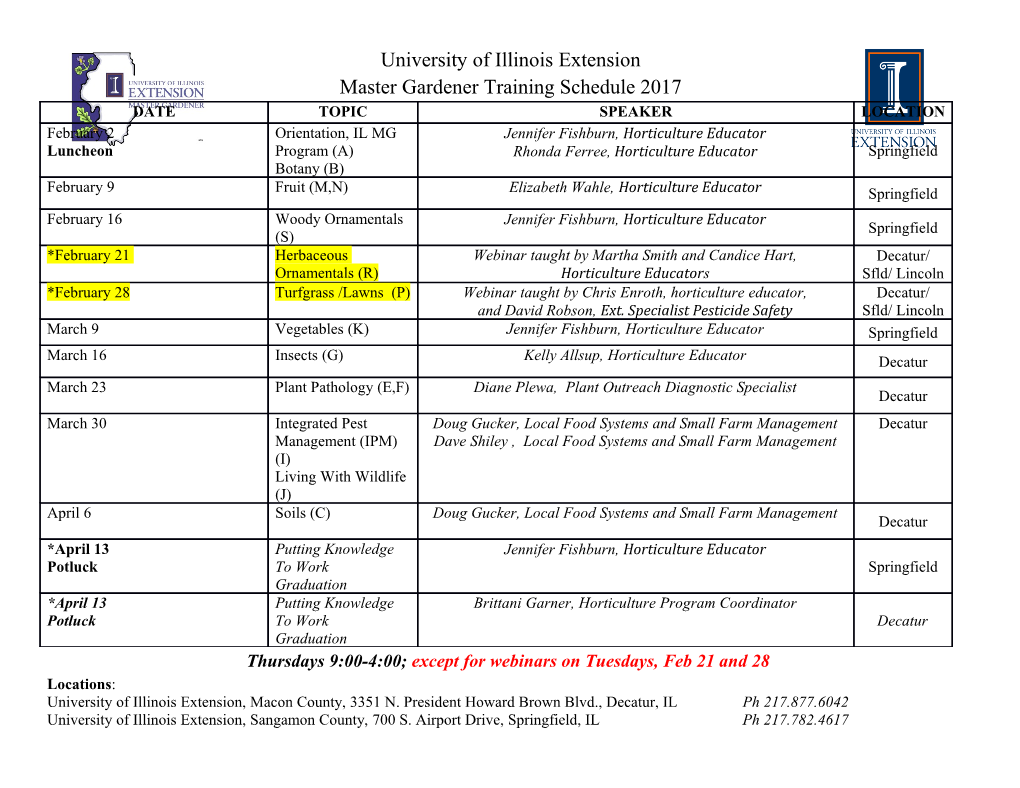
Human-AI Collaboration for Natural Language Generation with Interpretable Neural Networks a dissertation presented by Sebastian Gehrmann to The John A. Paulson School of Engineering and Applied Sciences in partial fulfillment of the requirements for the degree of Doctor of Philosophy in the subject of Computer Science Harvard University Cambridge, Massachusetts May 2020 ©2019 – Sebastian Gehrmann Creative Commons Attribution License 4.0. You are free to share and adapt these materials for any purpose if you give appropriate credit and indicate changes. Thesis advisors: Barbara J. Grosz, Alexander M. Rush Sebastian Gehrmann Human-AI Collaboration for Natural Language Generation with Interpretable Neural Networks Abstract Using computers to generate natural language from information (NLG) requires approaches that plan the content and structure of the text and actualize it in fuent and error-free language. The typical approaches to NLG are data-driven, which means that they aim to solve the problem by learning from annotated data. Deep learning, a class of machine learning models based on neural networks, has become the standard data-driven NLG approach. While deep learning approaches lead to increased performance, they replicate undesired biases from the training data and make inexplicable mistakes. As a result, the outputs of deep learning NLG models cannot be trusted. Wethus need to develop ways in which humans can provide oversight over model outputs and retain their agency over an otherwise automated writing task. This dissertation argues that to retain agency over deep learning NLG models, we need to design them as team members instead of autonomous agents. We can achieve these team member models by considering the interaction design as an integral part of the machine learning model development. We identify two necessary conditions of team member-models – interpretability and controllability. The models need to follow a reasoning process such that human team members can understand and comprehend it. Then, if humans do not agree with the model, they should be able to change the reasoning process, and the model should adjust its output accordingly. In the frst part of the dissertation, we present three case studies that demonstrate how interactive interfaces can positively afect how humans understand model predictions. In the second part, we introduce a neural network-based approach to document summarization that directly models the se- lection of relevant content. We show that, through this selection, a human user can control what part of a document the algorithm summarizes. In the fnal part of this dissertation, we show that this de- sign approach, coupled with an interface that exposes these interactions, can lead to a forth and back between human and autonomous agents where the two actors collaboratively generate text. This dis- sertation thus demonstrates how to develop models with these properties and how to design neural networks as team members instead of autonomous agents. iii Contents 1 Introduction 1 1.1 Thesis Overview .................................. 7 1.2 Contributions ................................... 11 2 Natural Language Generation 13 2.1 Notation ...................................... 15 2.2 Natural Language Generation Tasks ........................ 15 2.3 Deep Learning for Natural Language Processing . 20 2.4 Approximate Search and Text Generation ..................... 34 3 Understanding Users and their Interpretability Needs 36 3.1 User Types: Architects, Trainers, and End Users . 42 3.2 Design Space for Integrating Machine Learning into Interfaces . 44 4 Evaluating Explanations in Automated Patient Phenotyping 51 4.1 Phenotyping in large EHR datasets ......................... 54 4.2 Data ........................................ 56 4.3 Methods ...................................... 57 4.4 Deriving Salient Features .............................. 60 4.5 Evaluation ..................................... 62 4.6 Results ....................................... 64 4.7 Discussion and Conclusion ............................. 68 5 Interactively Understanding Recurrent Neural Networks 70 5.1 Visualization for understanding neural networks . 72 5.2 User Analysis and Goals .............................. 73 5.3 Design of LSTMVis ................................ 75 5.4 Use Cases ...................................... 81 5.5 Long-Term Case Study ............................... 86 5.6 Conclusion ..................................... 88 iv 6 Debugging Predictions of Sequence-to-Sequence Models 90 6.1 Motivating Case Study: Debugging Translation . 94 6.2 Goals and Tasks ................................... 98 6.3 Design of Seq2Seq-Vis .............................. 101 6.4 Use Cases ...................................... 106 6.5 Conclusions .................................... 111 7 Bottom-Up Summarization: Extending Models with Controllable Variables 113 7.1 Related Work .................................... 116 7.2 Background: Neural Summarization . 118 7.3 Bottom-Up Attention ............................... 119 7.4 Inference ...................................... 123 7.5 Data and Experiments ............................... 124 7.6 Results ....................................... 125 7.7 Analysis and Discussion .............................. 128 7.8 Conclusion ..................................... 132 8 Collaborative Semantic Inference 134 8.1 Interactive Collaboration .............................. 136 8.2 Rearchitecting models to enable collaborative semantic inference . 139 8.3 Use Case: A Collaborative Summarization Model . 143 8.4 Details on the Summarization Model Hooks . 145 8.5 Towards a Co-Design Process for CSI Systems . 154 8.6 Conclusions .................................... 156 9 Discussion and Conclusion 158 9.1 The evaluation of text-generating models . 159 9.2 The ethical permissibility of text-generating models . 162 9.3 Collaboration in other domains . 166 9.4 Conclusion ..................................... 167 References 169 v Acknowledgments In graduate school, you are thrown into the deep water and your advisors teach you to swim as you go along. The frst time I wrote a paper, my advisors told me that they would like me to write it again. For my latest paper, I received small editorial comments. I am, therefore, confdent that I have learned to swim. For that, I would like to thank my advisors Barbara Grosz and Sasha Rush, who have given me the opportunity to become a researcher and have supported me in so many ways. Barbara was frst person who exposed me to AI, and I am forever grateful for taking me taking me on as a student and believing in me to grow as a researcher. I know most things I know about NLP thanks for Sasha’s guidance who imprinted on me his striving for fawlessly presented arguments and well-crafted code. Their support has been unwavering and their advice and subtle nudges continue to steer me in the right direction. T list of people who helped make this dissertation possible continues with Stuart Shieber, who never fails to amaze with his thoughtful suggestions and impromptu lectures on his whiteboard. I am grateful to Krzysztof Gajos for accepting me as a perpetual guest into the HCI group. Our conversa- tions indirectly infuenced much of this dissertation. I will miss our 11am tea’s and participating in paper trashing sessions. Yet another person who I am happy to count as an advisor and friend is Ofra Amir. She guided my frst steps in the research world, frst as advisor and later as an ofce mate. Her advice is always useful, be it about AI or hiking. Despite my great advisors, my research would be nothing without the support from my collabo- rators. This list cannot start without thanking Hendrik Strobelt, who over the last years has been in- strumental in most work presented in this dissertation. Despite our diferent backgrounds, we some- how learned each others language and managed to successfully work on projects. Discussions are always better over cake. Continuing the list of amazing collaborators, I would further like to thank David Grant, Eric Carlson, Falcon Dai, Franck Dernoncourt, Hanspeter Pfster, Henry Elder, Jody Lin, Joy Wu, Lauren Urke, Lee Sanders, Leo Celi, Michael Behrisch, Mirac Suzgun, Ned Moseley, Patrick Tyler, Payel Das, Robert Krüger, Steven Layne, Tom Sercu, Yeran Li, Yonatan belinkov, Yun- tian Deng, and Zach Ziegler for all their contributions toward making this dissertation possible. I am also extremely thankful for all the great experiences I had during my internships. I got to work with a group of wonderful people at the Bruno Kessler Foundation in Italy, most of all Alessandro Cappelletti, Eleonora Mencarini, Gianluca Shiavo, Massimo Zancanaro, and Oliviero Stock. Simi- larly, I happily look at back at my times at Adobe where I got to work with Carl Dockhorn, Franck Dernoncourt, and Lynn Gong. I also thank my Adobe ofce mates and friends Anthony, Chan- vi Young, Darian, Dung, Gabbi, Jessica, Nham, Sean, and Steve for the great time. Eating ribs at the beach, taking photos of the redwood trees while hiking, and building LaCroix statues are memories I will never forget. I could not have asked for a better group of people to be stuck with me in a tiny conference room. Next, I would like to thank all of the permanent and temporary members of the Harvard NLP group, Alex, Allen, Angela, Falcon, Justin, Kelly, Luke, Mirac, Rachit, Sam, Yonatan, Yoon, Yuntian, and Zach. Our reading groups were more educational than any class, and the fun I had at conferences
Details
-
File Typepdf
-
Upload Time-
-
Content LanguagesEnglish
-
Upload UserAnonymous/Not logged-in
-
File Pages205 Page
-
File Size-